From Copilots to Agents: What Are AI Agents & How to Build and Benefit from Them
Gen AI steadily evolves from supportive tools into fully autonomous agents capable of independent decision-making and execution. Discover what are AI agents, AI agents' use cases, the transformative potential of Generative AI agents, how to build an AI agent, and how you can use technology to streamline workflows and drive innovations and higher profits.
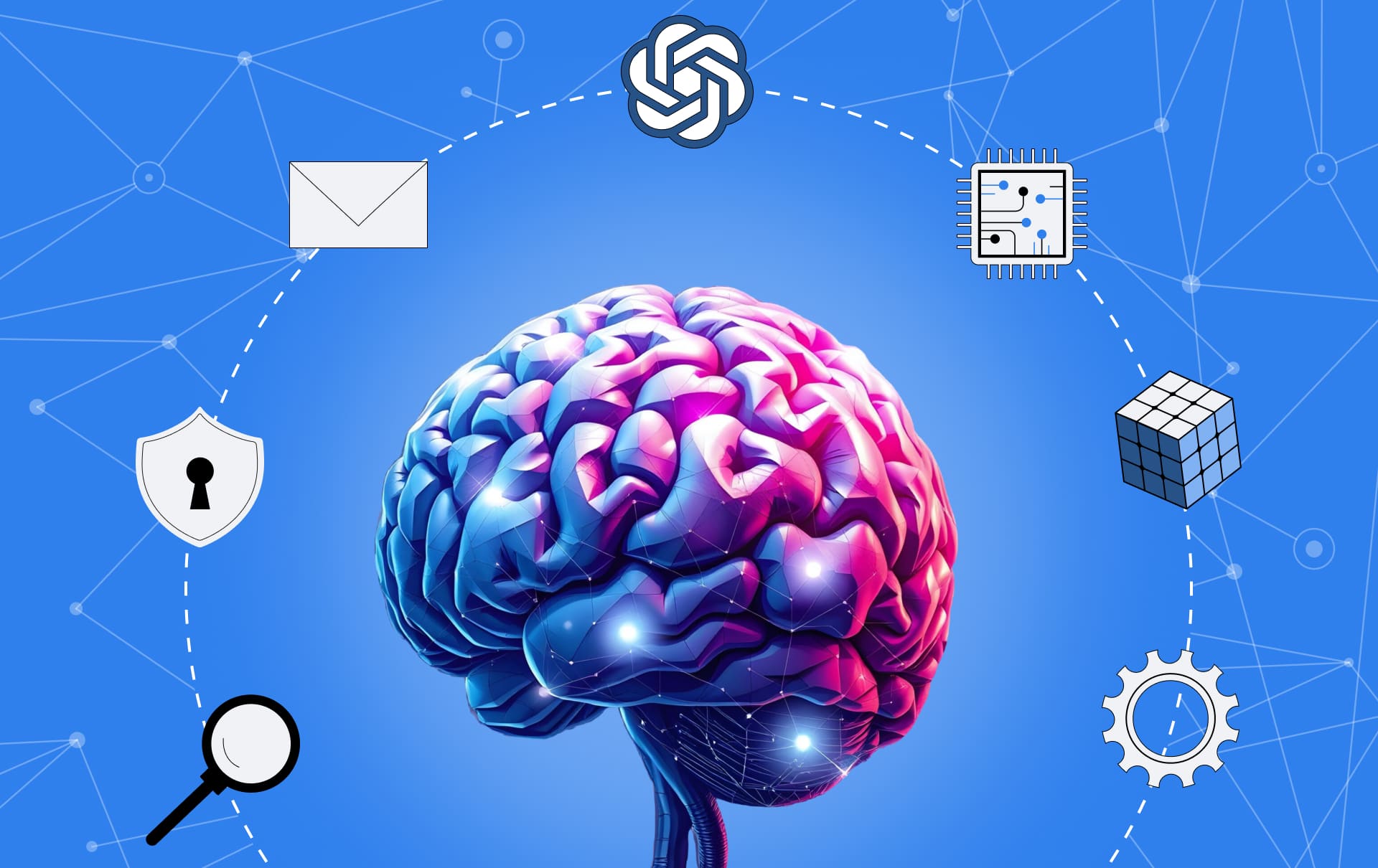
Generative AI agents go beyond automation — they can recall past experiences, infer insights about themselves and others, and craft strategies based on their input and surroundings. These intelligent systems are not just reactive but strategic, paving the way for smarter, more efficient workflows. They act as partners in decision-making, delivering insights that are revolutionizing industries and transforming customer experiences.
This article dives deep into what are AI agents and types of AI agents, cutting through the buzzwords to reveal how they can revolutionize your business.
Introduction to Generative AI Agents
AI agents are intelligent systems that can independently decide how to tackle tasks without constant guidance. They are designed to interact with their environment, gather data, and autonomously perform tasks to achieve set goals.
These agents "sense" their environment, whether through physical sensors (like in robotics) or digital inputs (like customer queries for chatbots). Over time, they learn from their task history, human feedback, and other inputs, continuously improving performance and adapting to changes in their environment.
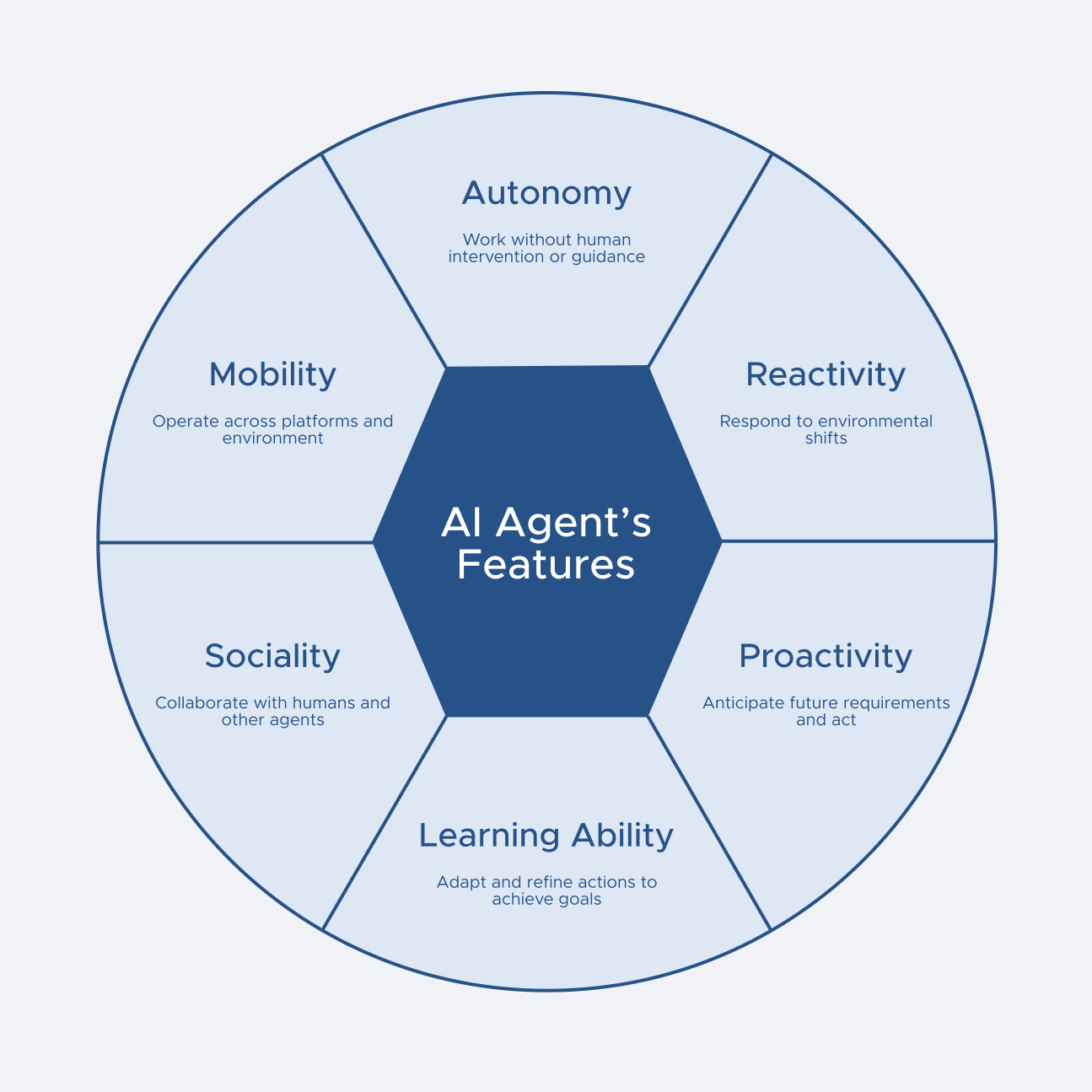
Imagine giving your AI agents a more ambitious project, like launching a glamping house business in a sought-after region of the U.S. The AI business analyst agent jumps into action, studying the market, identifying trends, and crafting a winning business model. It then calls on the AI marketing agent, who fine-tunes the strategy, determining the best marketing platforms, messaging, and targeting.
Lastly, the AI developer agent builds and tests the website — all without human input. This dynamic, fully autonomous team of agents works seamlessly together, delivering a ready-to-go business with no need for constant oversight. This is the cutting-edge AI solution.
In contrast, a chatbot operates on a simpler level. It follows set instructions and completes tasks as programmed, but it lacks the ability to independently verify or refine its actions.
Dr. Andrew Ng, one of the foremost leaders in AI, founder of DeepLearning.AI, Landing AI, and an Adjunct Professor at Stanford University’s Computer Science Department, emphasized that AI agents are transitioning from being mere assistants to fully autonomous entities capable of handling complex tasks independently. Ng highlighted that upcoming AI models will feature enhanced planning and reasoning capabilities. While current LLMs)are adept at replicating patterns from vast datasets, they still fall short in deep logical reasoning. To bridge this gap, researchers are developing new techniques such as allowing models more time to "think" before decision-making through inference stages and using gameplay-like value iteration to simulate deeper reasoning. These innovations could significantly boost AI’s cognitive abilities, enabling them to tackle more advanced tasks that require higher levels of thought, strategy, and planning.
However, generative AI agents' complexity can range from simple systems that carry out predefined tasks in controlled environments to advanced AI systems that learn, adapt, and thrive in complex, ever-changing situations.
A recent survey by Capgemini revealed that 82% of 1,100 tech executives plan to integrate AI agents into their organizations within the next three years. The findings showed that 70% of respondents would trust AI agents to analyze and synthesize data, while 50% are confident in letting AI agents send professional emails on their behalf. Additionally, around 75% said they aim to use AI agents for tasks like generating and refining code.
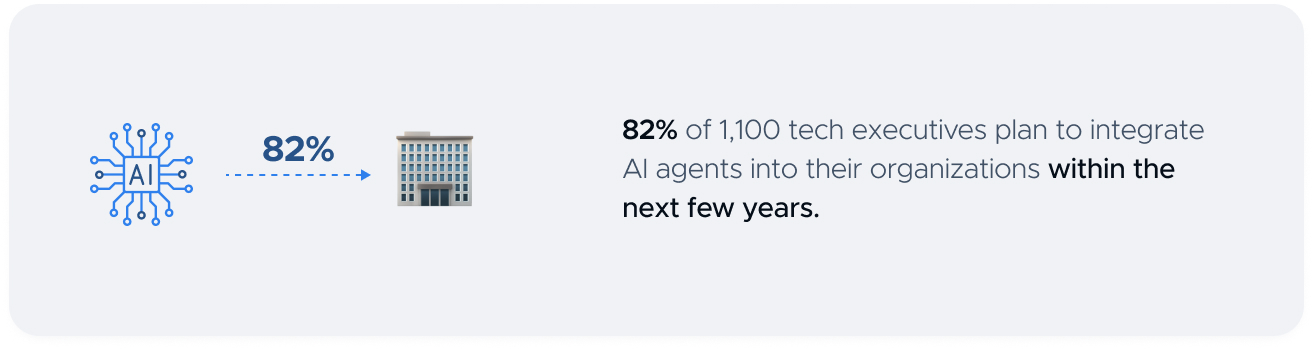
How Does a Gen AI Agent Work: A Detailed Perspective
A Gen AI agent's workflow is a finely tuned machine, blending data analysis, decision-making, and continuous learning in a complex, ever-evolving process. By understanding how these AI agents function, businesses can better define objectives, allocate resources efficiently, and make sense of the results, whether in areas like customer service, supply chain management, or strategic planning. Let's dive into the intricacies of how an AI agent operates:
Goal Determination
The AI agent starts by receiving a clear instruction or goal from the user. With this in hand, it maps out a plan of action designed to produce results that are not only relevant but highly useful.
For instance, you need to create an email marketing campaign for Black Friday. The AI agent understands this goal and aims to maximize engagement and conversion.
Task List Creation
Once the goal is set, the Gen AI agent crafts a detailed game plan. Whether it is crunching market trends or automating customer support, the agent takes that broad objective and breaks it down into bite-sized, actionable tasks. It prioritizes the tasks, organizes them in the best order for execution, and prepares for any curveballs that might come its way.
For the Black Friday campaign, the agent breaks it down into:
- Analyzing the historical performance of previous Black Friday campaigns.
- Identifying top-performing campaigns and understanding why they succeeded.
- Using insights to draft a high-impact email template.
- Determining the audience segments most likely to engage with the campaign.
- Curating a targeted contact list for the email blast.
Data Gathering & Analysis
The agent gathers the necessary data to complete the tasks. It may pull information from previous campaigns, external databases, or relevant industry trends. In doing so, the AI ensures that all actions are data-driven and highly customized to the user’s needs.
The AI collects and analyzes past Black Friday campaign performance, such as open rates, conversion rates, and engagement metrics. It also examines trends in customer behavior during holiday sales.
Task Execution
With the task list and data ready, the AI agent begins executing each step. It may use a range of tools, from natural language processing for drafting content to analytics engines for sorting through data.
The AI agent drafts the email copy using the successful elements it identified from previous campaigns. It creates a personalized subject line optimized for higher open rates. Later, the agent designs the email layout, ensuring it's visually engaging and on-brand. To complete the process, the agent also sets up email automation by determining the best time to send emails, defining audience triggers, and ensuring follow-ups are delivered automatically. This allows the campaign to run efficiently without human intervention.
Multi-Agent Collaboration
For more complex tasks, the Gen AI agent can call upon other agents or sub-agents specialized in different areas, such as copywriting, data analysis, or design. Each agent works independently on its subtask but collaborates toward the final goal.
- One AI agent focuses on writing the email content.
- Another handles data analysis to refine the target audience.
- A third agent works on designing the email template and ensuring it is mobile-optimized.
Self-Reflection & Feedback Loop
The AI agent continuously monitors its progress, analyzing whether its actions align with the end goal. If an issue arises, the agent adjusts its plan or seeks feedback. It refines the output in real time, making iterations to ensure that the final product is as polished as possible.
If the initial email draft isn't engaging enough based on the AI's internal analysis or feedback, it adjusts the content or design until it reaches optimal performance levels.
Result Validation & Delivery
Before finalizing, the agent checks the output to ensure it meets the criteria and goal originally set by the user.
The agent may conduct tests, such as A/B testing the email subject lines or verifying the segmentation of the audience list. Later, the agent finalizes the email template and audience list, ensuring that all aspects align with the best practices identified in its data analysis. It then presents the complete campaign to the user for review.
Learning & Improvement
The AI agent isn't just running through tasks. It is learning from every step. By fine-tuning its approach based on past experiences, the agent evolves to handle even more complex functions in the future.
After the Black Friday campaign, the agent evaluates key performance indicators (KPIs) like open rates, click-through rates, and conversions, using this data to refine future campaigns.
6 Types of AI Agents to Supercharge Your Project and Streamline Business Operations
Custom AI development partners deploy various categories of intelligent agents, each suited to different tasks. We share some types of AI agents below.
#1. Simple Reflex Agents
Simple Reflex AI Agents react to specific triggers based on predefined rules, without needing to store past experiences or learn from them. These agents are programmed to follow a "condition–action" process, which means they respond to certain conditions or stimuli with a predetermined action.
Unlike more advanced AI agents, simple reflex agents do not store information about past interactions. They don't learn from their previous actions or adapt to new situations either. Simple reflex agents perform the task they are programmed to do when a specific task arises. Ideal for simple tasks like resetting passwords by recognizing keywords in conversations.
Industries: Customer Support, IT Services, E-commerce
Use Cases: Password resets, basic troubleshooting, responding to common inquiries (e.g., FAQs in chatbots), processing routine transactions.
Companies: IT service providers like Atlassian could use these agents to automate common helpdesk tasks.
E-commerce platforms like Amazon or Shopify might deploy them for handling frequent customer queries (order tracking, returns).
#2. Model-based Reflex Agents
Model-based reflex agents have the ability to store and reference past experiences as well as make smarter decisions over time. They don't just react to immediate triggers and act based on predefined rules — they also maintain an internal representation of the world around them.
This model keeps track of changes in the environment, allowing the agent to recognize what has happened before and avoid repeating mistakes or redundant actions. For example, if a robot vacuum has already cleaned a certain area, it remembers that and avoids cleaning the same spot again. As these agents can learn from past experiences, they are better suited to environments where conditions change frequently.
Industries: Home Automation, Manufacturing, Supply Chain, Smart Devices
Use Cases: Navigation for autonomous robots, optimizing warehouse operations, home devices like robot vacuums, tracking product placements.
Companies: iRobot (robotic vacuum cleaners) could utilize this agent type for memory-based navigation. Tesla or Waymo could implement model-based reflex agents in autonomous driving for route optimization. Amazon Robotics might use these agents in automated fulfillment centers to track items efficiently.
#3. Goal-based Agents
Goal-based agents focus on achieving a clearly defined goal, whether it's solving a problem or executing a task. They plan and adapt their actions to reach the target, constantly checking and evaluating multiple approaches before choosing the best one.
For instance, a robot might assess several paths to navigate around obstacles before selecting the shortest and safest route. Their ability to replan makes them highly efficient in dynamic environments.
Industries: Healthcare, Robotics, Customer Service, Logistics
Use Cases: Robotic surgeries, self-driving cars, supply chain management, personal assistants that need to plan meetings or arrange complex schedules, complex question-answering in chatbots.
Companies: NVIDIA or Boston Dynamics in robotics could use goal-based agents for automating precise operations like assembly-line robotics or medical procedures. OpenAI and Google might deploy these agents in natural language processing for applications like Google Assistant or ChatGPT for task-solving through user interaction.
#4. Utility-based Agents
Utility-based agents prioritize certain factors, such as user preferences, to make decisions that maximize satisfaction or efficiency. Unlike goal-based agents, which focus solely on reaching a goal, utility-based agents weigh trade-offs between multiple options to find the best overall outcome.
For instance, in travel booking, a utility-based agent might analyze factors like travel time, cost, and layovers to recommend flights. If a user values convenience over price, the agent will prioritize shorter flights even if they are more expensive.
These agents are widely used in recommendation systems, customer service, and personalized marketing, where decision-making involves multiple variables and user preferences play a significant role.
Industries: Travel & Hospitality, E-commerce, Financial Services, Retail
Use Cases: Travel planning (finding optimal flights), personalized shopping recommendations, financial investment advisors, or insurance recommendations based on client needs.
Companies: Expedia or Booking.com could use these agents to optimize travel recommendations based on user preferences. Amazon or eBay may implement utility-based agents to offer personalized product suggestions that prioritize customer satisfaction.
#5. Learning Agents
Learning agents are designed to improve their performance over time by continuously learning from their environment and experiences. Unlike other types of agents that rely on predefined rules or models, learning agents adapt through feedback loops, allowing them to evolve and make better decisions with each interaction.
For instance, a customer support chatbot might start with limited responses but, over time, learns from interactions with customers and improves its answers, becoming more accurate and helpful. Similarly, in autonomous driving, learning agents adapt to real-world road conditions by constantly processing new data from sensors and making adjustments in real time.
Therefore, learning agents are particularly valuable in dynamic and complex environments where conditions change frequently, such as financial trading, personalized recommendation systems, and autonomous robots.
Industries: Education, Finance, Healthcare, Gaming, Software Development
Use Cases: Personalized learning programs, autonomous trading systems in finance, medical diagnostic systems that improve over time, AI-powered video games that adapt to player behavior.
Companies: Duolingo could deploy these agents in personalized education tools that adapt to individual learning styles. JPMorgan or Goldman Sachs may use learning agents in automated trading algorithms for improving financial predictions and investment decisions. Epic Games could use learning agents in gaming to enhance NPC behavior that adapts to player actions in real-time.
#6. Hierarchical Agents
Hierarchical agents operate in a multi-tiered system, with higher-level agents managing complex tasks by delegating subtasks to specialized lower-level agents. This structure allows large problems to be broken down into smaller, manageable parts, ensuring efficient task completion.
Higher-level agents focus on broad objectives, distributing tasks to subagents responsible for specific areas. For instance, in supply chain management, a top-level agent oversees logistics, while subagents handle inventory, transportation, and supplier coordination.
Hierarchical agents are ideal for industries like manufacturing, robotics, large-scale operations, and even AI-driven project management systems, where multiple teams or systems need to collaborate efficiently to reach a common goal.
Industries: Enterprise Software, Manufacturing, Project Management, Defense
Use Cases: Large-scale project management (breaking down complex tasks into smaller ones), automated factory operations, handling complex supply chains, and coordinating multiple drones in defense or logistics.
Companies: SAP or Oracle could implement hierarchical agents to manage large-scale enterprise resource planning (ERP) systems. Boeing or Lockheed Martin may leverage hierarchical agents in defense for managing complex systems like drones or autonomous combat systems. Siemens and General Electric could deploy them in smart factories for managing automated production lines that require task delegation and coordination.
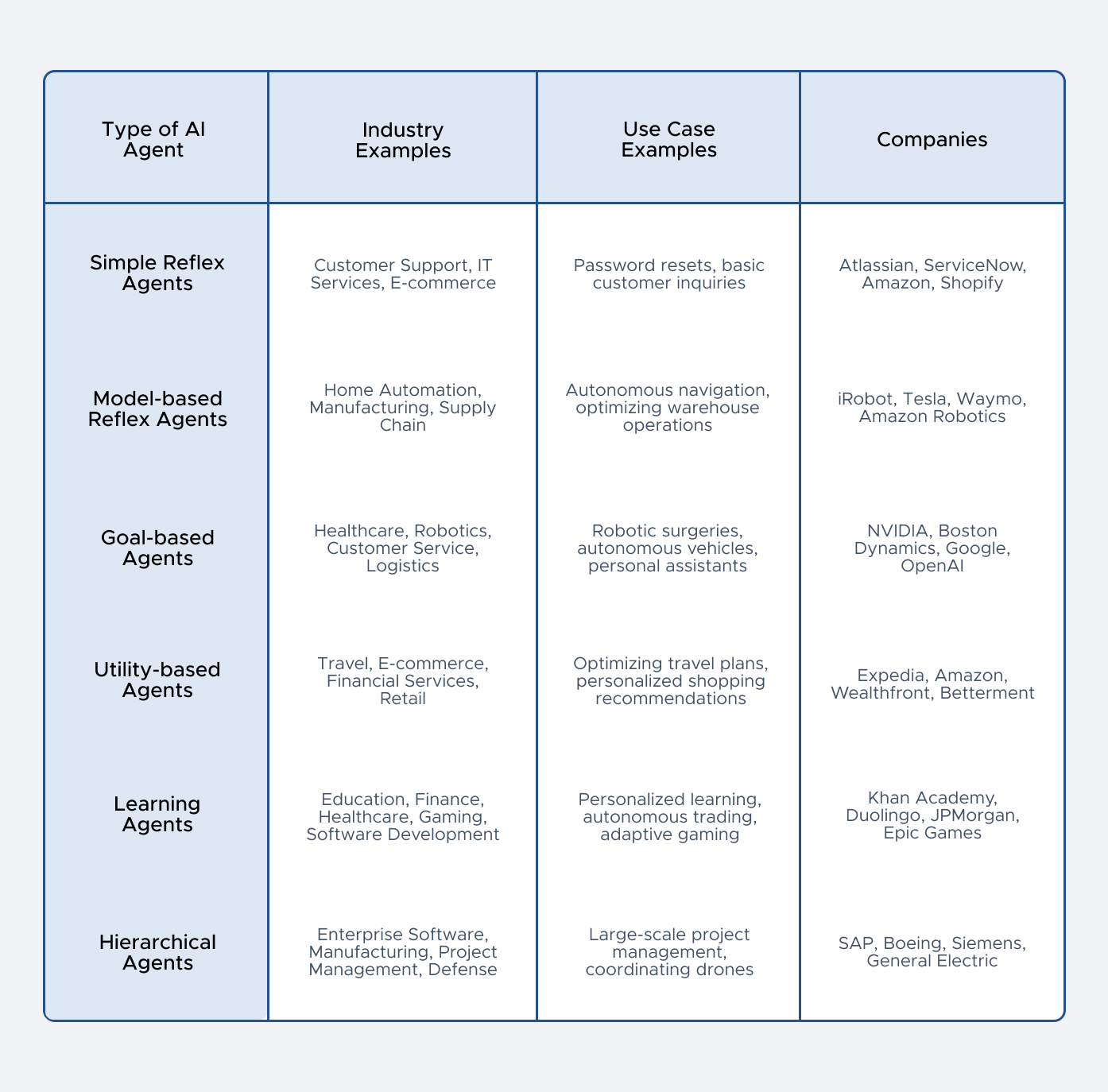
In recent years, tech giants like Google, Microsoft, and OpenAI have heavily invested in enhancing AI agent capabilities. Applications powered by LLMs, like Microsoft Copilot, Amazon Q, and Google's Project Astra, are evolving from passive knowledge-based tools to dynamic, action-driven solutions.
At the same time, companies and research labs are advancing agent-based models and multiagent AI systems. With the rapid pace of generative AI innovation, likely, AI agents will soon be as ubiquitous as chatbots are today.
Get a free consultation with the top AI agency 😎Contact us to get a consultation and prototype free of charge from one of our AI experts who worked with companies like Honda, Adidas, Samsung, and more 🚀
Framework for Implementing Generative AI Agents
How to create an AI agent? To build highly effective AI agents, follow this framework:
→ Pinpoint Core Capabilities
Begin by identifying the key skills needed for your task — whether it is data analysis, writing, coding, or information retrieval. This will shape how you design your AI agents.
→ Incorporate Reflection
Embed a reflection mechanism where the agent evaluates its work, spots errors, and iterates for improvement. Encourage a critical, "reviewer" mindset, pushing the agent to scrutinize and refine its outputs.
→ Leverage External Tools
Identify useful tools (e.g., web search, image models, databases) the agent can access. By enabling API calls, your AI agents can integrate these tools seamlessly into their workflows, expanding their capabilities.
→ Plan for Complex Tasks
For multi-step tasks, design a planning process where the agent not only crafts an action plan but also adapts if things go awry.
→ Foster Multi-Agent Collaboration
Create a multi-agent system where each agent takes on a specialized role, from researcher to decision-maker. Implement debate models among agents to refine outputs, combining strengths to enhance results.
→ Emphasize Rapid Iteration
Build an architecture that allows for fast cycles of output generation and refinement. Quick iterations ensure that the agents can continuously improve without relying on single attempts to get things right.
→ Test and Tweak
Continuously optimize agent prompts and workflows for reliability and quality. Recognize that some inconsistency is natural, so embrace iteration as part of the process.
The secret to success lies in blending these capabilities — reflection, tool integration, strategic planning, and multi-agent collaboration — into a unified architecture tailored to your specific needs. Start with simple tasks and gradually introduce more sophisticated features. Even with early models, this method can yield powerful results.
We were recently approached by a large warehouse services company with a team of a thousand employees. Their challenge? Not all sales managers were successfully closing deals.
The root cause was often a lack of experience and insufficient knowledge about potential clients who were getting stuck in the sales pipeline. To address this, the company implemented an AI agent that would prepare sales managers for client meetings by acting as a virtual Senior Sales Manager.
The AI agent's role was to thoroughly analyze the customer and prepare detailed reports for the company's employees. It identified who the potential client was, what type of business they ran, the current issues they faced, the industry challenges they dealt with, and who the key decision-makers were. It even helped craft conversation starters and anticipated the client's questions before they made a purchasing decision. For such complex scenarios, AI agents are the perfect solution.
However, this powerful tool isn't just for intricate tasks. AI agents can be deployed for simpler use cases too, gathering critical information quickly, executing tasks, making decisions autonomously, and self-checking to ensure accuracy.
Bespoke Generative AI Agents Tailored for Your Business
Crafting custom generative agents offers unique advantages for your business. While challenges like upfront investment, technical complexity, data quality issues, and security concerns may arise, they are manageable with proper planning, the right technical partners, and a solid data strategy.
Ready to elevate your customer service and streamline internal processes? Contact us for an interactive demo with our experts and discover how Gen AI agents can transform your business.