How AI Is Transforming Medical Billing and Coding
Discover how AI is revolutionizing medical billing and coding by reducing claim denials, cutting administrative costs, and boosting accuracy. Learn how healthcare providers use AI and generative AI to automate coding, optimize revenue cycles, and ensure compliance—with real-world case studies and practical tips for implementation.
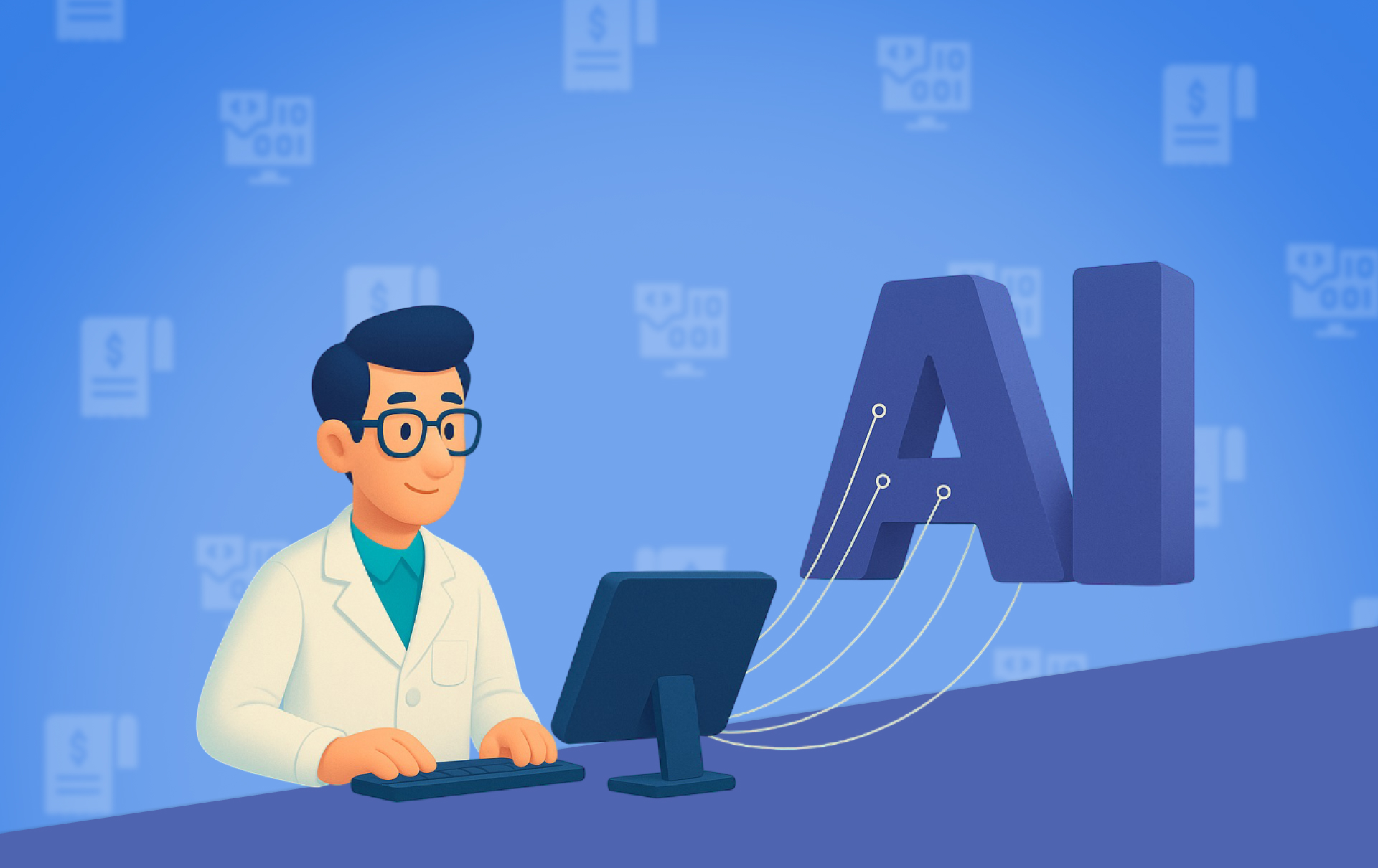
Medical billing and coding represent the financial backbone of healthcare organizations, yet these critical processes face significant inefficiencies, labor shortages, and costly errors. Astonishingly, administrative tasks—primarily billing and coding—consume up to 30% of healthcare spending. Fortunately, Artificial Intelligence (AI), including generative AI, is dramatically transforming these processes, offering substantial efficiency gains and cost savings.
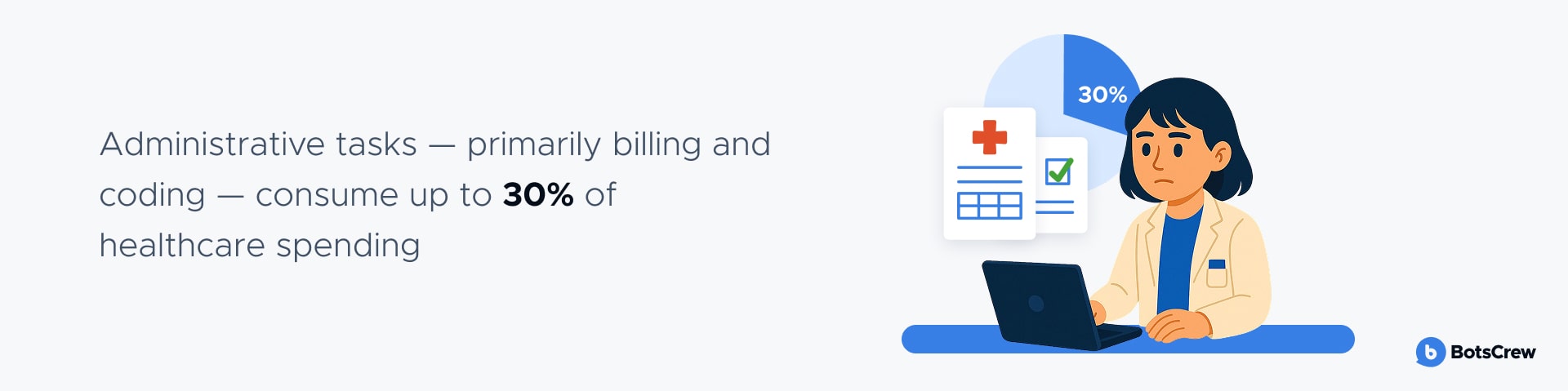
From reducing claim denials to automating routine tasks, AI in medical billing and coding is reshaping healthcare operations. This article explores the transformative power of AI—including generative AI—and how custom solutions from BotsCrew can streamline revenue cycle management while cutting costs and boosting accuracy.
The High Cost of Billing & Coding Today
Healthcare organizations face mounting challenges with traditional billing and coding processes.
Administrative Overhead
Billing and coding are highly labor-intensive tasks, involving the interpretation of massive volumes of patient data, insurance policies, and procedure codes (ICD-10, CPT, HCPCS). With constantly changing payer rules and frequent updates to code sets, keeping up is a full-time burden. Administrative processes account for roughly 25–30% of total healthcare spending, a staggering figure that drains resources away from patient care. Industry reports show hospitals spend tens of millions annually on billing administration—costs that could be significantly reduced with smarter technologies.
Costs & Inefficiencies
Manual billing and coding not only slow down the revenue cycle but also expose providers to frequent mistakes. Slow billing cycles delay reimbursements, weakening cash flow, while errors in coding lead to costly claim denials. Studies show that up to 90% of claim denials are preventable, with coding errors cited as a leading cause. Each denied claim not only delays revenue but also demands additional administrative effort to correct and resubmit, multiplying operational costs.
the cost of medical billing errors in the U.S. healthcare system
Workforce Challenges
In 2025, approximately 30% of medical coding positions remain unfilled, reflecting a significant talent gap. High turnover rates, burnout, and repetitive workload pressures exacerbate the shortage, leaving billing departments overstretched and vulnerable to errors. As coding demands grow in complexity, the gap between the volume of work and available skilled staff continues to widen, making automation an urgent necessity.
Without modernization, the "old way" of handling billing and coding is unsustainable—posing financial, operational, and compliance risks. Organizations that fail to address these medical billing challenges risk falling behind in an increasingly competitive healthcare landscape.
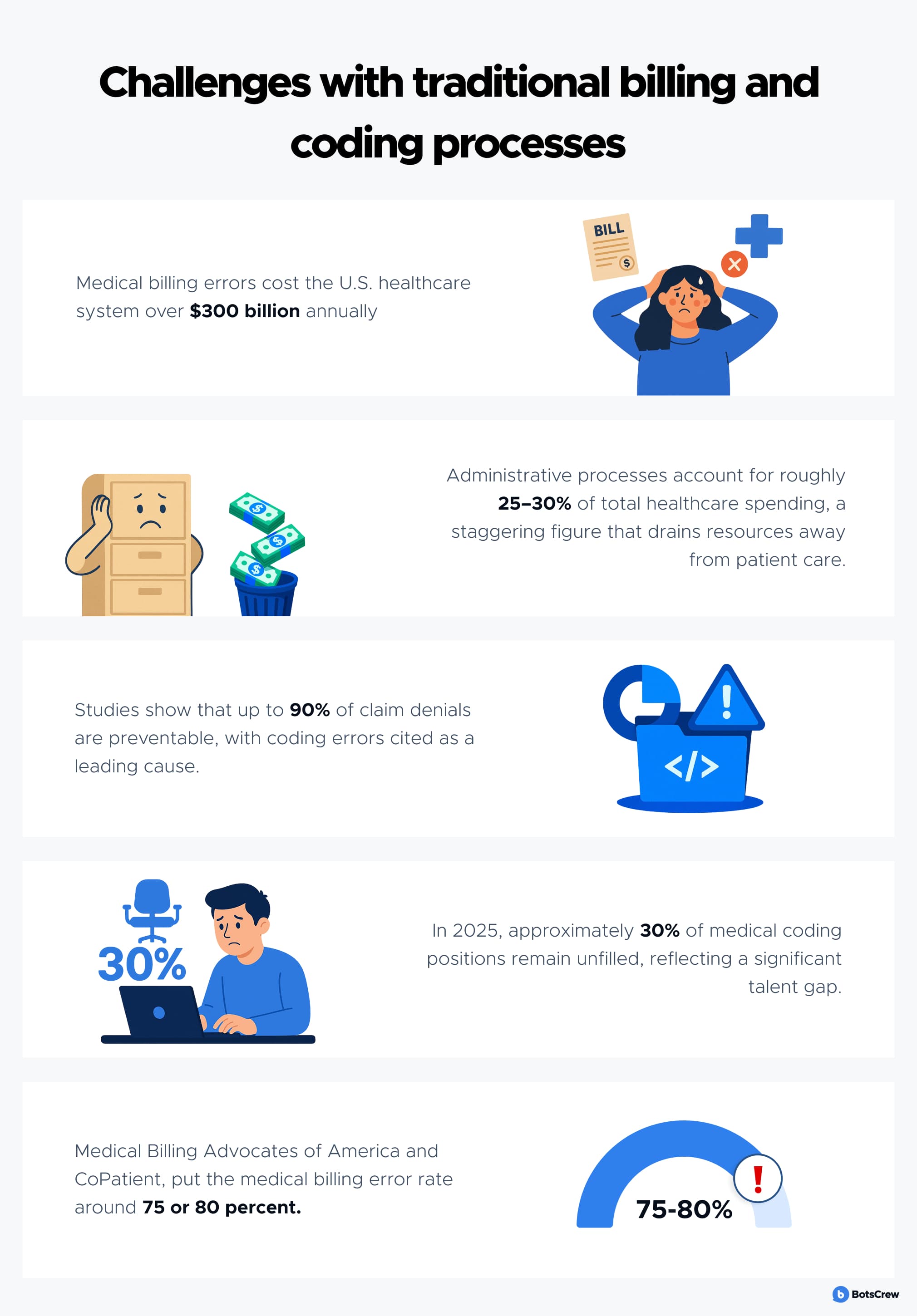
How AI and Medical Coding Work Together
Artificial intelligence (AI) is revolutionizing medical billing and coding by automating routine tasks, improving accuracy, reducing costs, and enhancing compliance. Healthcare providers embracing AI gain significant operational advantages and ensure stronger financial health. Below, we explore key applications of AI, showcasing real-world benefits and practical examples from industry leaders.
1. Automated Medical Coding
Manual medical coding is time-consuming and error-prone, leading to costly claim denials. AI-powered automation changes the game by accurately interpreting clinical documentation, dramatically improving accuracy and efficiency.
How It Works:
AI uses Natural Language Processing (NLP) technology to automatically interpret physician notes and electronic health records (EHR) documentation. It accurately translates medical terminology and clinical descriptions into standardized ICD-10, CPT, and HCPCS codes, significantly reducing the need for manual coding.
Benefits:
- Higher Accuracy: Minimizes human errors that commonly cause claim denials.
- Increased Productivity: Dramatically speeds up the coding process, allowing coders to focus on more complex cases.
- Cost Reduction: Cuts overhead by reducing manual coding labor.
of medical bills have at least one error
Real Example:
Case Study – Geisinger Health System (U.S.):
Leveraged AI-driven NLP technology to automate coding for radiology reports, achieving a 98% accuracy rate, significantly reducing administrative coding time and costs. Reallocated 5 FTE resources to higher-value work and reduced costs by 90% to resolve eligible duplicate patient records.
2. Claims Management and Error Reduction
Claim errors are a leading cause of payment delays and lost revenue. Claim denials remain one of the biggest financial burdens for healthcare providers, with studies showing that denial rates can reach up to 15% of total claims submitted. AI-driven solutions proactively identify and correct errors before submission, streamlining the revenue cycle.
How It Works:
AI-driven platforms automatically validate claims data, checking for accuracy and completeness prior to submission. The technology identifies potential errors, inconsistencies, or missing information and alerts billing staff to correct issues proactively, thus increasing the likelihood of claim acceptance on the first submission.
Benefits:
- Reduced Claim Denials: AI-driven checks proactively catch errors, decreasing denial rates by up to 30%.
- Improved Revenue Flow: Fewer denials mean quicker payments, improving overall cash flow.
Real Example:
Example – ENTER.Health's:
AI-powered Revenue Cycle Management (RCM) platform offers a proactive approach to claims management. By intelligently scrubbing claims before submission, reconciling payments, and auto-generating appeals, ENTER empowers healthcare organizations to protect revenue and reduce wasteful rework. Their system has helped reduce billing errors by up to 40%, while saving teams hours each week.
3. Predictive Analytics for Denial Management
Denial management traditionally relies on reactive processes. AI predictive analytics empowers healthcare providers to anticipate and prevent claim denials before they occur, optimizing financial performance.
How It Works:
Machine learning models analyze historical claims data, payer behaviors, and denial patterns to predict which claims are likely to be denied. This enables healthcare teams to proactively address potential issues by correcting or clarifying claims before they are submitted, reducing denials and speeding reimbursement.
Benefits:
- Proactive Management: Resolves potential issues early, ensuring smoother billing cycles.
- Enhanced Cash Flow: Speeds up payments by addressing problems upfront, boosting revenue stability.
Real Example:
Example – Jorie AI
A mid-sized hospital implemented Jorie AI's AI-driven predictive analytics solution to tackle their high denial rates. By analyzing historical claim data, Jorie AI's solution identified patterns and trends associated with denials. The hospital was able to preemptively address issues before claims were submitted, resulting in a 25% reduction in denial rates within six months. This not only improved their cash flow but also enhanced their overall operational efficiency.
4. Revenue Cycle Optimization
Inefficient revenue cycles negatively impact healthcare profitability. AI provides actionable insights to streamline and automate revenue cycle processes, significantly boosting operational efficiency.
How It Works:
AI analyzes billing and operational data across the entire revenue cycle to pinpoint inefficiencies and bottlenecks. It automates repetitive tasks like claims follow-ups, identifies patterns of unpaid claims, and recommends targeted improvements to optimize workflow, ultimately increasing revenue collection and operational effectiveness.
Benefits:
- Maximized Revenue: Better identification of claims likely to require follow-up reduces missed payments.
- Operational Efficiency: Frees billing teams to focus on complex or high-priority issues.
Real Example:
Example – AKASA:
Utilized AI to automate revenue cycle operations at health systems like Banner Health, achieving substantial cost reductions and enhancing revenue capture. Within six months, AKASA worked more than 23,000 claims for both their hospital and provider groups. It also played a role in improving days in accounts receivable (A/R) by 13%.
5. Compliance Monitoring and Audit Readiness
Maintaining regulatory compliance is critical but challenging due to constantly evolving healthcare guidelines. AI offers continuous, real-time compliance monitoring, ensuring accurate coding and reducing audit risk.
How It Works:
AI continuously audits coding practices by comparing submitted codes against current regulatory guidelines and payer rules. It automatically flags potential compliance risks or discrepancies, enabling coding teams to quickly address any issues and stay consistently aligned with regulations.
Benefits:
- Reduced Audit Risk: Proactively maintains coding accuracy and compliance with regulatory standards.
- Avoidance of Penalties: Protects healthcare providers from costly fines and legal exposure.
Real Example:
Case Study – Inovaare’s Universe Management System (UMS):
The provider adopted Inovaare’s UMS, an AI-powered platform designed to automate regulatory reporting and ensure data accuracy. Achieved a 100% success rate in passing CMS program audits on the first submission. Reduced audit review time by nearly 90% and decreased the cost of audit follow-ups by 67%. Minimized subjectivity in data validation by 88%, leading to more consistent and reliable audit outcomes.
6. Fraud Detection
Healthcare fraud poses a significant financial threat. AI-based fraud detection swiftly identifies suspicious activities, safeguarding healthcare providers from costly financial losses.
How It Works:
AI algorithms analyze vast sets of claims data to identify irregular billing patterns, duplicate submissions, unusual diagnosis-treatment combinations, or other signs indicative of potential fraud. By quickly flagging suspicious activity, healthcare organizations can swiftly intervene to prevent financial loss.
Benefits:
- Revenue Integrity: Protects healthcare organizations from financial losses due to fraud.
- Regulatory Compliance: Supports adherence to federal anti-fraud regulations and guidelines.
Real Example:
Example – Humana:
Humana implemented machine learning algorithms to analyze vast amounts of claims data, identifying discrepancies and irregular billing patterns indicative of potential fraud. In the first year of implementation, Humana's AI system helped eliminate potential fraudulent actions worth over $10 million.
7. AI-powered Virtual Assistants and Chatbots
Patient inquiries regarding billing and administrative matters often overwhelm healthcare providers. AI-driven virtual assistants and chatbots efficiently manage these communications, reducing administrative burdens and enhancing patient satisfaction.
How It Works:
Intelligent chatbots use AI technology to handle routine patient interactions, such as answering billing questions, clarifying insurance coverage, assisting with payment plans, or managing appointment scheduling. By addressing common patient queries instantly and accurately, these virtual assistants alleviate the workload of administrative staff.
Benefits:
- Enhanced Patient Experience: Immediate, 24/7 support for billing questions reduces patient frustration.
- Reduced Administrative Burden: Less manual workload allows for more efficient resource allocation.
Real Example:
Case Study – BotsCrew Chatbot for a Genetic Testing company
The companies challenges were:
- 25,000 - 30,000 calls per mont
- No 24/7 support, only Mon-Fri, 9am - 5p
- Customers waiting for days to receive their replie
- The company grows 25-30% annually, so does the number of CS request
- ~35%of calls volume were not handled annually
The client and BotsCrew started working on improving the company's customer experience by introducing the website & voice chatbot. The chatbot's goal was to provide patients with immediate answers to questions about their test orders & payments. In just a few month the chatbot conversed with 125,300 users, automated 25% of conversations, and helped to save $131,140/annually.
Want to Enhance Patient Support Without Overwhelming Your Staff? Discover how our AI-powered healthcare chatbots reduce call volumes, automate billing inquiries, and improve patient satisfaction—24/7.
Adopting AI-driven medical billing and coding solutions offers healthcare organizations enhanced efficiency, reduced costs, and improved revenue performance. Real-world examples from organizations like Geisinger Health, Olive AI, and Banner Health illustrate how AI positively impacts operational and financial outcomes, positioning healthcare providers for sustained growth and compliance excellence.
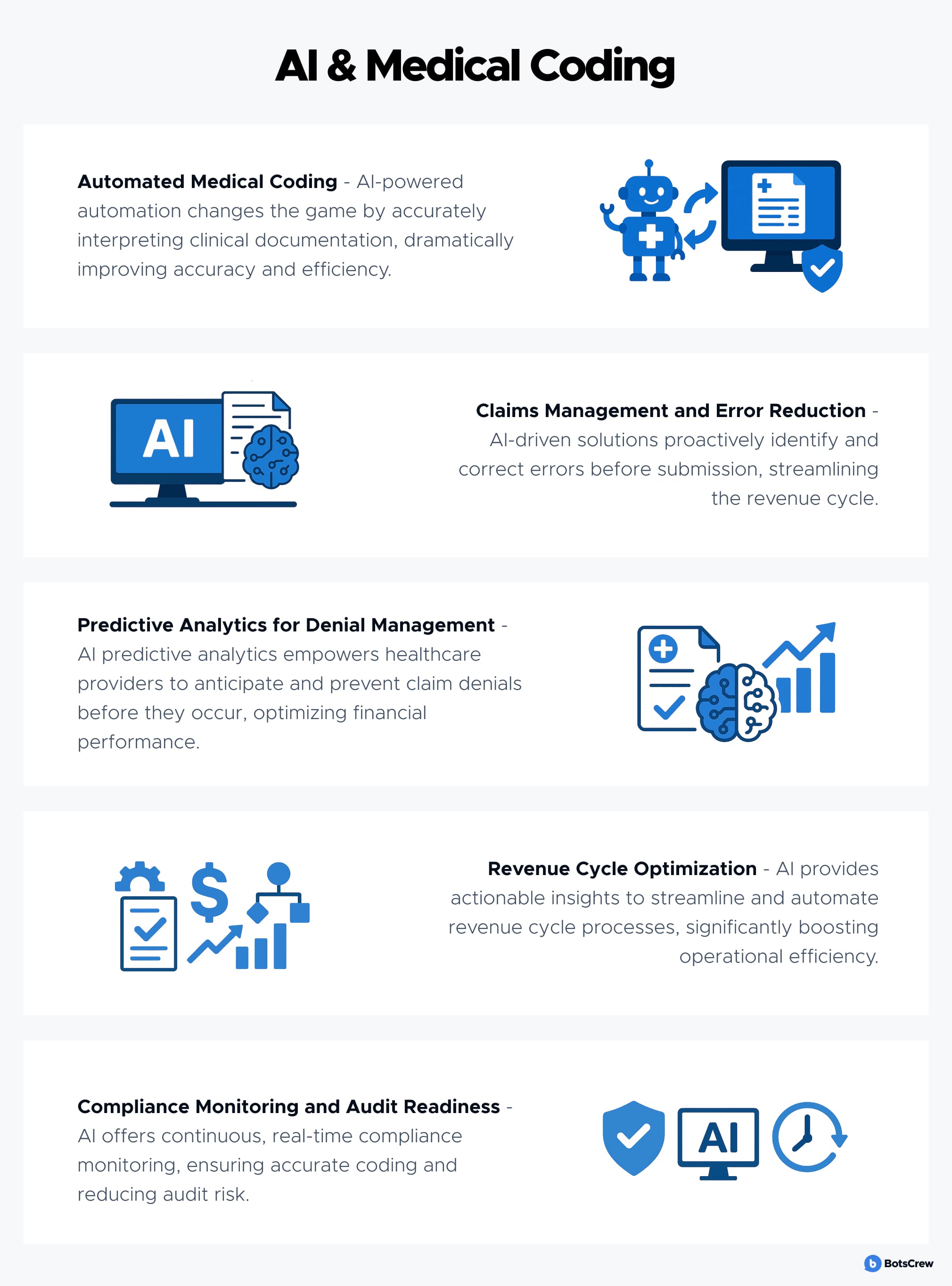
Challenges and Limitations of AI in Medical Billing and Coding
While AI offers tremendous potential, it's important to recognize its current challenges and limitations in the billing and coding landscape:
Data Quality Dependence: AI systems require high-quality, structured, and comprehensive data to function effectively. Inaccurate or incomplete clinical documentation can lead to incorrect code suggestions and billing errors.
Complexity of Medical Language: Human medical professionals often use nuanced or ambiguous language. Even the best NLP models can misinterpret context, leading to inaccurate coding if not supervised by experienced professionals.
Integration Difficulties: Implementing AI into existing EHR or billing systems can be complex, requiring careful planning, customization, and sometimes significant IT investment.
Regulatory Compliance Risks: Healthcare organizations must ensure that AI solutions are HIPAA-compliant and that any automated process still adheres to payer-specific documentation and coding requirements. Missteps can expose providers to audits, fines, or payment clawbacks.
Human Oversight Needed: Despite advances, AI is not infallible. It must be overseen by qualified coding and billing specialists who can validate outputs, correct errors, and ensure compliance with evolving regulations.
Understanding these limitations highlights why a thoughtful, phased approach to AI adoption—with strong human-in-the-loop oversight—is essential for success.
Tips for Implementing AI in Billing and Coding
1. Start Small and Focused: Begin with a pilot program targeting a single specialty, department, or task such as automating eligibility verification or claims scrubbing. Monitor outcomes closely, gather feedback, and demonstrate early successes before scaling across the organization.
2. Ensure Data Quality and Accessibility: Prepare your data by cleaning, structuring, and standardizing patient records and billing data. Good data hygiene ensures the AI model can learn and operate effectively. Collaborate with your IT team to ensure seamless data flow between AI systems and EHRs.
3. Invest in Staff Training and Change Management: Engage billing and coding teams early. Provide comprehensive training on how AI tools work, what tasks they will support, and how coders' roles will evolve. Position AI as a partner that reduces manual burden rather than a replacement, building trust and driving adoption.
4. Integrate with Existing Systems Thoughtfully: Choose AI solutions that can integrate smoothly into your current workflows and tech stack. Avoid siloed implementations; instead, embed AI within familiar systems like EHRs and billing platforms to reduce friction and speed adoption.
5. Maintain Rigorous Human Oversight: Establish processes where human experts validate AI outputs, especially during the early stages. Regular audits and random sampling of AI-generated codes or claims can ensure quality and regulatory compliance, while providing feedback loops to improve the AI system.
6. Prioritize Compliance and Security: Work only with HIPAA-compliant AI vendors and solutions that offer secure data handling. Ensure AI-driven processes meet payer and governmental documentation standards. Document AI workflows carefully to be prepared for potential audits.
7. Measure and Optimize Continuously: Define clear KPIs from the start—such as reduction in claim denials, turnaround time improvement, or coder productivity increases. Track results, identify bottlenecks, and iteratively fine-tune your AI deployment to maximize ROI.
By following these tips, healthcare organizations can ensure a smooth, successful transition to AI-enhanced billing and coding operations, minimizing risks while maximizing the value AI can deliver.
Why BotsCrew
At BotsCrew, we build custom AI solutions tailored to your healthcare workflows. From automating coding with NLP to deploying patient-facing billing chatbots, our HIPAA-compliant tools integrate seamlessly with your existing systems.
Unlike off-the-shelf platforms, BotsCrew solutions are built around your processes—resulting in faster implementation, better ROI, and long-term scalability. Whether you're looking to reduce claim errors or transform the entire revenue cycle, BotsCrew can help.
Ready to Cut Costs and Improve Billing Accuracy with AI? Let’s talk about how custom AI solutions can streamline your revenue cycle and reduce claim denials.
👉 Schedule a free consultation with BotsCrew’s healthcare AI experts today.