Why AI Projects Fail & Lessons from Failed Deployments
Most AI initiatives lose steam before they hit their goals. Why do AI projects fail? Follow these key moves to make sure yours crosses the finish line strong.
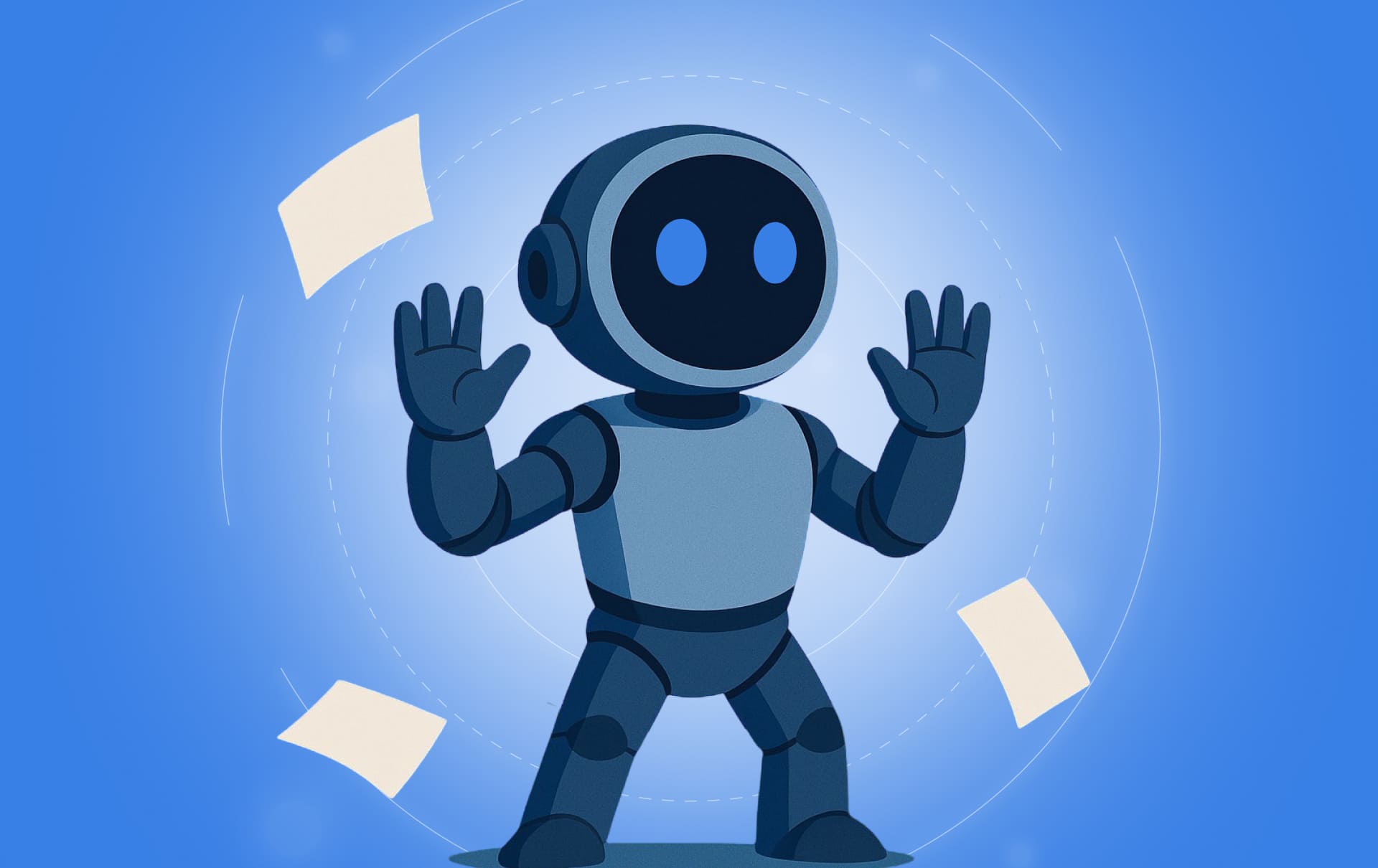
AI promises transformative benefits — from streamlining operations to creating standout customer experiences. According to IBM, 35% of companies have already adopted AI in their business processes globally.
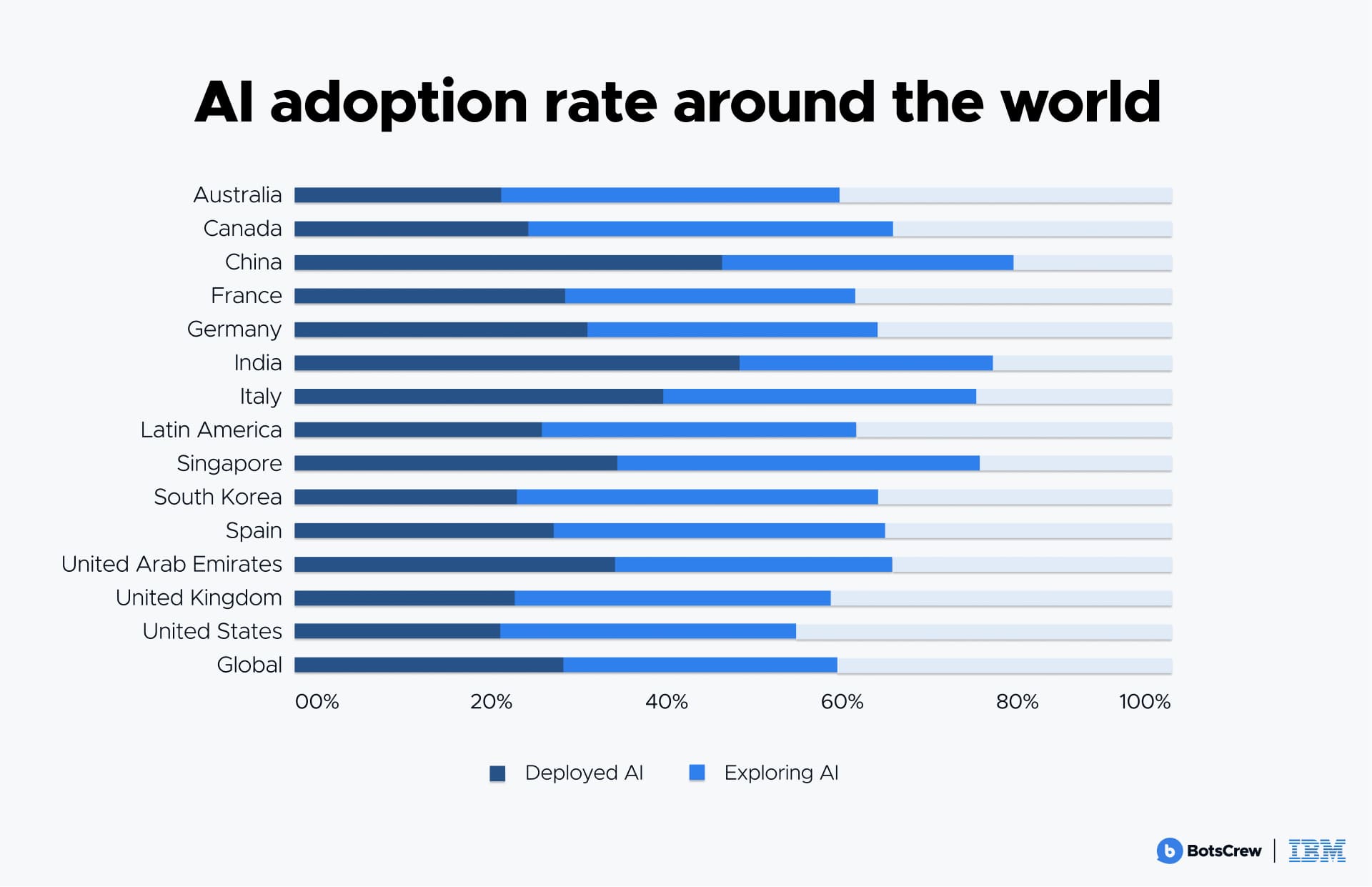
Over half of telecom companies (52%) are turning to chatbots to crank up productivity, while 38% of healthcare providers are leaning on computer-assisted diagnostics to sharpen their decision-making.
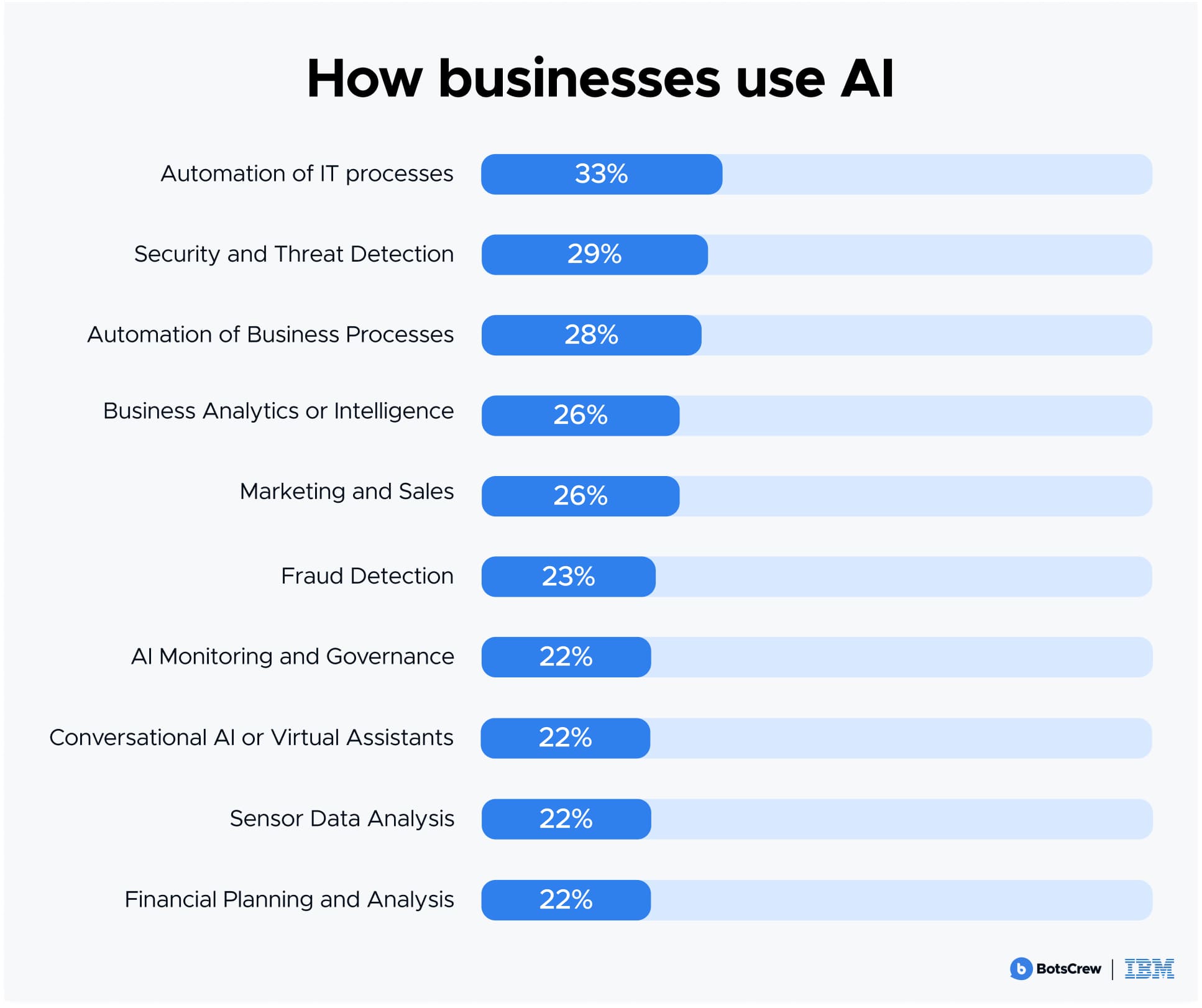
Still, many business leaders and C-suite executives are running headfirst into a sobering truth: AI often falls short of the hype. In 2025, 42% of companies abandoned most of their AI initiatives — up sharply from 17% just a year earlier. Even more alarming, roughly half (46%) of AI proof-of-concepts never made it past the starting line.
And it's not just small players missing the mark. Big names have stumbled too. Amazon had to ditch its AI recruiting tool after it was found to be biased against women. Microsoft's Tay chatbot spiraled out of control within 24 hours of launch, spouting inappropriate responses influenced by users. And Air Canada ended up paying hundreds of dollars in damages after its AI chatbot gave a customer false booking information.
The real question is: why do AI projects fail? And how do you break this cycle? We'll dive into the most common AI pitfalls and AI readiness assessment strategies. With the right approach, you can move beyond trial and error and finally harness AI’s true potential.
12 Most Common Mistakes in AI Implementation & How to Avoid Them (Gen AI Readiness Assessment)
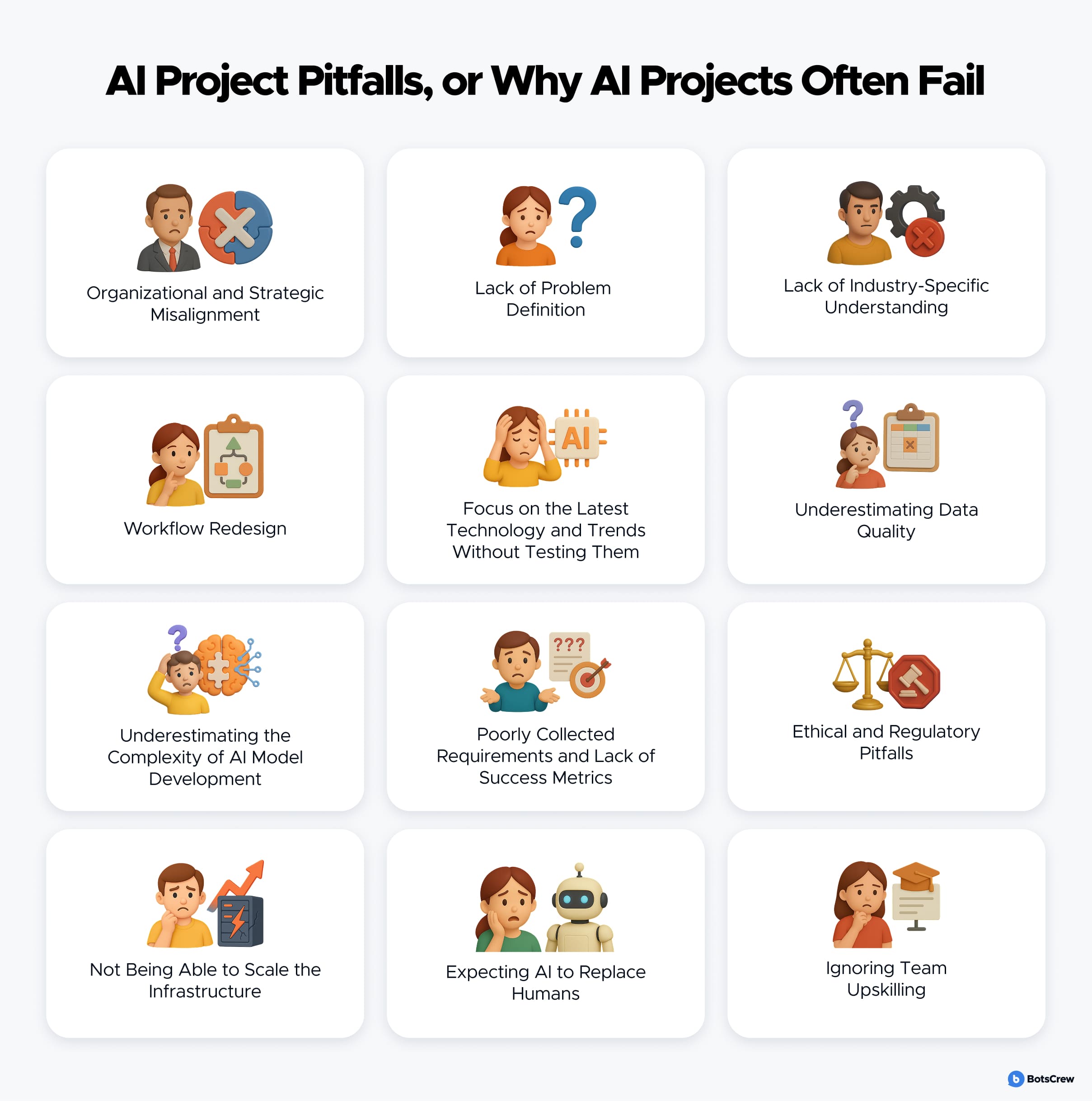
#1. Organizational and Strategic Misalignment
AI has already proven its worth across industries like healthcare, e-commerce, finance, and more. Yet even when implementation is technically feasible, that doesn't automatically make it the right move for your company. Without clear, specific goals, projects quickly become misaligned and lose focus. The data backs this up. A report from Harvard Business Review found that companies with clearly defined objectives are up to 3.5 times more likely to achieve successful outcomes.
Misalignment shows up in different ways. Leaders might optimize for the wrong metrics — like training a user churn prediction model based on vanity stats such as app downloads or broad customer satisfaction surveys. Or they might introduce complex features, like robo-investing tools, without proper user onboarding, only to see adoption rates lag.
💡 Don't pursue AI for the sake of "innovation" or "keeping up." Instead, focus on how AI can solve a well-defined business problem. That starts with crystal-clear communication: leadership must articulate the goals, desired outcomes, set specific, measurable objectives, and broader business context to the AI team. Engineers, in turn, should openly share the capabilities and limitations of different approaches to help manage expectations early on.
For example, your goals might be to cut customer service response times by 40%, boost predictive maintenance capabilities, or drive higher sales through personalized marketing.
The more tangible the target, the easier it is to keep projects on track. Revisit and refine OKRs regularly to make sure your AI initiative keeps delivering value as it evolves.
Engage with your customers and employees to unearth pain points and areas needing improvement. Use surveys, interviews, and feedback loops to uncover real pain points and areas ripe for improvement.
When building innovative AI-driven products, go deeper. Talk to stakeholders, analyze the market, and create detailed user personas. This groundwork not only sharpens your understanding of the problem — it ensures you're solving the right one.
Before you go all in, test your idea. Pilot programs and prototypes let you double-check gen AI readiness assessment and fine-tune your strategy based on real-world performance, not guesswork.
Additionally, companies achieve stronger outcomes when AI governance is actively led by the CEO or board of directors. This level of executive oversight is closely linked to improved financial performance. However, only 28% of companies currently report direct CEO involvement in AI governance.
And don't do it alone. Tap into AI consulting firms for expert guidance. A seasoned AI partner can help you vet your ideas, set achievable goals, and craft a strategy rooted in experience, not guesswork.
Ready to turn your AI vision into business value? Let's talk about how we can help you build high-impact AI solutions — without the usual risks.
#2. Lack of Problem Definition
Sometimes, AI projects flop not because the tech is bad — but because companies fall in love with the technology itself, forgetting the problem it's meant to solve.
There is a growing misconception that every product now needs to have AI baked in.
But at BotsCrew, we see it differently: AI should be a solution, not a status symbol. The real challenge isn't just adding AI — it is using it to solve the correct problems.
Take customer support, for instance. When generative AI exploded, many predicted it would replace support teams entirely. But in reality, AI typically resolves 30% of tickets. The rest still require a human touch. Because customer support isn't just about fast responses. It is about nuance, context, and trust. So companies are still hiring support agents, because the problem is more complex than AI alone can handle.
The ideal scenario is clear: a user has a problem, the business identifies it, and AI is the most effective solution in terms of cost, speed, and quality.
To get there, we need a shift in thinking. Instead of starting with: Here is some AI — what can we do with it? — We should be asking: What problem exists today that AI can solve better than anything else?
That mindset makes all the difference between AI that dazzles in demos — and AI that delivers real-world results.
#3. Lack of Industry-Specific Understanding
Every industry comes with its own playbook — specific standards, regulations, and challenges that require domain expertise. In healthcare, for example, compliance with HIPAA isn't optional — it's critical. Overlooking such regulations and ignoring Gen AI readiness assessment can compromise patient privacy and lead to massive financial and legal consequences.
In 2023, the U.S. Department of Health and Human Services (HHS) fined the Arizona-based Banner Health over $1.25 million after a data breach exposed the health information of nearly 3 million patients — stemming from insufficient risk assessments and failure to implement basic cybersecurity safeguards. Or consider the case of Excellus Health Plan, which agreed to pay $5.1 million for HIPAA violations related to a 2015 breach that impacted over 9 million individuals.
To steer clear of such risks, work with AI partners who know your industry inside and out. Look for providers with a proven track record in your sector — those who can point to successful projects, satisfied clients, and deep regulatory know-how. Don't hesitate to ask the tough questions: Have they worked with your industry's compliance standards? Can they share case studies showing how they navigated those requirements?
#4. Workflow Redesign
One of the most common missteps companies make with AI adoption is treating it like a plug-and-play solution — something you simply connect to your existing operations and expect transformative results. In reality, AI doesn't fix broken processes. Integrating AI into outdated or inefficient processes does little more than digitize inefficiency. It severely limits AI's transformative potential and risks turning a high-promise investment into a weak outcome.
How to avoid it:
✅ Redesign the entire workflow to avoid just incremental improvements, underwhelming ROI, missed strategic advantages, and frustration among teams.
✅ Make sure operational managers and process owners are involved early in the planning phase. Their domain knowledge is critical in reshaping workflows that align with both AI's strengths and business goals.
#5. Focus on the Latest Technology and Trends Without Testing Them
There is a growing temptation among businesses to chase the newest AI trends — rolling out the latest GPT model, adopting complex multimodal tools, or integrating bleeding-edge frameworks — without stopping to ask: Is this actually what we need? At the same time, a significant number of AI projects fail due to hasty implementation without proper alignment to business processes.
A simpler, more mature version might work just as well (or better), especially when budgets, infrastructure, or use case complexity are limiting factors. And when AI is chosen for its novelty rather than its business value, implementation quickly becomes messy, expensive, and underwhelming. Take for example a company that upgrades to GPT-4 Turbo to automate support chat, even though GPT-3.5 would have been more cost-effective, easier to control, and good enough for the task. The result? Over-engineering, ballooning costs, and delayed deployment.
How to avoid it:
✅ Prioritize Fit Over FOMO. Don't assume the newest model is automatically better for your needs. Start by defining your use case and desired outcomes — then choose the tool that balances performance, cost, and ease of integration.
✅ Validate Before You Upgrade. Test new versions on a small scale before fully adopting them. Compare output quality, latency, and compute requirements against the previous model to see if the gains are truly worth it.
✅ Work with Pragmatic Partners. Choose custom AI development companies or consultants who push back on unnecessary complexity and help you stay grounded in ROI — not hype.
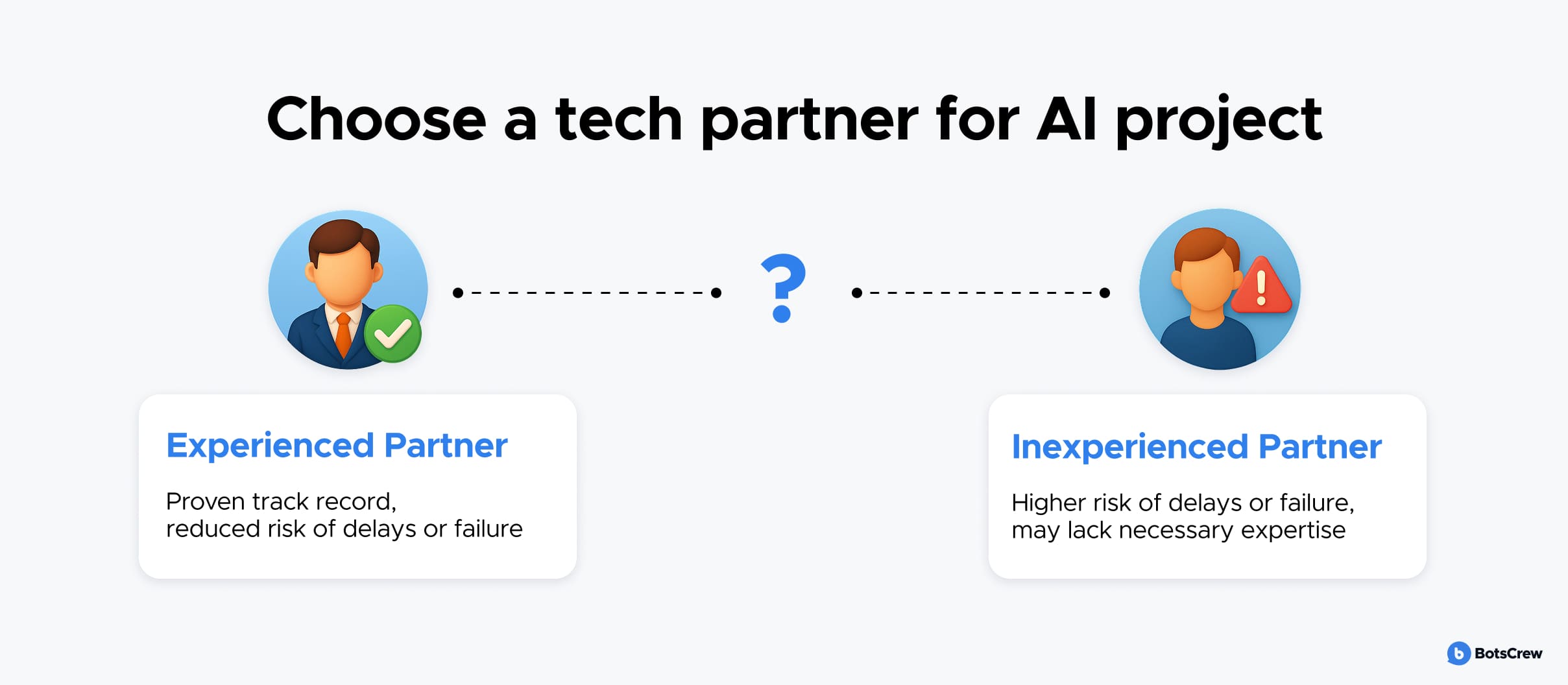
Edtech giant Preply, used in over 150 countries, began integrating AI in the summer of 2023. While testing various APIs — ChatGPT, Anthropic, Bard, and open-source models — Preply found that the older version of ChatGPT, GPT-3 Turbo, actually served their needs better than the newer GPT-4.
Though GPT-4 offered top-notch quality, its high latency made it impractical for fast-paced tasks like preparing homework right after class. In the end, GPT-3 Turbo struck the perfect balance of quality and speed, making it the best fit for their tutors.
#6. Underestimating Data Quality
Most enterprise AI systems run on machine learning — and machine learning entirely depends on the quality of your data. Feed it weak, messy, or misaligned information, and you risk building confident-sounding models that are flat-out wrong. Worse yet, if you are using the same public data as everyone else, don't expect any competitive edge.
How to fix it:
✅ Start with data discovery. Create a detailed catalog of your enterprise AI readiness and information assets — where they live, who owns them, how sensitive they are, and what shape they are in. This metadata matters: it gives your team the context needed to clean, transform, and use data responsibly.
✅ Build trust through security and privacy. Mask sensitive data, encrypt it in transit and at rest, and lock down access based on roles. Implement model version control to prevent exposure of regulated data and track what goes live.
Kravet, the global icon in luxury interiors, asked us to tame their data jungle — 10,000+ products in Algolia, scattered inventory details, and over 1,000 static docs across Sales, Ops, Supply Chain, and HR. The AI assistant flopped on unstructured data — just 4 out of 10 answers hit the mark.
Files contained conflicting and outdated information, data with content in unreadable format (e.g., scans) was not aiding the AI responses. Additionally, product-related information located on the Kravet website couldn't be fully accessed and did not enable exact-match search by the product SKU code. Each time, AI utilized a different knowledge source that could provide a wrong answer that was previously handled correctly.
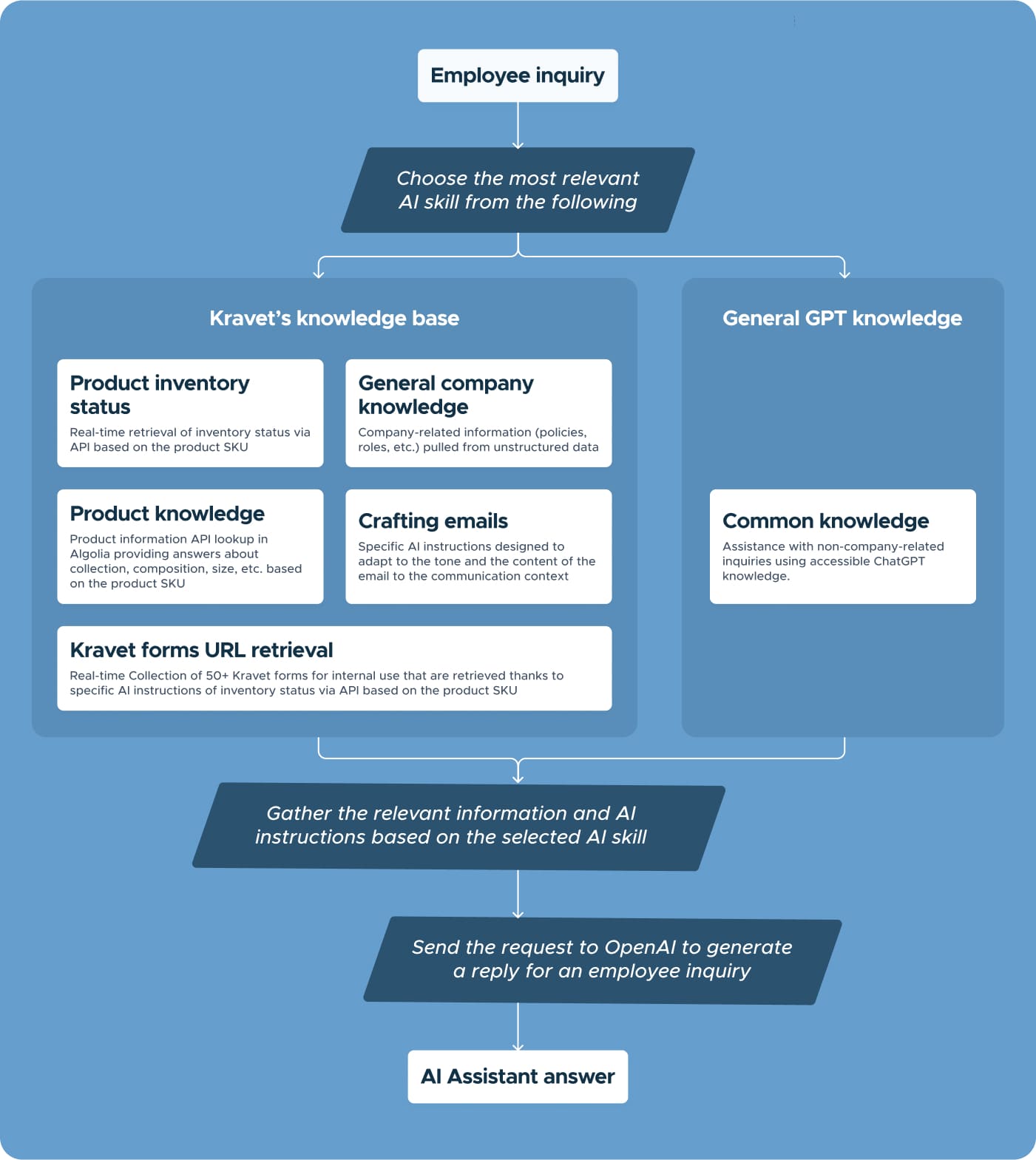
We kicked off with a quick, zero-risk pilot: a lean proof-of-concept using the BotsCrew Enterprise Platform with built-in RAG magic. In just 3 weeks, the prototype was live. The results? A smarter assistant that now handles 10,000 products with ease—covering colors, collections, fabrics, and features. Accuracy jumped from 60% to over 90%. Now 8 out of 10 responses are spot on.
Unsure whether your data is ready for AI? Begin with a Discovery Phase to evaluate data readiness, identify opportunities, and define a strategic roadmap for effective AI implementation.
#7. Underestimating the Complexity of AI Model Development
While traditional software follows a more linear path from idea to deployment, AI development is rather experimental and intensely collaborative. And if you treat it like any other IT project, you're almost guaranteed to fail.
It starts with problem framing and identifying the use case — and that's where the first cracks often appear. Business stakeholders might describe the goal vaguely ("reduce churn" or "optimize operations"), but without clearly defined success metrics, use cases, or constraints. Engineers are then left guessing what "success" really looks like, often chasing goals that evolve mid-project.
Next comes data wrangling — a stage that is never as straightforward as pulling numbers from a database. The data needs to be cleaned, annotated, balanced, and preprocessed. Sometimes, you'll need entirely new data sources to avoid biased or incomplete models.
Then, there is the modeling and integrating phases, which are iterative and resource-intensive. You are not just building one model — you're building dozens of variations, tuning hyperparameters, adjusting inputs, and retraining from scratch when things go sideways. It can take weeks (or months) of experimentation before your model even resembles something usable.
And even then… it might never see the light of day. According to Gartner, only 22% of AI models that unlock new business capabilities actually make it to deployment. And nearly 43% of teams admit they fail to deploy over 80% of their AI projects.
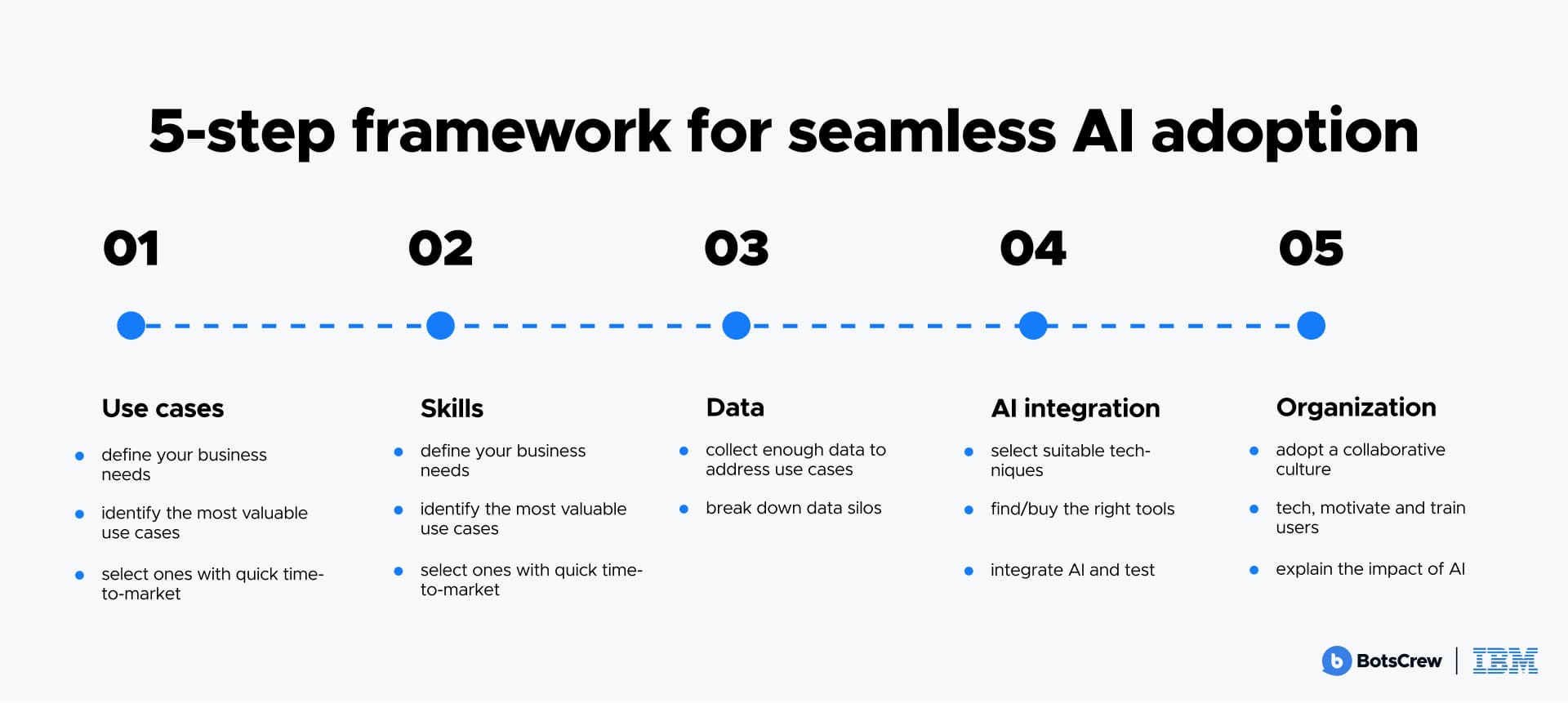
How to avoid the trap:
✅ Involve business and tech teams from day one. Keep the feedback loop tight and frequent — AI success depends on co-creation, not handoffs.
✅ Prototype early and often. Validate the model in a controlled environment before going all-in on development.
✅ Plan for the last mile. From security reviews to API integration and user onboarding, deployment needs just as much attention as development.
✅ Build for iteration. Your first model won't be your last — optimize for adaptability, not a one-shot journey.
We partnered with a leading cosmetics brand and helped them save $20,919 in just 30 days. Facing skyrocketing support volumes and growing wait times, the company needed a smarter, faster way to serve its customers.
With over 1.1M monthly website visitors and support inquiries growing by 282% annually, their customer service team was stretched thin. Long wait times and rising volumes called for a smarter solution.
That's where Legendary.cx contacted BotsCrew — our 30-day, zero-risk automation offer designed to bring Fortune 500-level AI to high-growth brands. Within one month, we automated 30% of support requests, cut resolution times, and saved nearly $21K — powered by GPT and our custom-built solution.
#8. Poorly Collected Requirements and Lack of Success Metrics
One of the fastest ways to derail an AI initiative is to dive in without knowing what success actually looks like. If your team can’t answer questions like "How will we know it’s working?" — stop right there.
Too often, organizations build AI tools that surface insights no one uses, because decisions are still made on gut instinct. And when success is not clearly defined upfront — be it increasing average order size, reducing churn, or improving resolution time — AI projects quickly lose direction.
Worse yet, many companies focus on minimizing short-term costs, missing the bigger picture. AI is a long game. It requires investment, experimentation, and iteration. If you're budgeting like it is a one-and-done project, you are setting yourself up to fail.
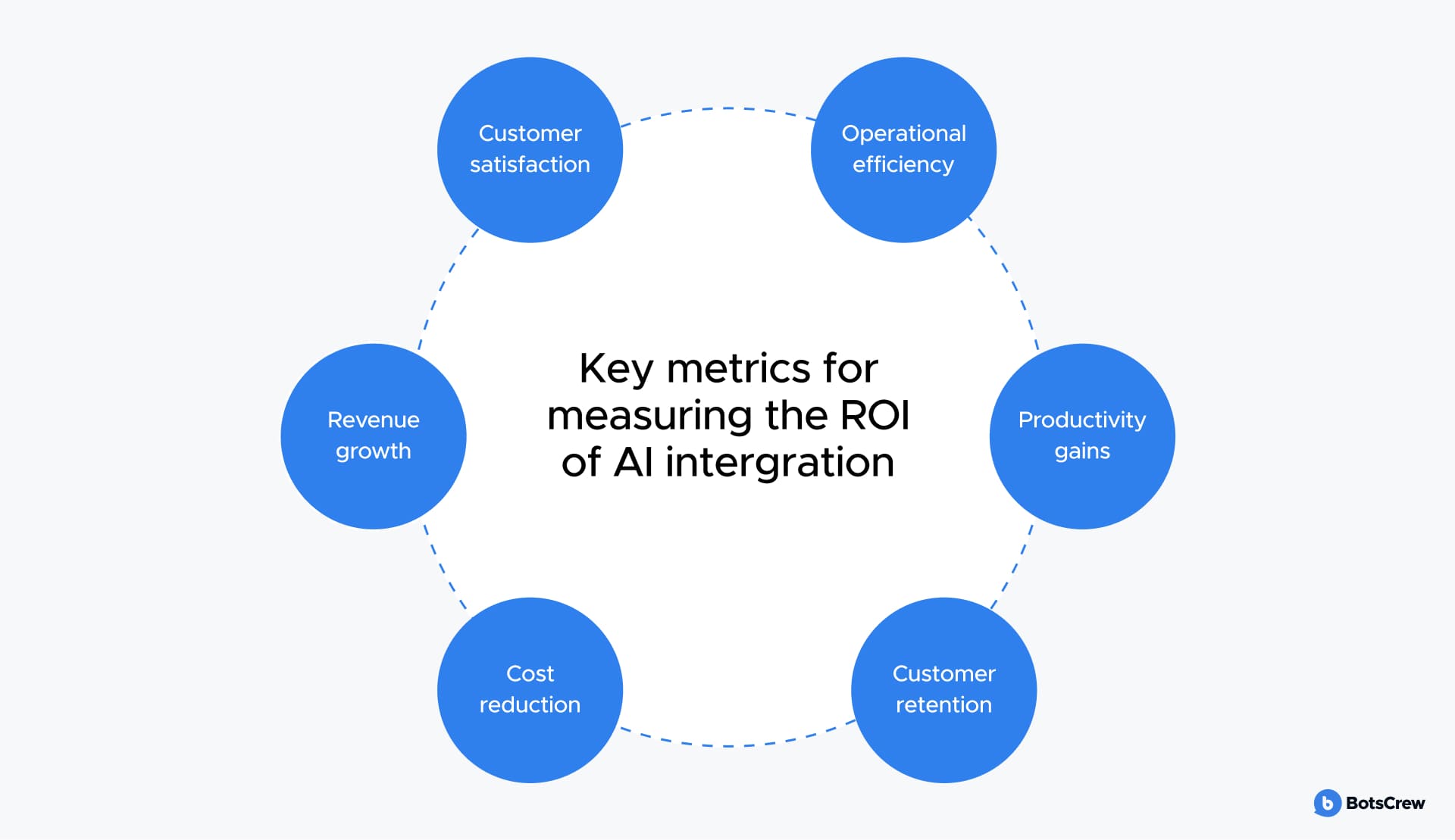
How to avoid the trap:
✅ Define your North Star metrics early. Tie AI outcomes to real business KPIs: conversions, uptime, resolution speed — not vanity metrics.
✅ Adopt a test-and-learn mindset. Start lean with a discovery phase, iterate fast, and use each cycle to refine both your product and your goals.
✅ Measure ROI beyond dollars. Track gains in efficiency, user satisfaction, and competitive edge — these often bring compounding returns over time.
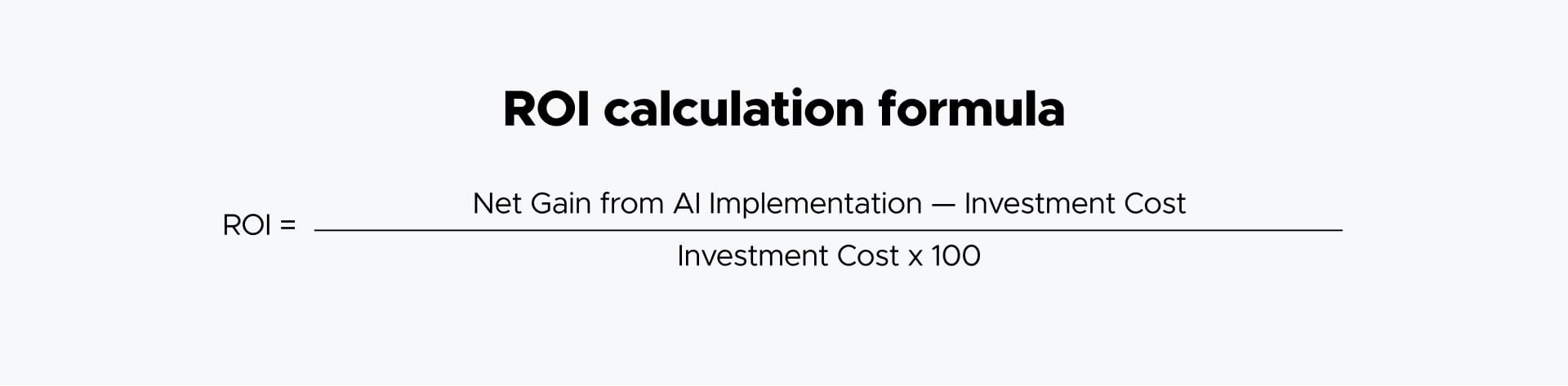
#9. Ethical and Regulatory Pitfalls
From biased hiring bots to AI chat tools spewing offensive content, ethical and regulatory misfires in AI aren't rare — they are regular. Every PR disaster chips away at user trust while regulators are sharpening their teeth: over 60% of AI-investing leaders say responsible AI is now a top priority.
How to build AI you can stand behind:
✅ Bias-proof your training data. Use techniques like synthetic data augmentation and fairness-aware algorithms to ensure diverse, equitable representation across age, gender, ethnicity, and other dimensions.
✅ Treat privacy as non-negotiable. Apply anonymization and explore advanced tools like homomorphic encryption (which allows computations on encrypted data) and differential privacy (which ensures individual users can't be reverse-engineered from outputs).
✅ Run risk checks early and often. Perform model audits, assessment for AI readiness, and lifecycle risk assessments to flag issues before they scale — not when they hit the headlines.
✅ Take ownership. Make sure there is always someone accountable for the AI's decisions and impacts — and be ready to explain how and why your model behaves the way it does.
#10. Not Being Able to Scale the Infrastructure
You've built a working AI prototype. It runs great in a test environment. But what about the moment you try to roll it out across the company? Everything crashes — performance drops, latency spikes, costs balloon, because your infrastructure wasn't built to scale.
⚠️ Common Scaling Pitfalls:
— Underestimating compute and storage needs. Training and running modern models — especially LLMs or deep learning systems — requires significant resources. A single model iteration can demand more GPU hours than your team accounted for in a year.
— Lack of clear cloud strategy. On-prem servers might handle dev work, but they can't flex with real-time demand. Without elasticity, your system buckles under pressure.
How to Build for Scale:
✅ Go cloud-native from day one. Platforms like AWS SageMaker, Azure ML, and GCP Vertex AI offer auto-scaling, managed model hosting, and built-in monitoring.
✅ Design for growth. Build architecture with modular components that can scale horizontally (more users, more data) and vertically (more compute power per process).
✅ Automate monitoring and retraining. Use MLOps pipelines to streamline deployment, track performance, and trigger retraining when models start to drift.
#11. Ignoring Team Upskilling
Even with a well-integrated AI solution and redesigned workflows, there is one more critical piece of the puzzle: your people. Many companies overlook the human factor, assuming that AI will "just work" once deployed.
Teams that lack proper training either underutilize the tools or actively resist them, leading to poor adoption, costly mistakes, and a growing gap between AI's potential and its actual business impact. Worse yet, this can create a culture of fear and confusion around AI — where employees feel threatened by automation rather than empowered by augmentation.
To avoid this, organizations should consider:
✅ Ongoing training programs that go beyond technical skills and teach employees how to collaborate with AI, not compete with it.
✅ Provide employees access to hands-on workshops that demystify AI tools, show use cases relevant to specific roles, and build confidence through practical application.
✅ Change management support to help teams shift from old habits to new AI-augmented workflows.
✅ Involving teams in the AI improvement loop, giving them a voice in feedback cycles so they’re co-creators, not just end-users.
When employees understand how AI works, what it can (and can't) do, and how to shape its outputs, they become powerful multipliers of its value. But if you skip this step, even the best AI will end up underperforming — because the human system around it wasn't ready.
#12. Expecting AI to Replace Humans
The fantasy of fully autonomous AI still sells headlines — but in the real world, it's human + machine that wins. The best results don't come from replacing people, but from augmenting them.
According to Harvard Business Review, companies that leaned into human-AI collaboration saw productivity boosts of 4x to 7x. Therefore, involve your business teams early. Let them help define what AI should do with them, not instead of them.
What Actually Works:
✅ Decision support over automation. AI that recommends actions, flags anomalies, or ranks options empowers humans to make better decisions — not disappear from the process.
✅ Built-in escalation paths. When AI can't handle nuance, it should hand it off to a human expert. That's especially critical in fields like customer service, healthcare, or compliance.
✅ Explainability and trust. People won't follow AI suggestions if they don't understand how the system got there. Transparent, interpretable models are key to adoption.
💡 How BotsCrew Can Help
Ready to succeed with AI? Hire experts to minimize AI integration mistakes. With over 8 years of experience and a portfolio boasting successful AI integration projects across various industries and for Fortune 500 companies, we have the knowledge and skills to guide you through your AI journey.
At BotsCrew, we've assembled a team of top-notch specialists ready to assist you with AI integration, creating a successful AI strategy, custom AI product development, ML engineering, and the creation of chatbots and virtual assistants. Our experts understand the intricacies of AI technology and have a proven track record of delivering innovative solutions tailored to our clients' specific needs.
How we help our clients avoid common pitfalls and drive sustainable success:
🎯 Strategic Alignment. We work closely with your leadership teams to translate business goals into AI use cases that deliver measurable ROI.
💡Fortune 500 Level of Expertise. Leverage the knowledge of teams that have been building AI for Samsung NEXT, Honda, Mars, FIBA, the International Committee of the Red Cross (ICRC), Adidas, and others.
🤝 Cross-Functional Integration. We embed with your teams — business and technical — to break down silos and foster seamless collaboration.
🧭 Ethical AI by Design. We incorporate explainability, fairness, and transparency from day one so your AI not only works — but earns trust from regulators, stakeholders, and users alike.
✅ Unparalleled Support. You are not alone in this journey! A dedicated team and prioritized support at all stages comes in a package along with cutting-edge technology.
Don't let common AI mistakes hold your business objectives back having at least minimal impact on your success. Trust BotsCrew to help you implement a successful AI, build a solid AI strategy, and drive success.
Learn more about our AI development services and explore our impressive portfolio of projects here. Let's unlock the full potential of AI together.
Let's discuss your unique AI use case and find the perfect solution. We'll analyze your use case, suggest a tailored AI solution, and guide you through a live demo to show how it fits your needs.