Chatbot Analytics: 14 Chatbot Metrics To Track in 2024
In this article, we want to cover the topic of chatbot metrics and KPIs you need to track to ensure chatbot success. Here you will find the 14 most important metrics to keep an eye on in 2022.
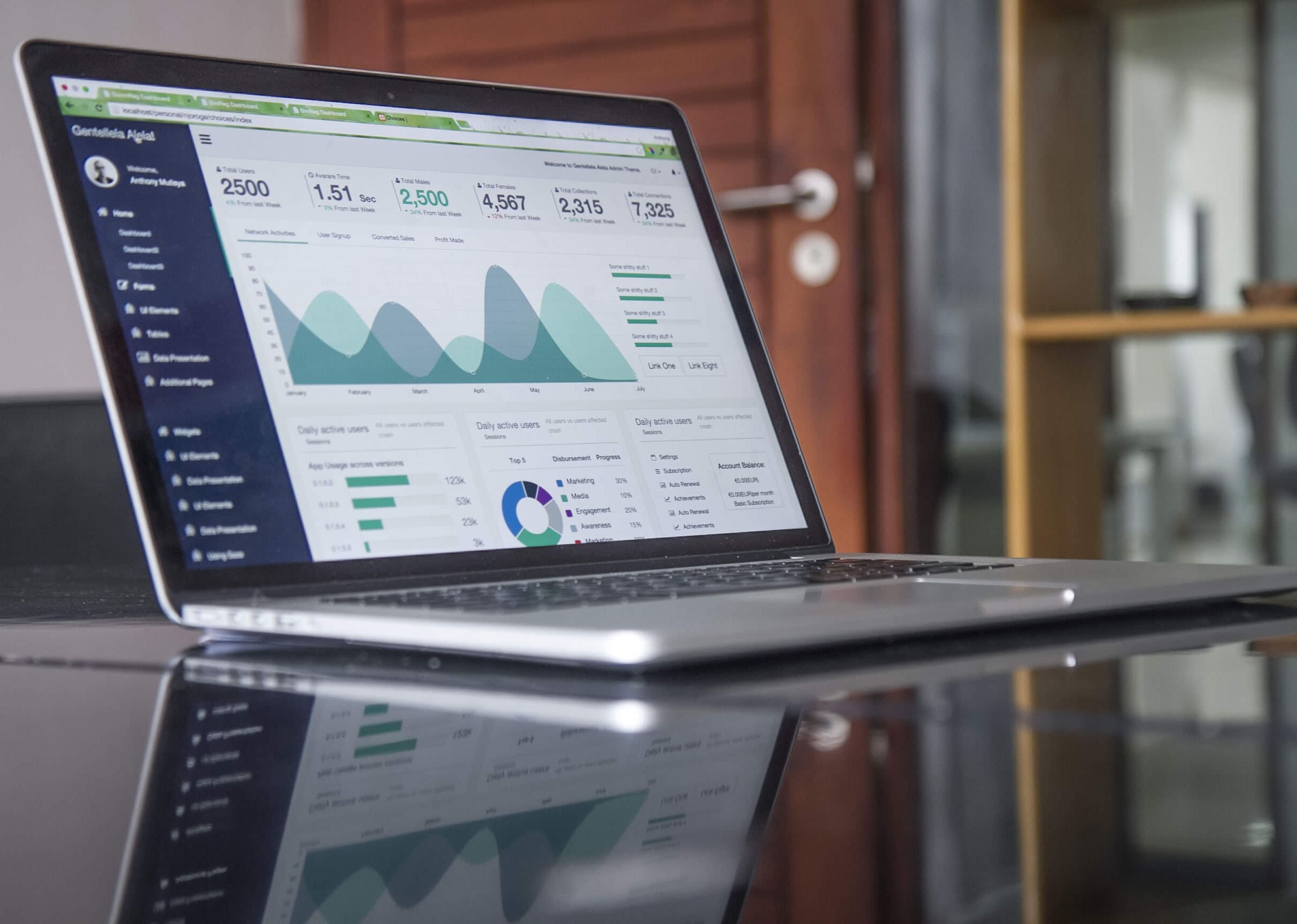
Chatbots are not the "set and forget" thing like many other software. If you want to achieve great results with your chatbot, you need to improve it constantly. (In case your chatbot operates in the customer support field, check out our research on how to improve customer service automation.) To know where and what to improve, you need to track and monitor chatbot analytics and the main chatbot metrics. It can be quite hard for someone who has not much experience to figure out which chatbot metrics to track and how to do it properly. We decided to share our 5+ years of experience in chatbots and tell you about the crucial chatbot metrics to keep an eye on.
Let’s dive in!
Which chatbot metrics to track?
The important thing to understand is that you don't need to track all of them. Some of these metrics may be redundant for your industry or use case. So, don't worry if not all of these metrics will suit your chatbot. We indicated which metrics are best suited for each use case for your convenience.
General chatbot metrics
- Total number of users
- User satisfaction
- Accuracy of the chatbot
Engagement metrics
- Active users
- New users
- Conversation Length
- Retention Rate
- Bounce Rate
- Flow Completion Rate
Conversational analytics
- Goal Completion Rate (GCR)
- Fallback Rate
- Human Takeover Rate
* Bonus: Revenue metrics
- Revenue generated
- ROI / payback period
General chatbot metrics
Total number of users
Use case: Customer service, Marketing, Sales
Importance: High
It’s a very basic metric, but it gives you an understanding of how popular your chatbot is. Also, you can use this metric to calculate other metrics like conversion rate if you have a website chatbot. For example, you can take the total number of chatbot users in 2021, divide it by the total number of your website visitors and figure out how “attractive” your chatbot looks to your website visitors. Here’s how it looks:
You had 50,000 website visitors in 2021, and you had 6,000 chatbot users in 2021. You need to divide your chatbot users by website visitors - 6000/50000= 0.12, then multiply your result by 100 - 0.12*100 = 12%.
This result is your conversion rate from a website visitor to a chatbot user. If this conversion rate is very low it may mean that chatbot looks unattractive, your welcome message looks unengaging, or simply users don't notice your bot because of the website or widget design.
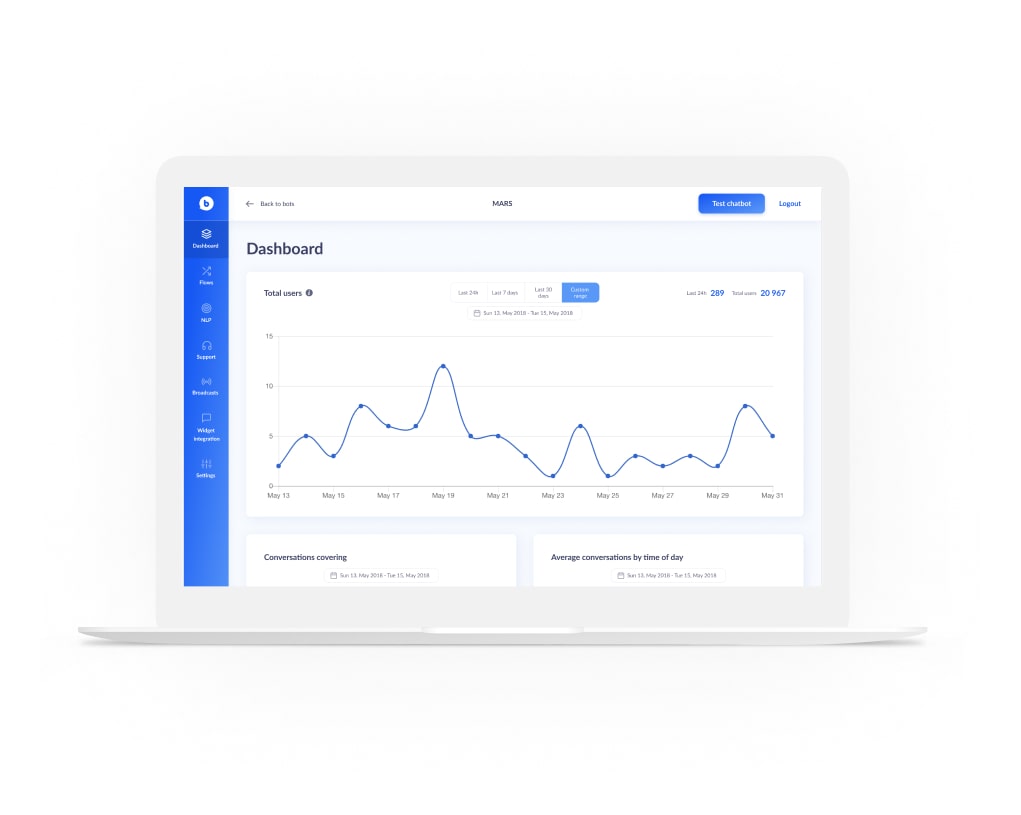
User Satisfaction
Use case: Customer service, Marketing, Sales
Importance: High
The user satisfaction metric shows how users rate your chatbot and if they find your chatbot useful or engaging. Usually, you can measure user satisfaction by doing surveys at the end of the conversation. You can also track user satisfaction during a conversation after some replies that a chatbot performs. However, don't overload the chatbot with surveys and rating options. Just focus on the most critical times when you want the users to rate the chatbot.
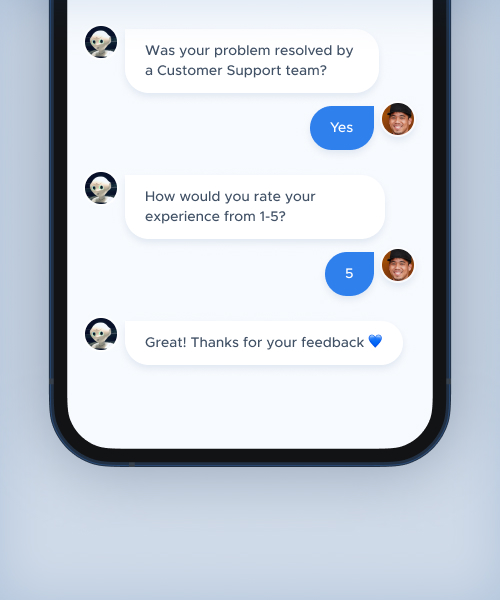
Accuracy of a chatbot
Use case: Customer service, Marketing, Sales
Importance: High
This metric is relevant only for AI and NLP-powered chatbots. These chatbots allow users to type in their requests, questions, and answers manually. They interpret user input and provide a response using Natural Language Programming skills. AI and NLP-powered chatbots require constant training. That is why you need to measure their Accuracy. With rule-based chatbots, you don't need to focus on the accuracy metric because they provide users with buttons and strictly pre-defined flows to communicate with them. If appropriately built, there can't be any inaccuracies with rule-based chatbots.
The accuracy of the chatbot metric answers 2 questions:
- Did the chatbot understand what the user was writing?
- Did the chatbot provide the correct answer?
Accuracy is the baseline requirement of your AI-powered chatbot. Without it, hopes of reducing customer friction and accelerating revenue aren’t realistic.
How to calculate the accuracy of a chatbot?
The easiest way is to check the total number of messages sent from the chatbot and check how many of them were Fallback messages. Deduct Fallback Messages from the total number of messages, then divide the number you got by the number of total messages and multiply it by 100.
Here’s an example:
You got 8,000 messages. 750 out of those messages were fallback messages.
So, here’s what you do:
8,000 - 750 = 7,250
7,250/8,000 = 0,906
0,906*100 = 90,6%
90,6% is your accuracy score in this example.
What is good accuracy for a chatbot?
We recommend aiming for an 80% or higher accuracy score.
Engagement metrics
Active users
Use case: Customer service, Marketing, Sales
Importance: High
This metric shows a count of unique users who send a message in a defined time frame. As well as with total users, you can track the active user's number by itself or calculate the percentage of active users out of total users, which will give you the broader picture.
New users
Use case: Marketing
Importance: Medium
This metric shows the number of unique first-time users in a defined time frame. New users metric helps to measure marketing or other bot promoting efforts, for example, if you launch a new ad campaign that features your chatbot.
Returning users
Use case: Customer service, Marketing, Sales
Importance: High
Returning users are the people who had communicated with your chatbot before and returned to communicate with it again. The higher the number of returning users, the better because this means that users find your chatbot useful or engaging.
Average conversation duration
Use case: Customer service, Marketing, Sales
Importance: Medium
This metric shows the total duration of all conversations (in seconds) during a specified time frame by the total number of conversations during that same time frame.
Conversation duration is a time from the user's first message to the bot's last message, and it should be calculated after the conversation ends. There is no benchmark for this metric because it's very individual. Don't think that the longer the conversation duration with your chatbot, the better because it can mean that your chatbot is complex, and people need much time to find the answers they are looking for. Short conversation length doesn't mean the chatbot underperforms. Maybe the users find the information they need in your chatbot fast, or you may have a short conversational flow. So I wouldn't make any major decisions based only on this metric.
For example, a Facebook chatbot Poncho provides information about the weather. Usually, his conversations with users can be concise, but having long conversations wasn't his goal.
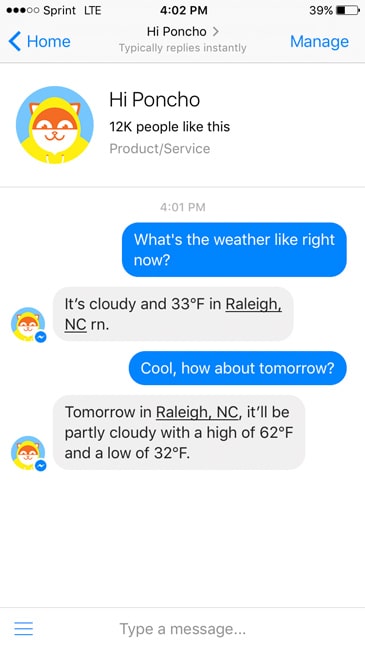
Bounce Rate
Use case: Customer service, Marketing, Sales
Importance: High
Bounce rate is the percentage of users who enter the chat and leave without interacting with the chatbot. Your aim should always be to have as low bounce rate as possible. A high bounce rate shows the chatbot fails to provide correct answers, helps users with their requests, or is not engaging enough.
Flow completion rate
Use case: Customer service, Marketing, Sales
Importance: High
The percentage of users who entered the flow completed all the steps and received the last message. You can calculate this metric by dividing the flow completions by the number of flow initializations.
Conversational analytics
Goal Completion Rate (GCR)
Use case: Customer service, Marketing, Sales
Importance: High
This chatbot metric captures the percentage of successful engagement through a chatbot. GCR indicates how many of your users reached their or your goals. For example, your goal could be for a user to finish the chatbot flow, subscribe to your services, book a meeting with you, resolve a customer issue, etc. The GCR metric shows how successful your chatbot was in helping users reach these goals. If your GCR is low, don't panic just yet. Try to find the reason why the chatbot isn't reaching your goals. Maybe the conversational flow is too confusing or lengthy. Perhaps the goal you set isn't naturally expected or logical at that point. Just analyze your user chatbot journey, and you might find what's stopping your users from reaching the goals you designed.
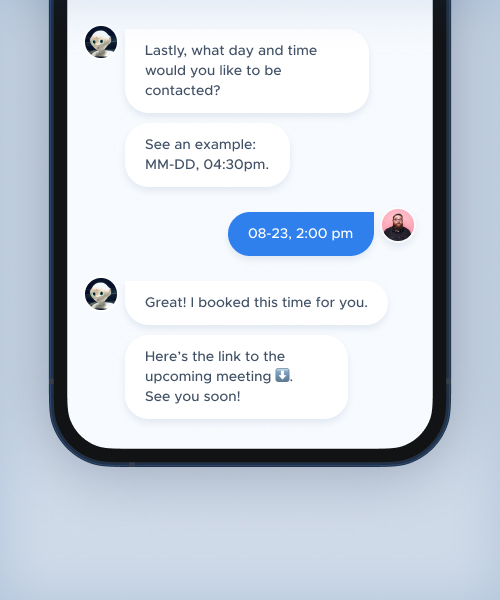
Fallback Rate
Use case: Customer service, Marketing, Sales
Importance: High
This metric measures the percentage of messages when the bot didn't get user intent or failed to provide an answer to the user's question. This metric is important because it helps to understand how often the bot has no answer and find areas for improvement.
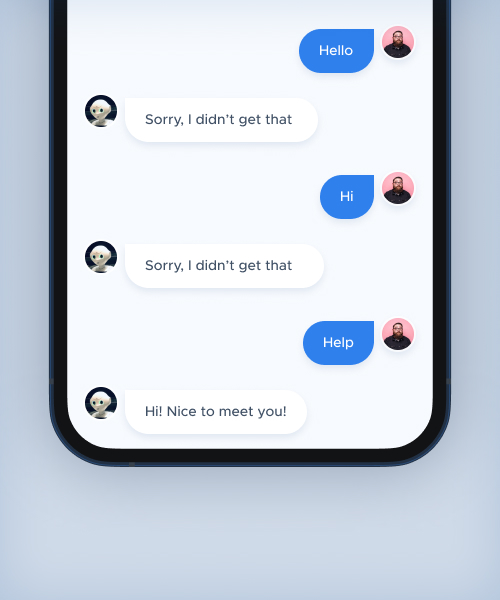
Human Takeover Rate (HTR)
Use case: Customer service
Importance: High
This metric measures how often your human agent had to jump in the conversation to help the user. For example, if your agent noticed that the user is getting "Sorry, I didn't get that" or "It seems like I can't help you with that" chatbot reply, your human agents can connect to the conversation and help the user from there. If your HTR is too high, you need to train your chatbot more, as it doesn't reach its goals. However, in some cases connecting to a human is a logical and expected next step, for example, if you want your users to chat with your sales or other professionals.
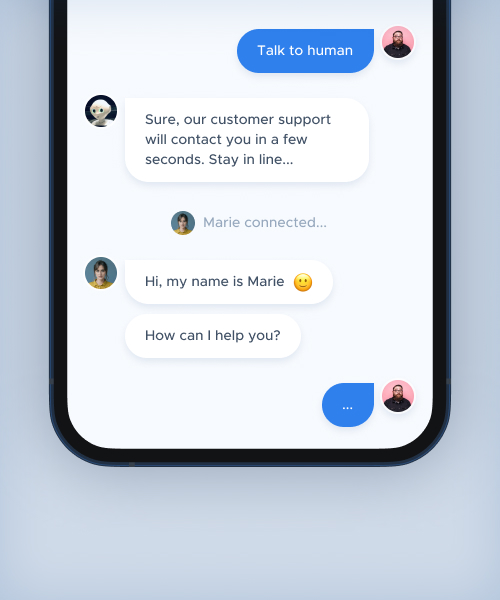
Revenue-oriented metrics
Revenue generated
Use case: Marketing, Sales
Importance: High
If your chatbot's primary goal is sales, it can bring revenue to your business. By calculating chatbot ROI, you can measure how much revenue was brought by a chatbot. The chatbot can make sales directly during the chat with the user (common for e-commerce chatbots) or transfer the user to a human sales agent who closes the deals. In both those cases, the chatbot helped you generate revenue, and it's better to track these results. Also, this revenue-generated metric allows you to calculate the next chatbot KPI we will talk about - ROI.
ROI / payback period
Use case: Customer Service, Marketing, Sales
Importance: Medium
This metric tracks the monetary value from the bot (for example, time saved for customers and support agents or customers brought by the chatbot). ROI is an important metric because it shows whether you should invest in future chatbot optimization.
Chatbot Analytics Tools
At this point in the article, you may be wondering if you have to measure and keep track of all these chatbot metrics by yourself.
Well, first of all, many chatbot analytics tools can help you with that. Secondly, you probably have a chatbot analytics dashboard if you use a chatbot platform. Thirdly, if you use custom chatbot development services, you can talk to your chatbot developers to either set up this analytics dashboard or ask them to track these metrics for you.
Here are a few Chatbot Analytics Tools that you can use:
- Botanalytics
- BotCore
- BotExpress.ai
- ChatBot
- Chat Metrics
- Dashbot
- Kore.ai
But as we mentioned before, if you use or are planning to use a chatbot platform, first research the chatbot analytics dashboard they provide, as you may not need to set up additional analytics tools. And if you use chatbot vendors, check in with them which chatbot analytics tool they recommend and if they can set it up for you.
How do I optimize my chatbot?
After you figure out which metrics to track and what analytics tool to use and start keeping track of these chatbot KPIs, you need to optimize your chatbot based on those things. Depending on the metrics that don't perform well in your chatbot, you'll have different optimization strategies. It often comes to training your chatbot and its NLP more or reviewing your conversational flow to make it simpler or more engaging. Check out our article on training chatbots for non-technical people to learn more about NLP training.
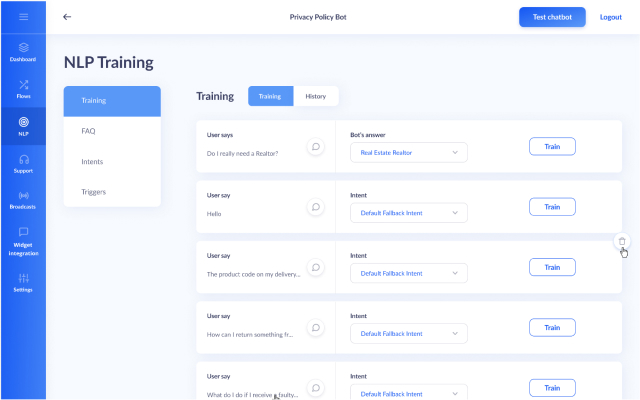
There is no universally applicable advice we could give you regarding chatbot optimization. It is better to talk to chatbot experts to reach the best results. Talking to experienced conversational designers or business analysts specializing in chatbots is the easiest and most effective way to improve your chatbot. You can book a free consultation with us if you'd like to get an expert view on chatbot and its KPIs.
Get your free table with 25+ chatbot metrics! All of the most important KPIs gathered in one place