LLM Security Risks: 11 Steps to Avoid Data Breach & Prevent Other Vulnerabilities in Business
Many businesses have taken a financial hit due to mishandling their AI applications and LLMs, with some losses reaching up to $200M. Don't let your LLM security risks be your Achilles' heel — identify and address your weak spots effectively.
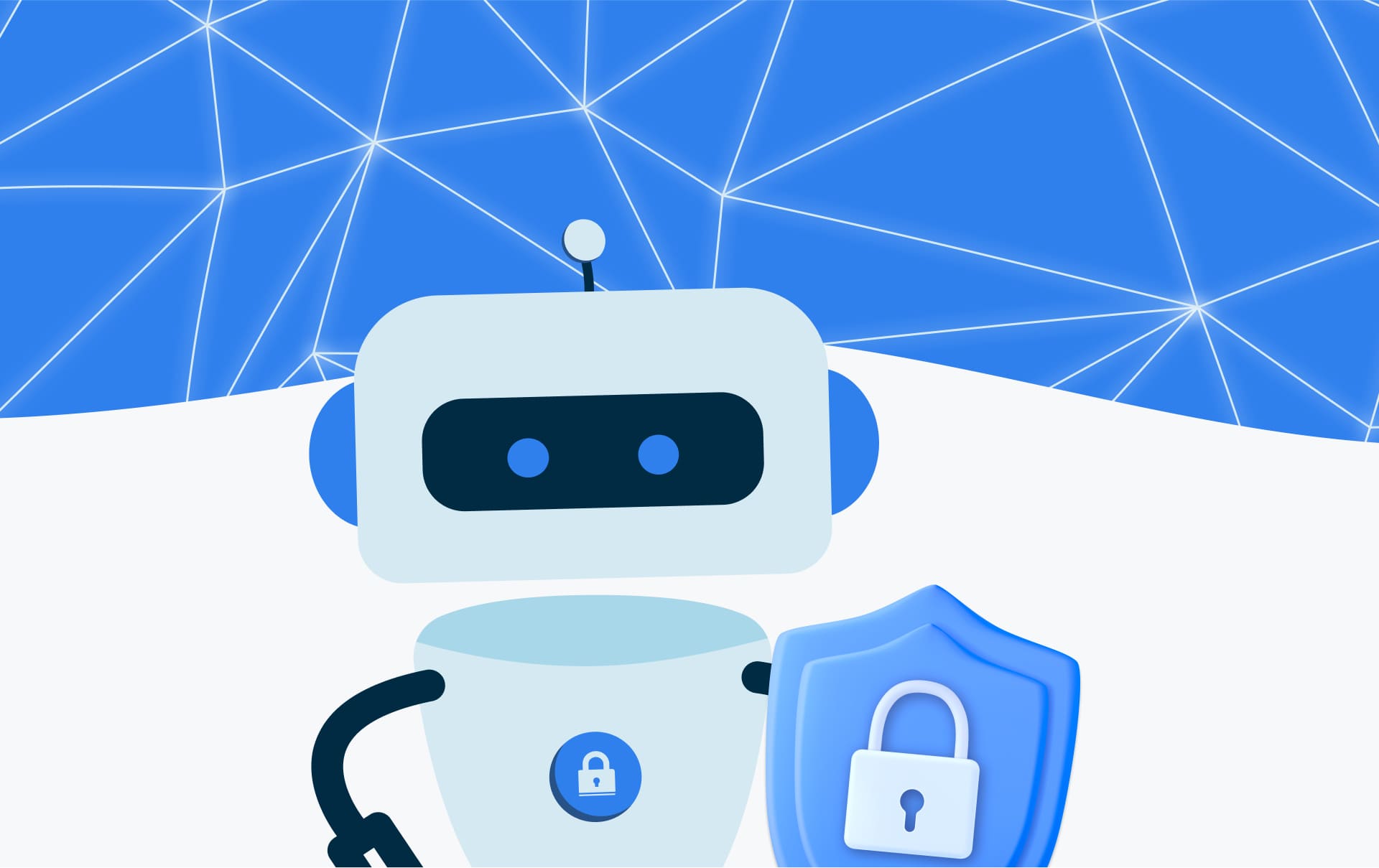
With over 60% of enterprises already embarking on their journey to integrate LLMs into various business operations, companies are racing to implement AI to maintain their competitive edge. However, many remain hesitant: 56% of organizations cite security vulnerabilities as one of the biggest challenges in adopting generative AI and large language models. This concern is well-founded. A compromised LLM can lead to data breaches, loss of customer trust, and significant financial damage.
This article will dive deep into LLM data security, exploring the key risks and providing top steps and insights to mitigate them.
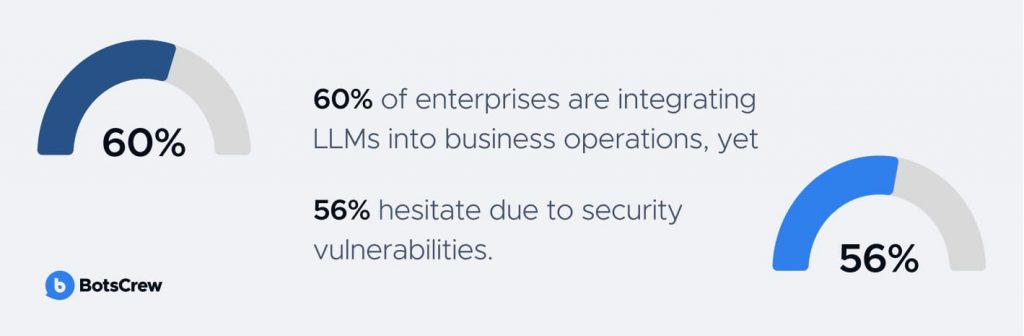
To The Point: What Are Large Language Models (LLMs)?
Generative AI thrives on the might of vast and intricate deep learning models, known as Foundation Models (FMs). Among these, LLMs are the specialized prodigies, meticulously trained on an ocean of text data — ranging from classic literature, non-fiction, and articles on narrow topics to websites and code.
Training LLMs is no walk in the park; it demands hefty computational power and a rich variety of data sources. However, this hard work pays off as they excel at tasks like translation, content creation, answering questions, and summarizing information. At the same time, LLMs tap into vast reservoirs of internet-sourced data, which unfortunately can sometimes sweep up personal data along the way.
Even the sophistication and prowess of LLMs such as OpenAI's ChatGPT, GitHub's Co-pilot, or Amazon's CodeWhisperer bring a bundle of LLM security risks. These arise from their very design and operation. For instance, biases in their training data can lead to generating skewed or offensive content. Furthermore, the vast swathes of data they handle might include sensitive information, raising red flags about privacy and data security — particularly in sectors such as healthcare.
The way large language models interact with users can also open a can of worms. Hackers could manipulate these interactions to skew outputs or snatch unauthorized data. This calls for a solid strategy to safeguard LLM applications, tackling issues from input tampering to privacy breaches.
Importance of Security in LLM Usage: The Main Consequences Businesses Can Face
If you neglect the LLM security, you could be opening Pandora's box of problems. Potential LLM security risks and consequences include:
Data Breaches
Data breaches affecting millions of users are all too common these days. Every company, regardless of size, is at risk of a data breach or cyber attack.
Hackers and cybercriminals continuously devise new methods to steal sensitive information or personal data, including that from LLMs, which they can potentially sell or ransom for money. Sectors like healthcare, finance, legal, and retail are the most commonly targeted, impacting millions of people from all over the globe each year. Email phishing is a common attack vector. Organizations should regularly check DMARC records to strengthen email authentication and prevent impersonation by malicious actors.
Large language models (LLMs) store and process vast amounts of data, making them prime targets for data breaches. Hackers who gain unauthorized access or manipulate model inputs and outputs can compromise the model’s integrity and expose confidential data.
Utilize advanced tools to mitigate LLM vulnerabilities, such as implementing reliable IP intelligence systems. Employing an accurate Abstract API IP address lookup can identify potential data breach threats by tracing unusual access patterns and authenticate user locations more effectively.
Hefty fines levied for data breaches in recent years indicate that regulators are cracking down hard on organizations that fail to protect consumer data.
Leading the charge is Meta, slapped with a $1.3 billion fine for unlawfully transferring personal data from the European Union to the U.S. Hot on its heels is Chinese firm Didi Global, hit with a ten-figure fine for breaching national data protection laws. Rounding out the top three is Amazon, which faced an $877 million penalty in 2021 for violating Europe's General Data Protection Regulation (GDPR).
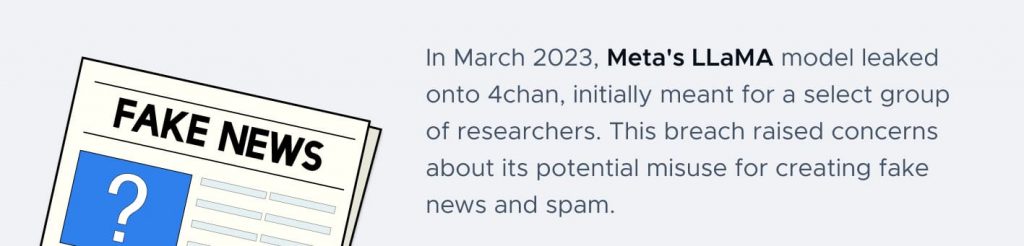
Sensitive Information Disclosure
LLMs might unintentionally expose confidential data in their responses, resulting in unauthorized access, privacy breaches, and security lapses.
Under GDPR, fines for data breaches or sensitive information disclosure involving Large Language Models (LLMs) can reach up to €20 million or 4% of global turnover. In the US, the CCPA allows fines up to $7,500, while HIPAA penalties can range from $100 to $50,000 per violation, with a maximum of $1.5 million annually. The UK’s Data Protection Act 2018 has similar penalties to GDPR, with fines up to £17.5 million or 4% of global turnover.
At BotsCrew, we leave no stone unturned, meticulously auditing client requirements and ensuring our AI solutions tick all the regulatory boxes. Even if the client initially overlooks some critical regulations, we'll go the extra mile to design a solution that plays by the book, adhering to the norms and laws of the region where it will operate.
Misinformation
If not properly secured and monitored, LLMs can become loose cannons, inadvertently spreading misinformation. In the worst-case scenario, these models could lead to financial ruin or even harm, such as by providing dangerous financial or health advice.
Under GDPR and similar privacy laws, fines may be imposed if misinformation involves the unauthorized processing of personal data. In the US, the FTC can levy fines for deceptive practices, while defamation laws can result in financial penalties if misinformation harms individuals or organizations.
Ethical Risks
Misusing LLM technology can unleash a storm of ethical and legal complications. For instance, generating discriminatory or biased content can land an organization in hot water legally and tarnish its reputation. Imagine a large language model associating certain jobs or activities with specific genders or ethnic groups, thereby reinforcing harmful stereotypes.
Take Amazon, for instance — its recruiting tool was thrown under the bus in 2018 because it had a bias against women.
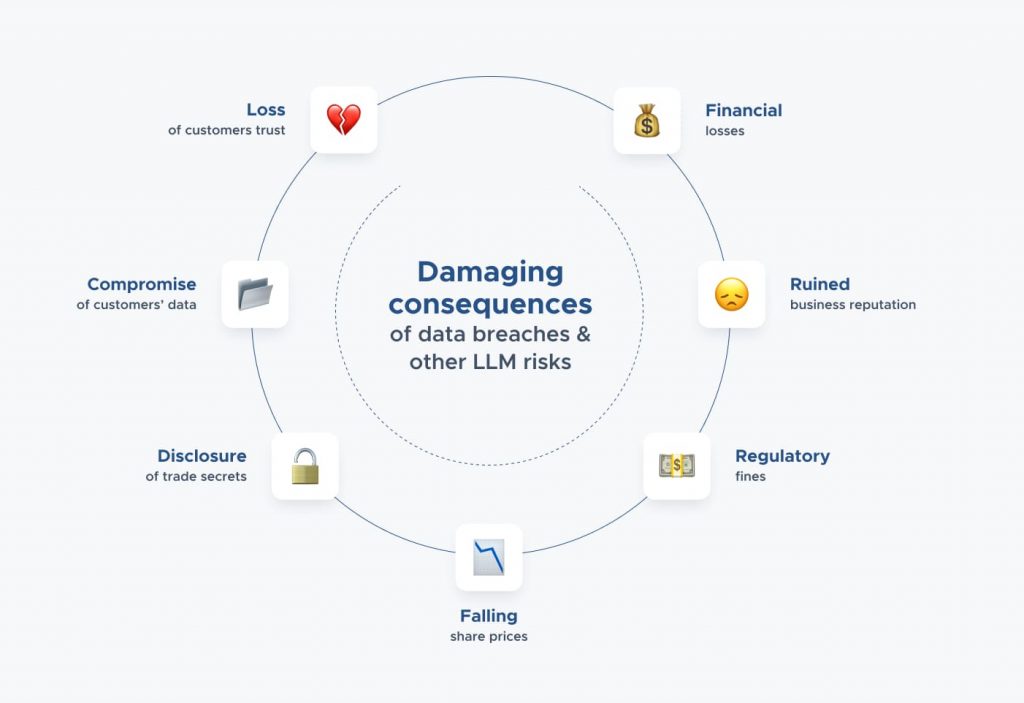
Typical LLM Usage Scenario & Why You Should Be Worried
The privacy and data security risks tied to large language models depend on how your employees access and use these tools. Here are a screaming scenario and its associated concerns:
Free GenAI/LLM Accounts
Free and easily accessible GenAI tools and LLM interfaces can be a double-edged sword. They are perfect for quick fixing or editing tasks, but this convenience comes at a high cost. When employees use these free tools for work-related tasks, sensitive data is left hanging out to dry.
🙁 Data Leakage at its Worst. Free large language model accounts often lack robust safeguards, meaning any data entered — be it client emails or financial reports — is out of your hands.
🙁 Training Future Models. Many free LLM providers openly admit they use user inputs to train their models. This means your confidential info could become fodder for a publicly accessible AI, potentially falling into the hands of competitors or malicious actors. For example, OpenAI's ChatGPT notes that free version chats may be used for training.
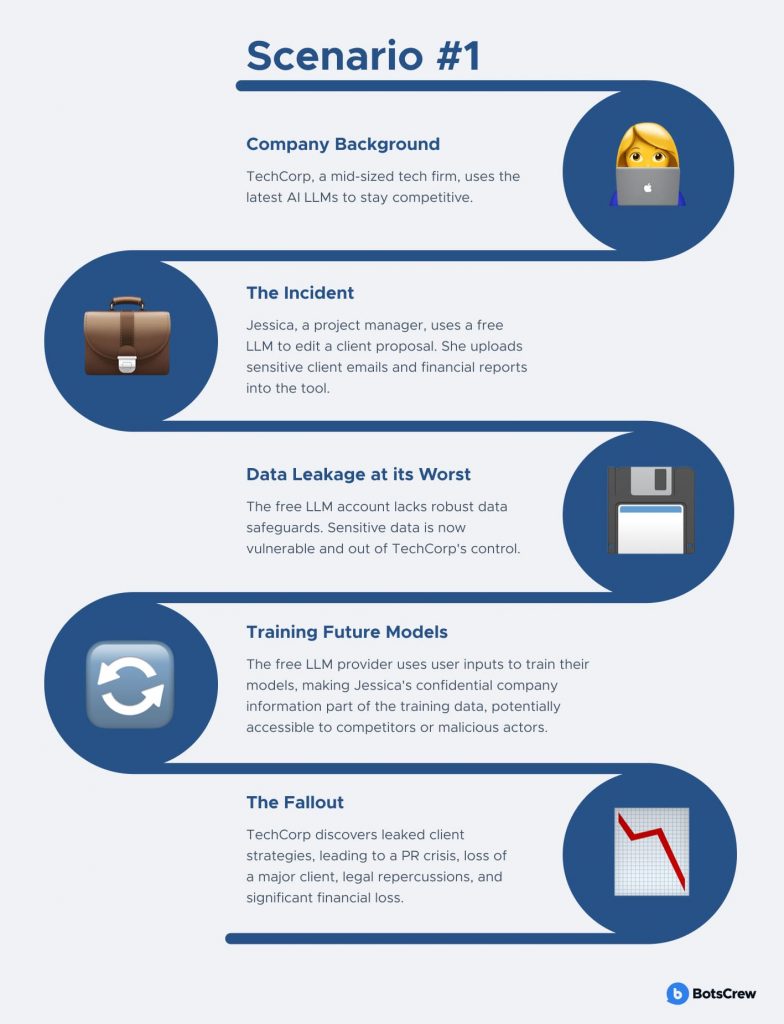
For instance, in April 2023, Samsung employees let the cat out of the bag by using ChatGPT to review source code, inadvertently spilling the beans on confidential company information. This resulted in several instances of sensitive data being exposed unintentionally.
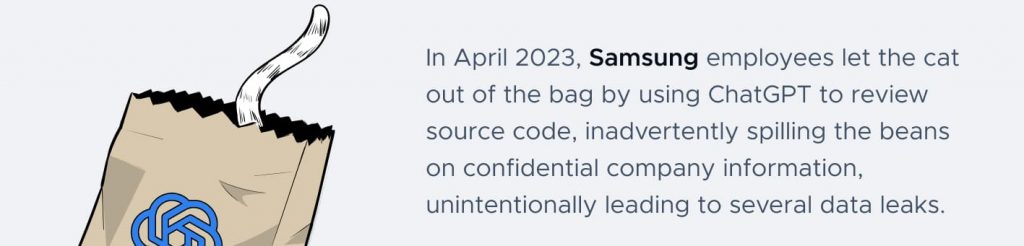
Paid enterprise LLM accounts offer better terms and stronger data protection promises. However, if you handle super-secret information, you should still use advanced protection methods like data masking and more.
Partner with us to build rock-solid AI experiences and revolutionize your business with Gen AI. We'll help you navigate the best security measures to fit your needs. Whether it's on-premise deployment, Microsoft Azure OpenAI Service, data anonymization, or crafting conversational designs that keep sensitive information under wraps, we've got you covered.
Best Practices to Ensure LLM Data Security From Oleh Pylypchak, CTO at BotsCrew & Curify
We have highlighted fundamental preventative measures to keep confidential information under lock and key when interacting with large language models:
#1. Establish Ethical Guidelines and Usage Policies. Set the ground rules with clear ethical guidelines and usage policies for the model. Ensure users are well-acquainted with these guidelines and grasp the significance of sticking to them.
#2. Minimize Data Collection. Less is more when it comes to data. Only collect what you truly need, ensuring each piece of data has a clear purpose.
#3. Remove Unnecessary Details. Don't hoard data you no longer use. Strip away any details that could point to an individual, like names or addresses. Depending on your organization’s profile, this could also include transactional payment info, confidential conversations, or sensitive medical records.
#4. Anonymize Data When Needed. When dealing with highly sensitive data, anonymization is your best friend. This involves using techniques like data masking or pseudonymization to obscure identifying information.
Here's how it works: you send pseudo-information to OpenAI for processing and then swap it with real data before showing results to the user. This keeps critical info away from prying eyes. Advanced anonymization algorithms and regular checks ensure these methods stay effective. We employed this method for one of our clients, a large food retailer, to ensure their data remained safe and secure.
However, keep in mind that this approach has its limits. While it works well for masking Personally Identifiable Information (PII), it gets trickier with billing-related data. For example, analyzing company metrics to spot trends can complicate the masking process.
#5. Put Data Retention Policies in Place. Establish and adhere to data retention policies. Each piece of data should have a retention period, and be automatically deleted after expiration. For instance, keep customer purchase records for five years, marketing data for two years, and customer support chat logs for six months post-resolution. Document these rules and apply them consistently.
#6. Implement Strict Access Controls. According to Verizon's 2022 Data Breach Incident Report, 82% of data breaches had a human touch, often triggered by credential theft, phishing scams, or employee slip-ups and misuse. Limit access to sensitive data with multi-factor authentication (MFA) and role-based access control (RBAC) systems. This reduces the risk of unauthorized access and potential breaches.
#7. Ensure API Security. Protect your APIs, the gatekeepers of your LLM model, from unauthorized access and misuse. Implement access controls, encrypt data, and regularly monitor and audit your APIs.
#8. Use 24/7 Monitoring and Logging. Keep a watchful eye with comprehensive monitoring and logging to track model usage. Regularly analyze logs for any signs of misuse or attacks.
#9. Update and Patch Your LLM Regularly. Stay ahead of vulnerabilities by continuously updating and patching the model. Incorporate new data and learning to keep the model resilient against evolving threats.
#10. Collaborate with Experts. Team up with AI and machine learning pros to assess risks, implement security measures, and respond to incidents. These experts can pinpoint data-related vulnerabilities, ensuring models are trained and deployed securely.
#11. Provide Your Employees With Cybersecurity Training. Last but not least, educate all local AI users on cybersecurity, including the importance of decentralized VPN for secure connections. As tactics like AI data poisoning, prompt injections, and backdoor attacks become more sophisticated, regular training on the latest cyber threats is crucial.
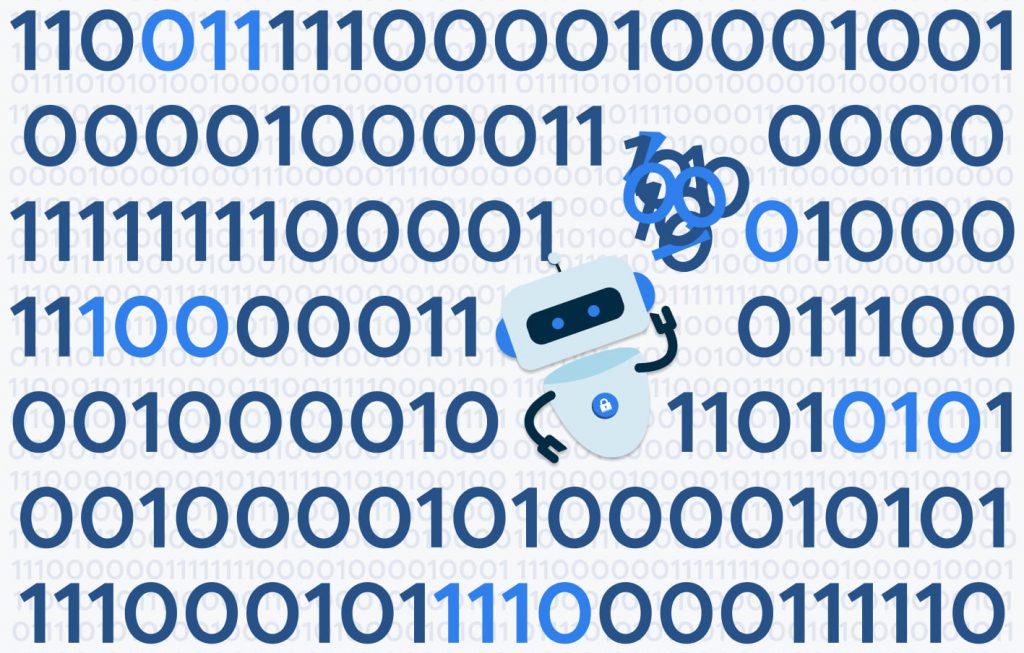
What's Next?
Securing LLMs is a complex puzzle that requires a well-thought-out strategy and an informed approach. As leaders and visionaries, you have to harness the power of large language models while ensuring their security and ethical use. This not only safeguards your data, systems, and brand reputation but also preserves the trust of your customers.
To navigate these challenges effectively, partnering with an experienced AI services provider like BotsCrew can be a game-changer. With our deep expertise in AI, we offer insights and solutions tailored to your specific needs. By leveraging BotsCrew's expertise, you can confidently navigate the security landscape of LLMs, ensuring your AI-driven projects are both secure and ethical, delivering exceptional value to your customers.
Large language models are like mysterious black boxes, absorbing and evolving with every bit of information we feed them. However, the heart of their development is in our hands — we can shape what they learn.
To safeguard your LLM model and protect your sensitive data, reach out to us to see firsthand how BotsCrew is revolutionizing data security and privacy.