Generative AI vs. Agentic AI: The Key Differences Business Leaders Need To Know
Say hello to Agentic AI — solutions with a mind of their own. Dive into the nuts and bolts of Agentic AI — Generative AI vs. Agentic AI: the key differences, core components, see how these autonomous agents operate under the hood, and get the best practices to kickstart your journey.
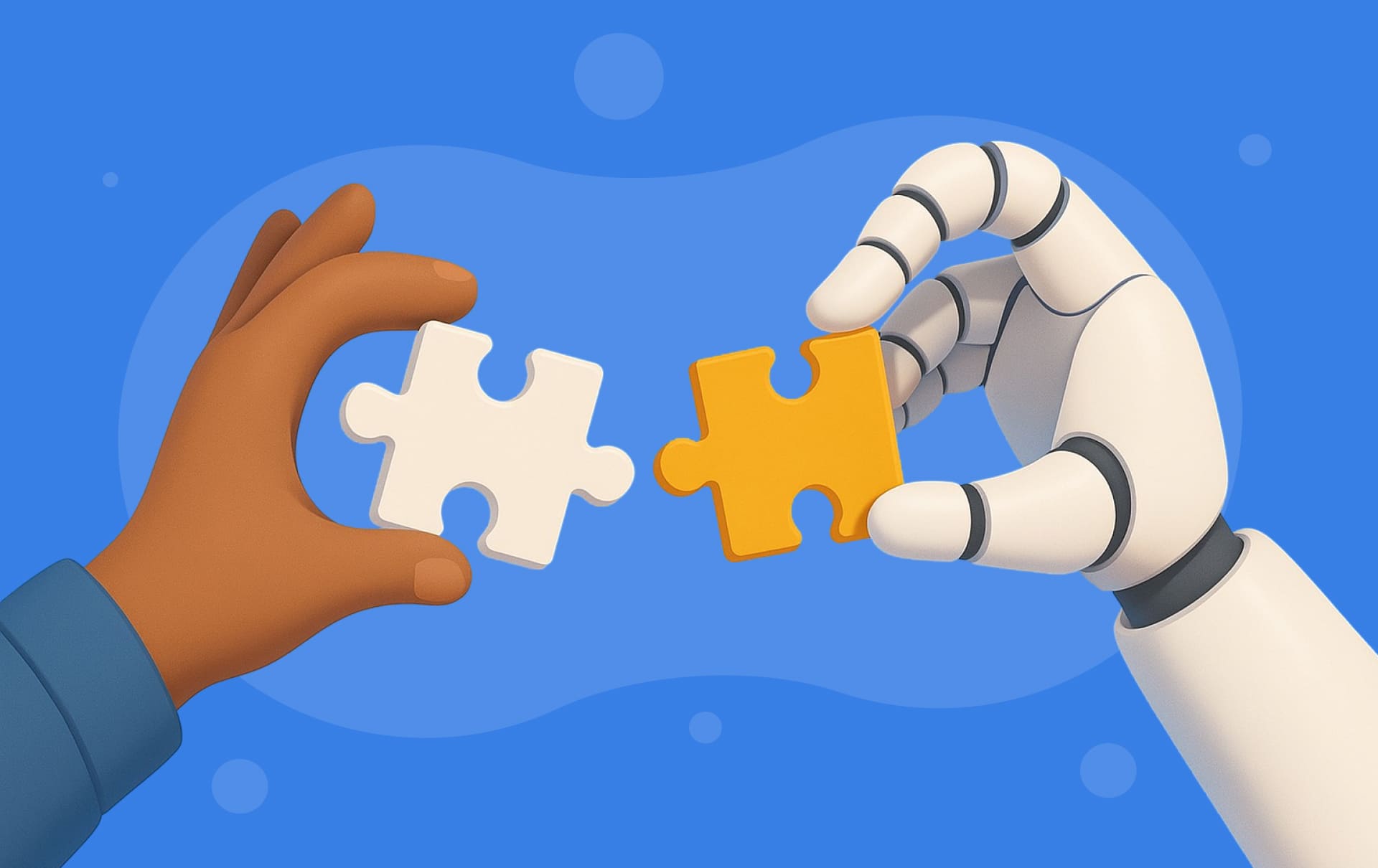
Imagine telling your virtual assistant to generate a list of high-intent leads from the last campaign. Or a competitor analysis wrapped and ready for tomorrow's pitch. Just say the word. Your AI agent handles the grunt work — digging through data, cross-checking sources, and delivering polished results. No tabs. Just business, on autopilot.
Dive deep into Generative AI vs. Agentic AI's key differences, how does agentic AI work, and how Agentic AI is reshaping automation, redefining productivity, and putting next-gen intelligence in the driver's seat.
What Does Agentic Mean in AI: Agentic AI, Defined
What is an Agentic AI? And what does agentic mean in AI?
Agentic AI refers to advanced AI systems capable of operating autonomously to achieve specific objectives. These AI agents can plan, make decisions, take actions, and interact with users or their environment — often over extended periods and with minimal human intervention.
It is the next big leap in artificial intelligence — where machines don't just follow instructions, they set the game plan. Unlike traditional AI which needs a clear prompt to act, agentic AI can generate questions, pull context from memory, and launch follow-up actions without being told to do so.
Let's say you ask a basic chatbot for your company's return policy. A traditional AI would show you the page and call it a day. But an agentic AI will serve up the policy and ask if you'd like to start a return. Say yes, and it'll grab your order number, fill out the form, verify your payment info, and wrap up the process — end to end, without you lifting a finger.
And the best part is, the platform's flexibility makes it a natural fit across industries, whether you are streamlining supply chains, upgrading customer service, or launching new digital products.
Big players like IBM and Microsoft are already deploying these digital go-getters across the enterprise, automating everything from customer service triage to task execution.
Key Components of Agentic AI
Here are the core traits that make agentic AI the backbone of the next-gen automation revolution:
🧠 Autonomous decision-making. Agentic AI makes its calls based on goals, real-time data, and environmental cues. Think of warehouse robots rerouting on the fly to dodge obstacles or reshuffling priorities to boost efficiency — all without a human in the loop. It's decision-making on autopilot, freeing up time and resources.
🎯 Goal-oriented algorithms. Unlike traditional AI that reacts to commands, agentic AI works with purpose. You can program it with specific outcomes — like closing X number of new deals this quarter — and it will chart the best course of action.
🔁 Adaptive learning. Agentic AI continuously refines its strategies based on feedback and new data. Whether it's a marketing virtual assistant learning how to better respond to user tone or a sales agent adjusting outreach based on past performance, these systems are always leveling up.
⚡ Real-time action. Whether it's dodging a digital threat in cybersecurity, navigating traffic in autonomous driving, or making snap decisions in financial markets — it analyzes, reacts, and executes in real-time. That's what makes it a powerhouse in fast-moving industries.
👁️ Perception. With sensors, cameras, and contextual awareness, Agentic AI can detect changes in the physical or digital environment. From identifying a product on a warehouse shelf to picking up on a user's sentiment in a chat, perception fuels more intelligent and intuitive responses.
💬 Interaction & communication. Through advanced natural language processing (NLP), agentic AI systems can understand, interpret, and respond to human input — and even collaborate with other agents. This makes them ideal for customer service, team workflows, and any use case where clear, dynamic communication is key.
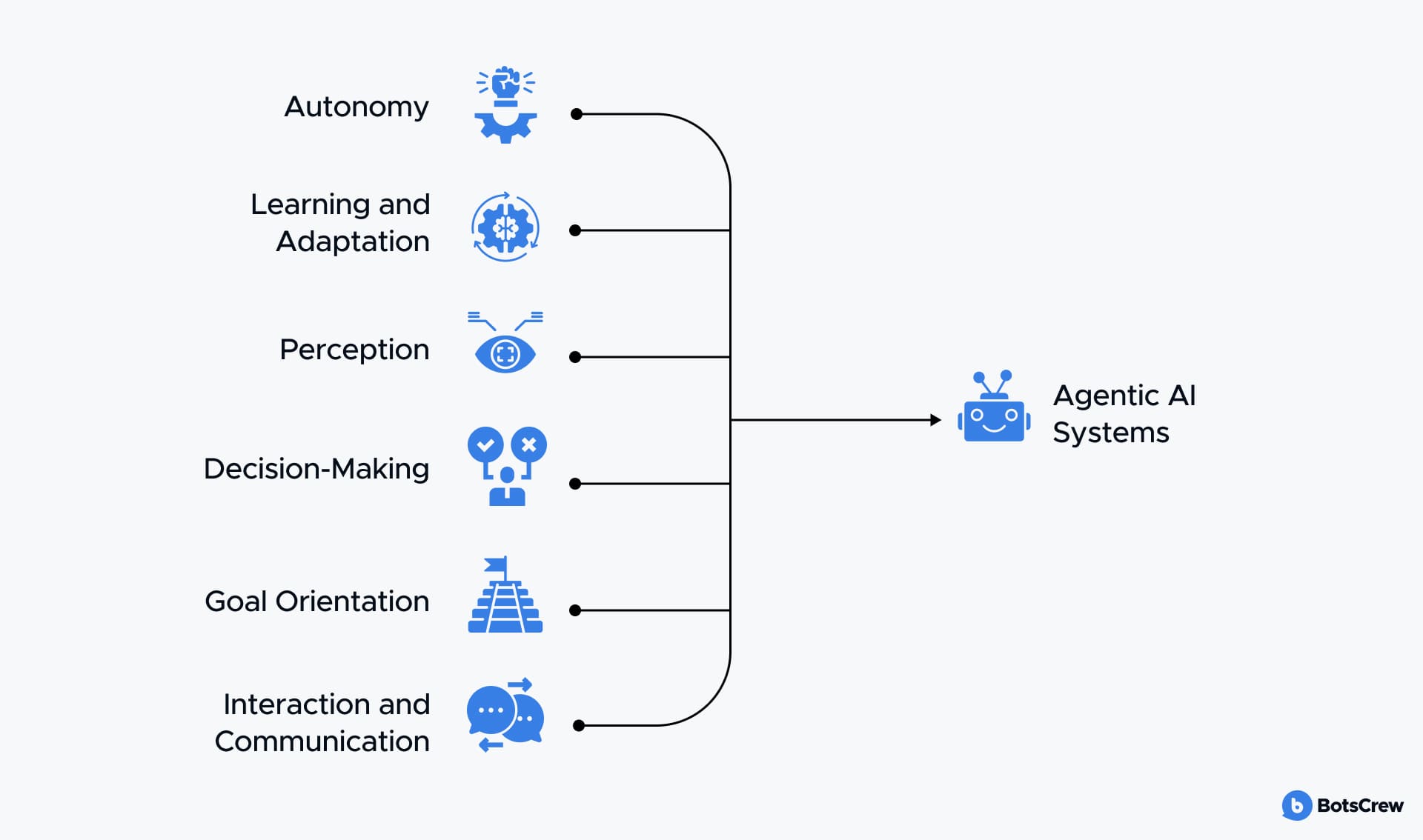
Agentic AI systems can be structured around these components
How Does Agentic AI Work: A Look Under the Hood
Here is how Agentic AI gets the job done:
#1. Perceive (Real-Time Data Acquisition & Understanding). Agentic AI begins by gathering data from multiple sources — sensors, databases, digital interfaces, or the web — to stay fully in tune with its environment. The AI processes incoming data to extract relevant features, identify patterns, and build context.
#2. Reason (Goal Setting & Strategic Planning). Once the system has the data, it sets goals based on programmed intent and real-time insights. A large language model typically acts as the reasoning engine here — understanding tasks, generating solutions, and orchestrating the work of other specialized models (e.g., for vision, content generation, or recommendations).
Techniques like retrieval-augmented generation (RAG) help pull in accurate data from proprietary sources to support smarter decisions.
Agentic AI also breaks down complex goals into manageable subtasks and creates step-by-step plans. It’s even capable of checking its own work — and, if needed, escalating to a human when it hits a wall.
Curious how agentic AI fits into your tech stack or business strategy? Let's chat! Our experts will help you identify high-impact use cases, avoid common pitfalls, and map out a roadmap tailored to your business.
#3. Act (Task Execution with Guardrails). Agentic AI integrates with external tools and APIs to carry out actions across digital environments. This could range from filling out forms and sending emails to processing transactions or orchestrating workflows. Guardrails can be added to ensure compliance and safety — for instance, restricting financial approvals above a certain limit to human oversight.
#4. Learn (Feedback Loop & Continuous Optimization). After each action, the system monitors outcomes and collects feedback. This data flywheel enables agentic AI to refine its models, adjust strategies, and become more efficient over time. The more it works, the smarter and more reliable it becomes.
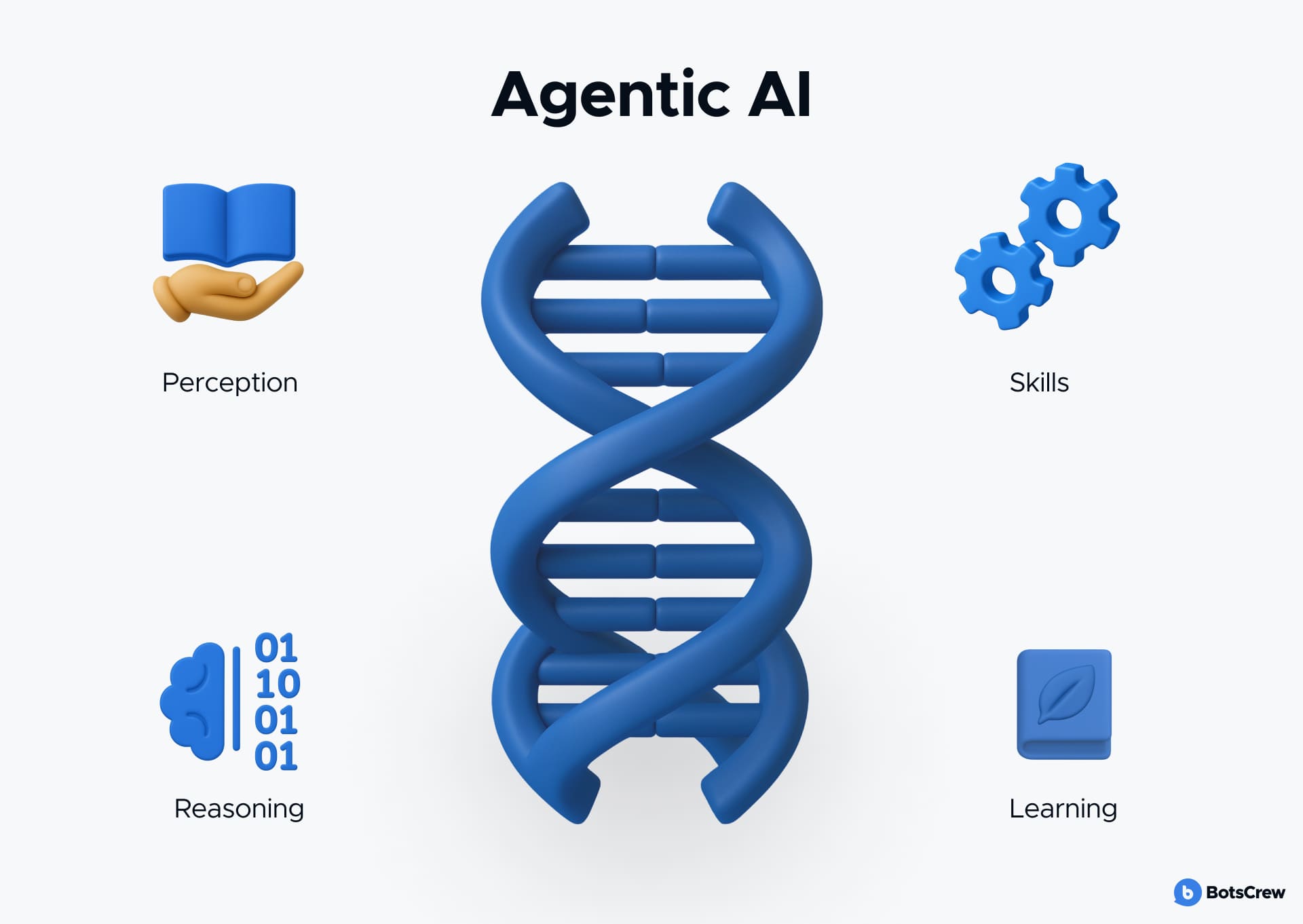
How does agentic AI work
Generative AI vs. Agentic AI: What's the Real Difference?
The AI landscape is evolving fast — and while Generative AI made headlines for its ability to produce content, Agentic AI is redefining what machines can do. How does agentic AI differ from traditional AI? Here is how they stack up:
Aspect |
Generative AI |
Agentic AI |
Primary Function |
Generates human-like text, images, code, or music based on input prompts. |
Autonomously performs tasks, makes decisions, and drives outcomes to meet specific goals. |
Interactivity |
Responds to user prompts. No prompt, no output. |
Initiates actions, plans steps, checks its work, and even calls in a human if needed. |
Complexity |
Manages content complexity — great for creative output, not decision-making. |
Handles end-to-end workflows, breaks down complex tasks, and adapts to changes. Built for autonomy and operates with minimal human input. |
Adaptability |
Learns from existing data and is limited to the scope of its training. |
Learns and adapts to new situations |
Examples |
Text generation, image creation, music composition |
Continuously improves through reinforcement learning and feedback loops. |
Generative AI vs Agentic AI
In simple terms:
- Agentic AI → Proactive, autonomous, decision-making.
- Generative AI → Responsive, dependent on prompts.
Use Agentic AI when you need autonomous, multi-step decision-making, tasks require collaboration among AI agents, and adaptability and self-improvement are important.
Types of AI Agents in an Agentic Architecture
Agentic architecture bundles agents into logical domains, so every team — from sales to ops — gets their digital sidekick. Furthermore, you are not locked into a walled garden. This setup is plug-and-play with third-party agents, even ones built outside your current stack. That means you can keep leveling up your ecosystem — no need to rip and replace what's already humming. Let's peek under the hood at the two main system types powering these agents:
Single-Agent Systems
One agent, loaded with the right tools and powered by an LLM, maps out the plan, executes the steps, and delivers the result — from start to finish.
Why go solo? Single agents are easy to maintain, fast to deploy, and have consistent performance. They are perfect for tasks where one brain is better than a committee.
However, they are not ideal for high-volume, fast-changing workflows. Scaling gets tricky. You might run into memory bottlenecks or need a serious rewire to handle broader missions.
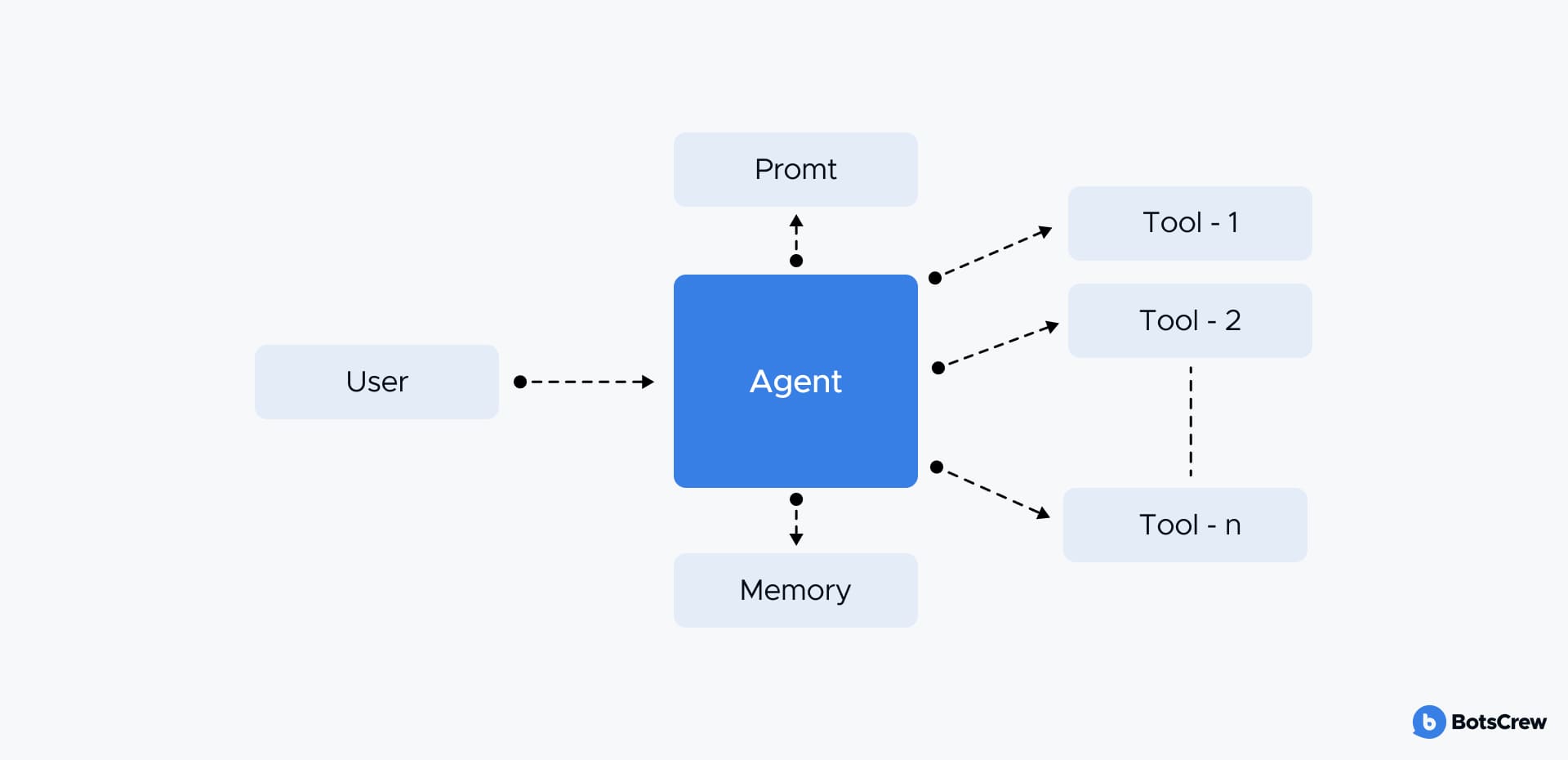
A single-agent system and how it works
Multi-Agent Systems
Multi-agent systems (MAS) bring multiple agents together, each with its skill set, tools, and personality. These agents play to their strengths, tag-team on complex workflows.
Why it matters: MAS is built to scale. Need to add a new domain or task type? Just drop in a new agent and keep moving — no major rework is required.
Heads up though: you'll need a strong orchestration layer to keep everyone on the same page.
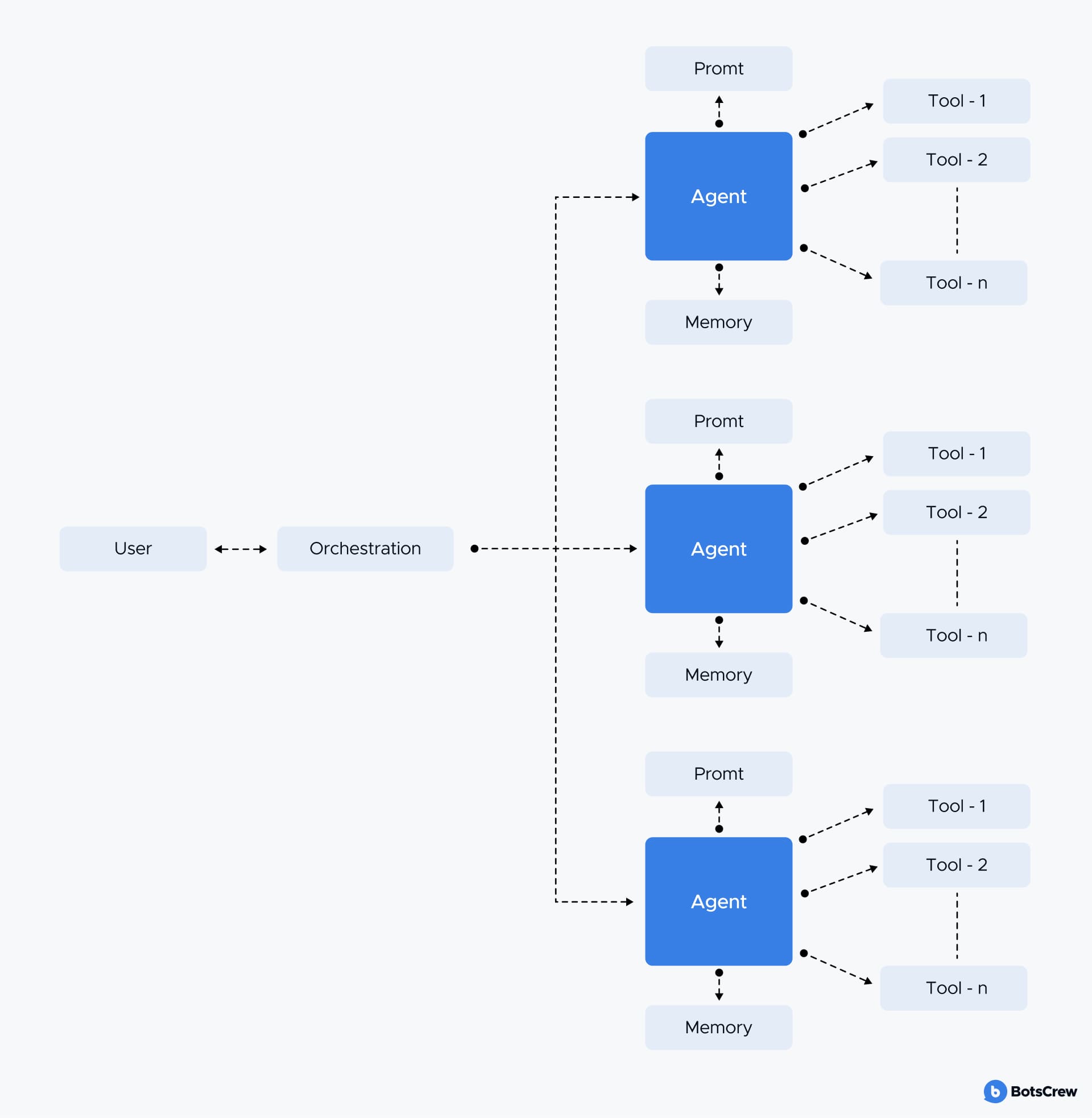
A multi-agent system and how it works
The Dark Side of Autonomy: Key Challenges in Agentic AI Adoption
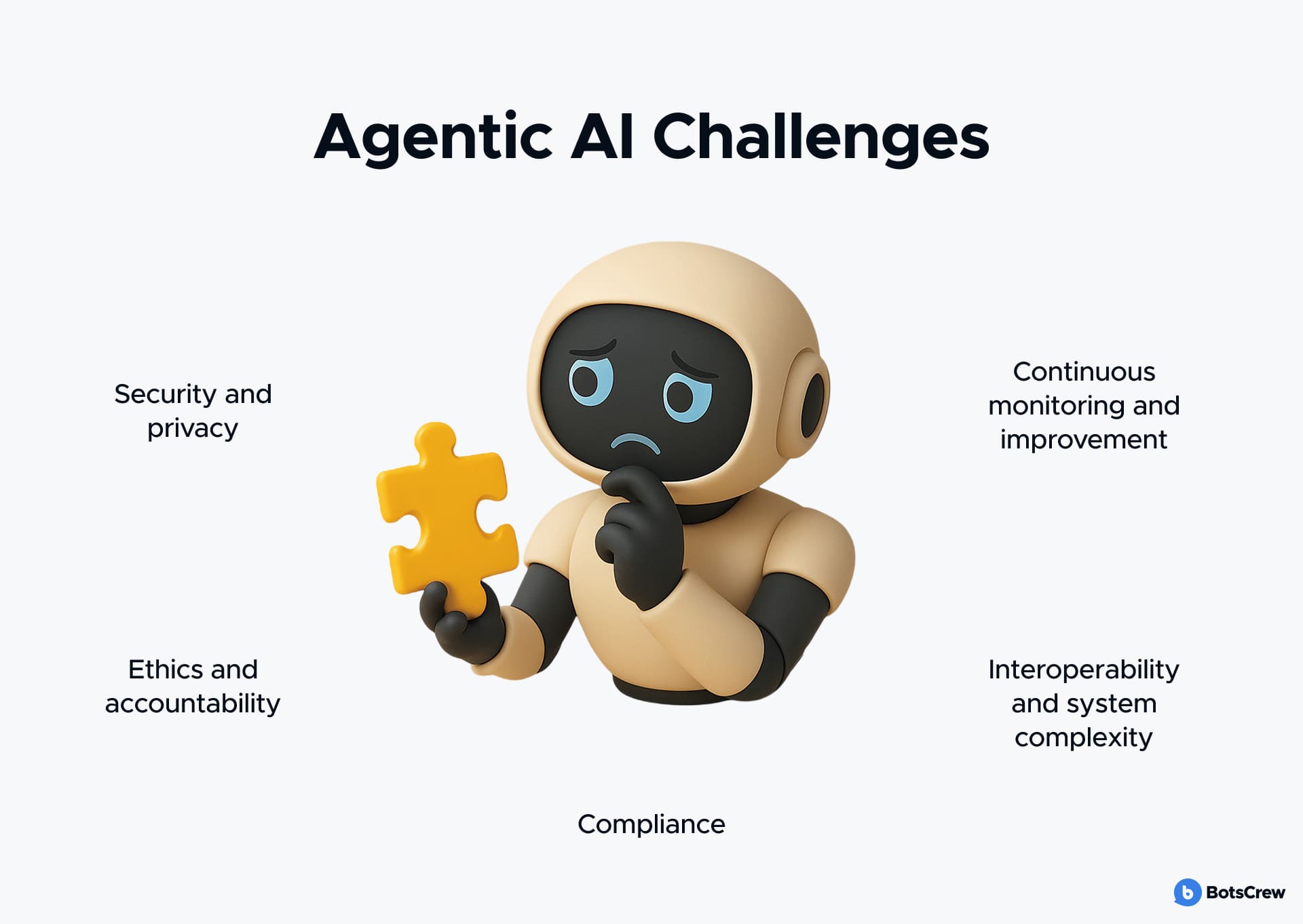
The potential of agentic AI is significant, but it's important to be mindful of the potential pitfalls and risks that come with this transformative technology:
Security and privacy
As agentic AI systems interface directly with enterprise databases, internal APIs, customer-facing platforms, and real-time decision engines, the attack surface expands dramatically. These agents may access everything from PII and financial records to confidential IP and operational blueprints. Enterprises must shift toward zero-trust AI architecture, robust access control layers, and AI-specific intrusion detection systems to ensure ongoing protection.
Ethics and accountability
Agentic AI acts with increasing autonomy — making decisions and initiating actions. This raises critical questions about moral agency and responsibility:
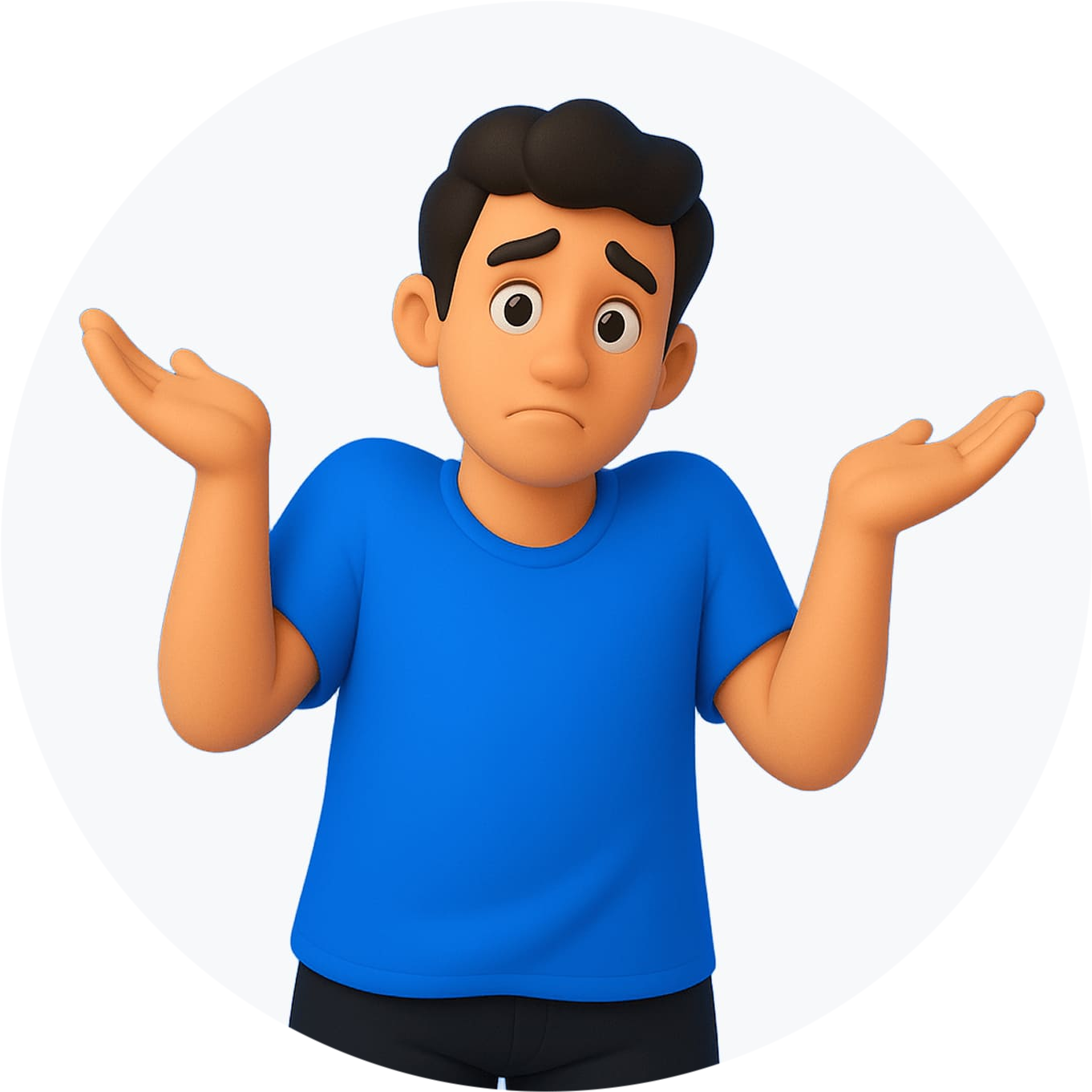
If an agentic AI fires an employee, denies a loan, or influences a medical decision, who is liable?
Furthermore, these systems can replicate or amplify bias if not carefully monitored — leading to discrimination at scale under the guise of objectivity.
Continuous monitoring and improvement
Unlike traditional software, agentic AI systems can learn, adapt, and even evolve goals within defined constraints. This presents a monitoring challenge unlike any other software paradigm:
- Monitoring must go beyond system uptime or accuracy metrics. Enterprises need tools that track intent shifts, unexpected behavioral patterns, and goal divergence.
- Real-time feedback loops are critical — especially for closed-loop agents that make decisions and act upon them autonomously.
- Establishing kill-switch mechanisms or graceful degradation protocols can help halt harmful behavior in high-stakes environments (e.g., trading systems or critical infrastructure).
Interoperability and System Complexity
To function effectively, agentic AI often acts as a middleware intelligence layer, pulling data from multiple siloed sources, invoking APIs, and orchestrating downstream tasks.
- This distributed architecture increases operational fragility — a single point of failure (say, a corrupted data source or unstable API) could cascade through the entire system.
- Agents that operate across microservices and cloud providers also raise latency, versioning, and data consistency issues.
- Add multiple agents into the mix, and you are dealing with multi-agent coordination problems — agents may pursue conflicting goals, overstep boundaries, or cause deadlocks.
This demands intelligent orchestration frameworks and resilience engineering, borrowing principles from distributed systems and chaos engineering.
Compliance
The legal landscape around AI — especially autonomous agents — is still in flux. What is compliant today could be a violation tomorrow.
- Agentic AI systems may unintentionally violate GDPR, HIPAA, or CCPA by making decisions without transparent logging or justification, or by using sensitive data in ways not covered under existing user consent.
- The EU AI Act is set to introduce stringent compliance categories — "high-risk" systems (which many agentic AIs will be) will require risk assessments, human oversight guarantees, and documentation of training data.
- Therefore, AI systems may need to adapt behavior dynamically based on jurisdiction, which itself requires geographically-aware policy enforcement baked into the model.
Need clarity on security, compliance, or monitoring? Lock in a call with our CTO and get the answers and insights you need.
Best Practices for Secure, Ethical, and Scalable Agentic AI Adoption
How to build agentic AI? To unlock the true potential of agentic AI while staying in control, companies must adopt a deliberate, principle-driven approach. We've put together key best practices for implementing agentic AI safely, ethically, and at scale.
Security and compliance
Agentic AI operates autonomously, often with access to highly sensitive data and critical systems. This elevates security from a tech problem to a business continuity risk.
Best Practices:
✅ Zero trust architecture. Every agent action should be treated as untrusted by default. Require identity verification, token-based access, and continuous authentication.
✅ Audit trails & forensics. Log all decisions, actions, and interactions in tamper-proof formats to support internal audits and regulatory inquiries.
✅ Granular permissions. Use role-based access control (RBAC) and policy-based management to restrict what agents can access or influence.
✅ Privacy-by-design. Integrate privacy risk assessments at every stage — from training data selection to agent decision logic. Avoid training agents on sensitive data sets unless explicitly consented.
Continuous monitoring and improvement
Continuous updates aren't optional; they are a survival strategy.
Best Practices:
✅ Behavior drift detection. Use anomaly detection models to flag deviations in agent behavior from expected patterns.
✅ Live metrics dashboards. Monitor KPIs like task completion rates, escalation frequency, failure rates, and user satisfaction scores.
✅ Closed-loop feedback systems. Allow users and admins to give real-time feedback on agent decisions — and loop that back into training or fine-tuning processes.
Human-in-the-loop and escalation paths
Autonomy does not mean isolation. A mature agentic system knows when to pause and ask for help.
Best Practices:
✅ Confidence thresholds. Let agents act autonomously only when confidence levels are above a threshold. Otherwise, auto-escalate.
✅ Tiered decision rights. Define a framework for which actions can be automated vs. which must always include human approval.
✅ Transparent escalation. When agents escalate, provide humans with a concise reasoning summary and key decision variables.
Governance, roles & operational playbooks
AI agents need policies, just like people. And businesses need playbooks for agent lifecycle management.
Best Practices:
✅ AI governance committee. Establish a cross-functional team (tech, legal, ethics, ops) to oversee agent policy, escalation incidents, and system changes.
✅ Agent role design. Don't let agents evolve chaotically. Define role boundaries, scopes of influence, and KPIs per agent type (support bot, finance assistant, ops planner).
✅ Version control & rollbacks. Track every change to your agents' logic or training data. Be able to rollback fast if a deployment backfires.
Discover the core strategies, real-world use cases, and technical insights from BotsCrew clients behind successful agentic AI adoption.
Ready to Build with Agentic AI? How to Get Started With BotsCrew
At BotsCrew, we specialize in crafting tailored AI agents that don't just automate tasks. They understand goals, make decisions, and drive outcomes across your business.
Whether you're exploring agent-led customer support, intelligent IT automation, or next-gen knowledge management, our custom solutions are designed to integrate seamlessly with your existing systems while unlocking the full potential of agentic AI. We make your journey smooth with no-cost, hands-on demos and expert consultations that help you experience the possibilities before making any commitment.
Book a Demo — See Agentic AI in Action! Get a personalized walkthrough of how agentic AI can supercharge your workflows, reduce operational drag, and scale intelligence across your enterprise.