How AI Is Revolutionizing Electronic Health Records (AI EHR)
Not long ago, doctors and nurses were drowning in paperwork, buried under mountains of patient files, and spending more time on forms than with actual patients. Traditional EHRs threw them a lifeline — but let's be honest, it was still a slog. Enter an AI EHR — the game-changer. How exactly is AI transforming the daily grind of medical professionals? Stick around to discover the revolution that is making hospitals smarter, faster, and more efficient than ever.
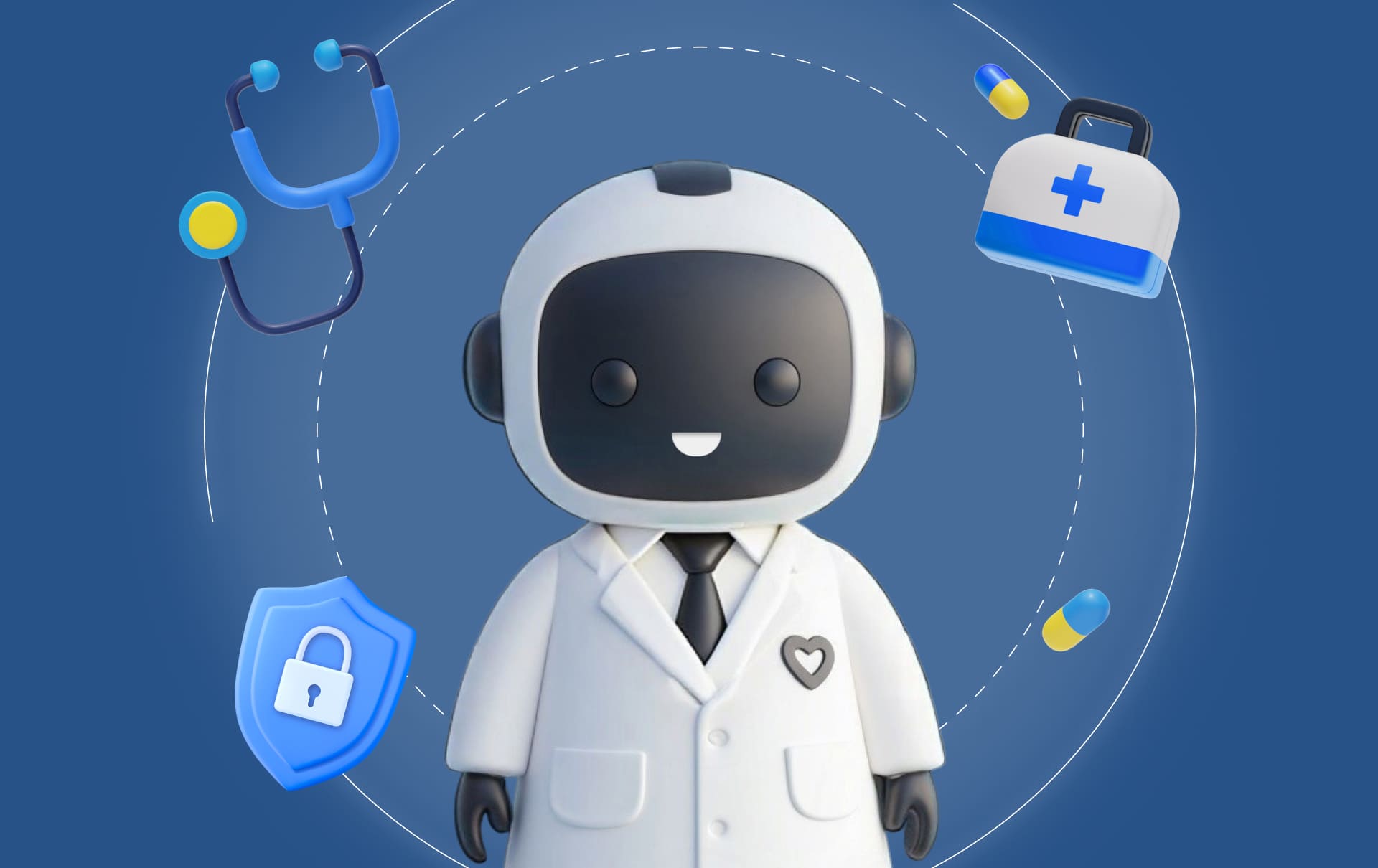
Before the widespread adoption of EHRs, healthcare professionals relied on paper-based record keeping — an outdated system riddled with inefficiencies:
☹️ Time-Consuming Documentation. Physicians and nurses spent excessive time filling out patient forms, leading to reduced face-to-face time with patients.
☹️ Risk of Errors. Handwritten notes were prone to misinterpretation, leading to medication errors and misdiagnoses.
☹️ Limited Data Sharing. Patient records were confined to specific hospitals or clinics, making it challenging to share data across institutions, especially in emergency situations.
☹️ Storage and Security Issues. Paper records required physical storage, were susceptible to loss or damage, and posed confidentiality risks.
The shift to EHRs was meant to solve these problems. Instead, it digitized them. Clunky interfaces, endless clicking, and rigid workflows have only added to physicians' frustration. This is where AI steps in. By transforming EHRs from passive data repositories into intelligent, proactive systems, AI has the potential to lift the administrative burden off healthcare professionals and enhance patient care.
It's no surprise that, according to Deloitte, industry titans raking in over $10 billion a year are throwing down more than $50 million on AI projects, betting big on a tech-driven future. Mid-sized players aren't far behind, strategically allocating funds to stay competitive.
In this article, we'll explore the benefits of AI in the EHR system and how exactly AI EHRs are revolutionizing healthcare, turning record-keeping from a burden into a seamless, intelligent process.
What Are Electronic Health Records (EHRs): Understanding the Basics
An EHR (Electronic Health Record) is a smart, secure hub that stores a patient's medical history, treatments, and test results, making vital information accessible anytime, anywhere.
It is the digital backbone of modern healthcare, these systems have revolutionized the industry, eliminating paper trails and making patient information more accurate, accessible, and secure. Unlike Electronic Medical Records (EMRs), which are limited to single-provider use, EHRs are built for collaboration, giving a 360-degree view of a patient's health history.
EHRs aren't a one-size-fits-all deal — they come in different formats to suit various healthcare settings. Ambulatory EHRs keep things running smoothly in clinics, Inpatient EHRs handle the heavy lifting for hospitals, and Specialty EHRs are fine-tuned for niche medical practices.
Behind the scenes, a powerhouse team of software engineers, healthcare pros, and data wizards come together to build these systems, ensuring they are not just functional but also smart, secure, and seamless.
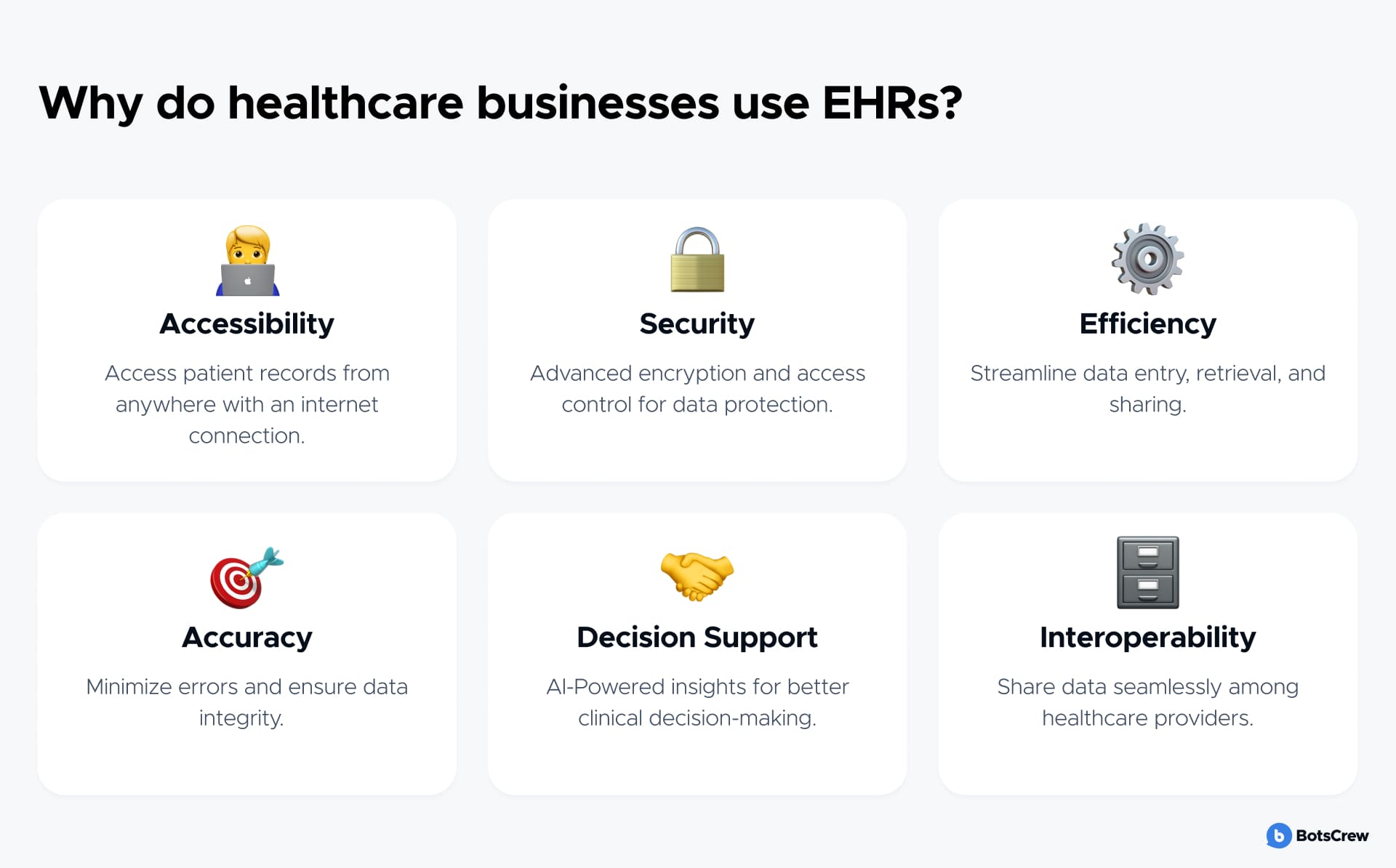
The Current State of EHR Systems: The Good, The Bad, and The Frustrating
Let's break down the numbers and some current stats:
Over 96% of U.S. hospitals and 89% of private practice physicians use EHRs, making them central to healthcare operations. Physicians dedicate an average of 4.5 hours each day navigating EHR systems, with their digital paperwork often spilling beyond clinic hours.
However, only 46% of hospitals report that their EHRs are interoperable with other systems. Lack of integration complicates data sharing, leading to increased treatment times and duplicated tests, collectively costing the healthcare system billions annually.
Nearly 60% of clinicians report that EHR systems negatively impact their productivity, with over half expressing frustration due to tedious interfaces and extensive data entry requirements. On top of their packed schedules, they spend an extra 1.77 hours daily on documentation after patient visits. This dissatisfaction is linked to a 23% increase in clinician burnout, impacting both staff retention and quality of care.
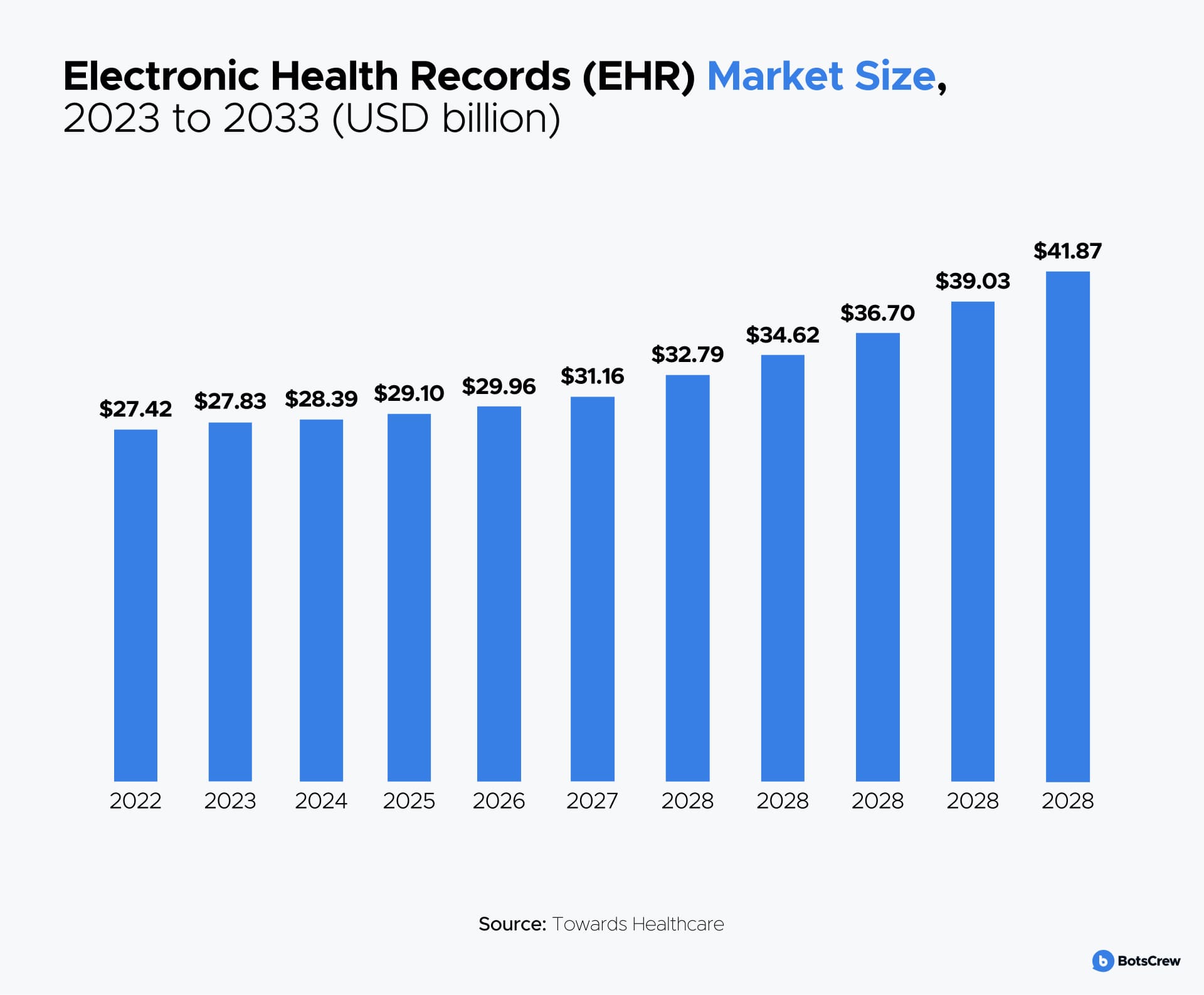
What's caused such statistics? From data entry mishaps to rising operational costs, here is where traditional EHRs are falling short:
🚨 Error-prone data entry. When healthcare professionals are stuck manually entering data, mistakes are inevitable. A single typo in a patient's record can mean the difference between the right treatment and a dangerous medical error.
🐢 Delayed care. Updating records, validating data, and navigating sluggish interfaces slow decision-making. Patients end up waiting longer for treatment, and hospitals struggle to deliver fast, value-driven care.
🔍 Fragmented records. When patient information is scattered across disconnected systems, miscommunication becomes a major problem. Disjointed records can lead to conflicting treatments, forcing doctors to play detective instead of focusing on care.
🤕 Patient dissatisfaction. Errors, delays, and disjointed records don't just frustrate doctors — they frustrate patients, too. When healthcare feels inefficient and impersonal, trust in the system erodes.
AI technologies are stepping in to fix these pain points, transforming inefficiencies into seamless, intelligent workflows.
Leverage AI capabilities to redefine your electronic healthcare record system!
Technologies & Use Cases of AI in EHR + Real-World Implementations
According to Markets and Markets research, AI in healthcare is set to hit $45.2 billion by 2026. And a KPMG survey found that 67% of healthcare executives say their teams are already on board with AI.
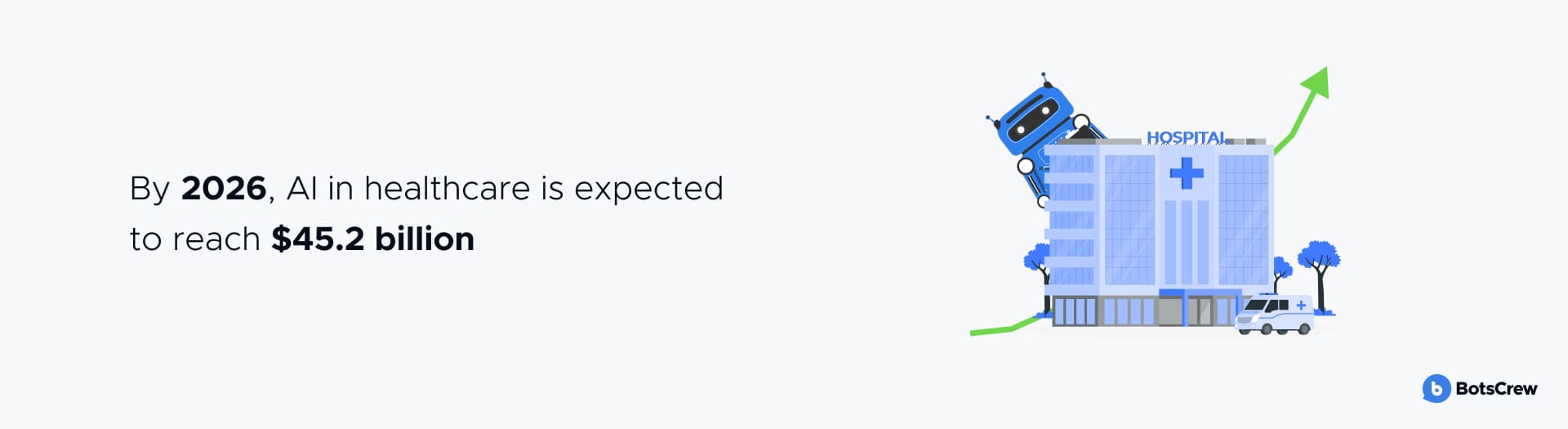
So, how is AI cutting through the red tape and transforming EHRs? Below are key use cases and technologies where using AI to improve electronic health records is making an impact:
Natural Language Processing (NLP)
NLP helps process unstructured data — like clinical notes and discharge summaries, which make up 80% of healthcare data. AI converts clinical notes into ICD-10 and CPT codes, cutting documentation time by up to 40%, giving doctors back approximately 3.5 hours per shift to focus on patients.
Extracting insights: for example, tools like Amazon Comprehend Medical and IBM Watson Health improve coding accuracy, pulling out crucial details on diagnoses, allergies, and medications.
Machine Learning (ML)
Machine learning is revolutionizing EHRs with predictive analytics, helping doctors stay ahead of complications. ML models identify patients at risk for sepsis or heart failure. Moreover, hospitals using ML-based prediction tools successfully reduced patient hospital stays by 6.5% and readmission rates by 2.1%, leading to significant cost savings.
A 2023 study developed an XGBoost model to predict 30-day mortality in sepsis patients, aiming to assist clinicians in tailoring precise management and therapy. The University of Chicago Medicine cut ICU transfers by 20% using ML-driven early warnings.
Northwell Health, New York's largest healthcare system, developed an AI-powered tool called iNav to enhance early detection of pancreatic cancer. By analyzing MRI and CT scans taken for unrelated health issues, iNav identifies cancerous masses or lesions early, reducing the time from diagnosis to treatment by 50%. This proactive approach aims to improve the five-year survival rate for pancreatic cancer, which currently stands at just 13% due to late diagnoses.
Robotic Process Automation (RPA)
RPA takes over time-consuming admin work, letting healthcare professionals focus on patient care instead of paperwork.
Hospitals using RPA cut admin costs by 50% saving over $150 billion in annual cost savings industry-wide.
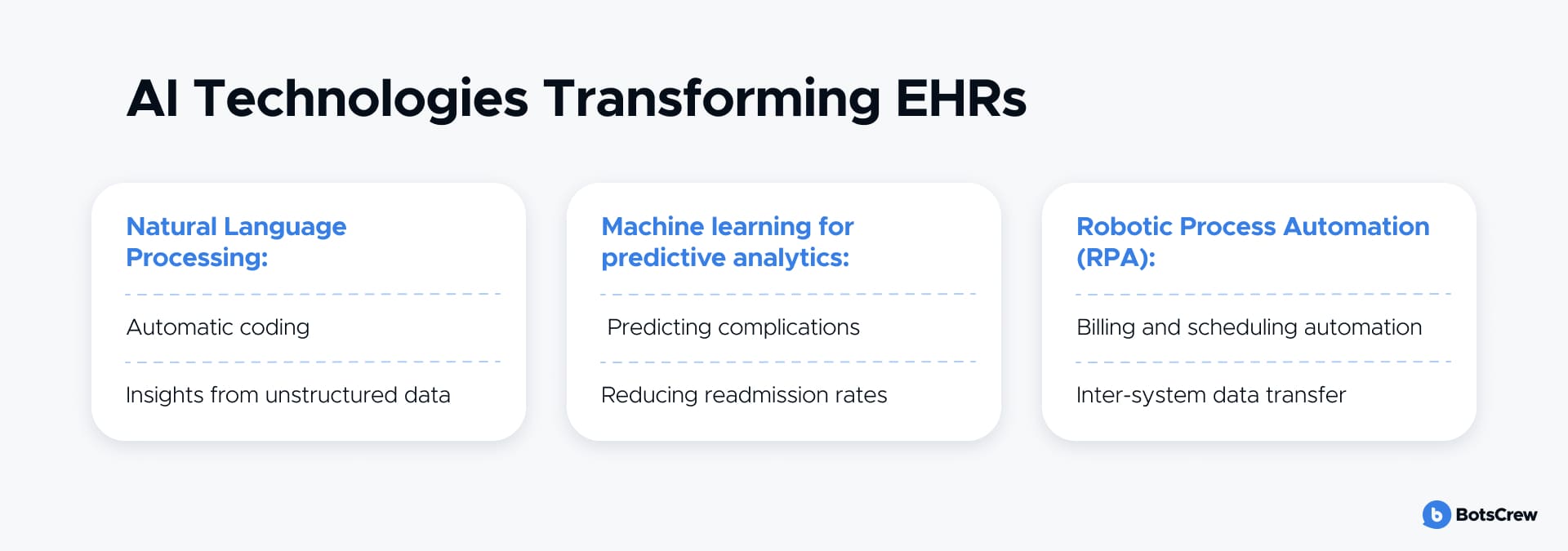
Personalized Treatment Plans
AI tailors treatment plans based on a patient's genetic makeup, medical history, and lifestyle, leading to more effective therapies.
IBM Watson Health has been used to suggest personalized cancer treatments by analyzing genetic data and medical literature, improving patient-specific care.
However, AI-driven treatment recommendations remain heavily regulated. Without proper certification, AI insights cannot replace medical advice. Instead, AI can assist by:
Medication Management and Adherence
AI helps in prescribing and monitoring medication adherence, reducing the risk of adverse drug reactions.
AI-powered virtual assistants send medication reminders to patients, significantly improving adherence rates for chronic conditions like diabetes and hypertension.
Virtual Medical Assistants for Administrative Efficiency
AI-powered virtual assistants handle scheduling, record updates, and answering patient inquiries, allowing healthcare providers to focus on direct patient care.
Lark Health, a digital health company based in Mountain View, California, offers AI-driven virtual health assistants that provide 24/7 support for chronic conditions such as type 2 diabetes and hypertension. These AI-powered assistants engage patients through a text-messaging interface, delivering personalized health coaching, medication reminders, and real-time feedback.
Image and Signal Analysis
ML and deep learning (DL) assist in the detection, classification, and quantification of abnormalities in medical images such as X-rays, MRIs, and CT scans. By learning from vast datasets, AI models can identify patterns and anomalies that may be challenging for human observers, leading to earlier and more accurate diagnoses.
Google's DeepMind has developed an AI system capable of analyzing retinal scans to detect over 50 eye diseases, including glaucoma and macular degeneration, with accuracy comparable to that of expert clinicians. In collaboration with Moorfields Eye Hospital, DeepMind trained the AI on a large dataset of retinal images, enabling it to recommend appropriate patient referrals based on the detected conditions.
Clinical Decision Support Systems (CDSS)
AI-driven EHRs provide real-time alerts and evidence-based recommendations for better clinical decisions.
Modern companies offer Clinical Decision Support Systems (CDSS) that assist physicians by providing real-time alerts for potential drug interactions and allergy risks, thereby reducing prescription errors. These systems analyze patient data to identify possible adverse reactions, ensuring safer medication management.
For example, a Medication Clinical Decision Support (mCDS) system can display alerts detailing the severity of potential drug-drug interactions and related order information. It can also provide alerts for drug-allergy interactions, enabling healthcare providers to make informed decisions and enhance patient safety.
Enhanced Data Security and Compliance
AI strengthens EHR security by detecting anomalies and ensuring regulatory compliance.
In fact, AI-powered anomaly detection flags suspicious activity up to 60% faster, helping hospitals stay compliant with HIPAA regulations and prevent costly data breaches.
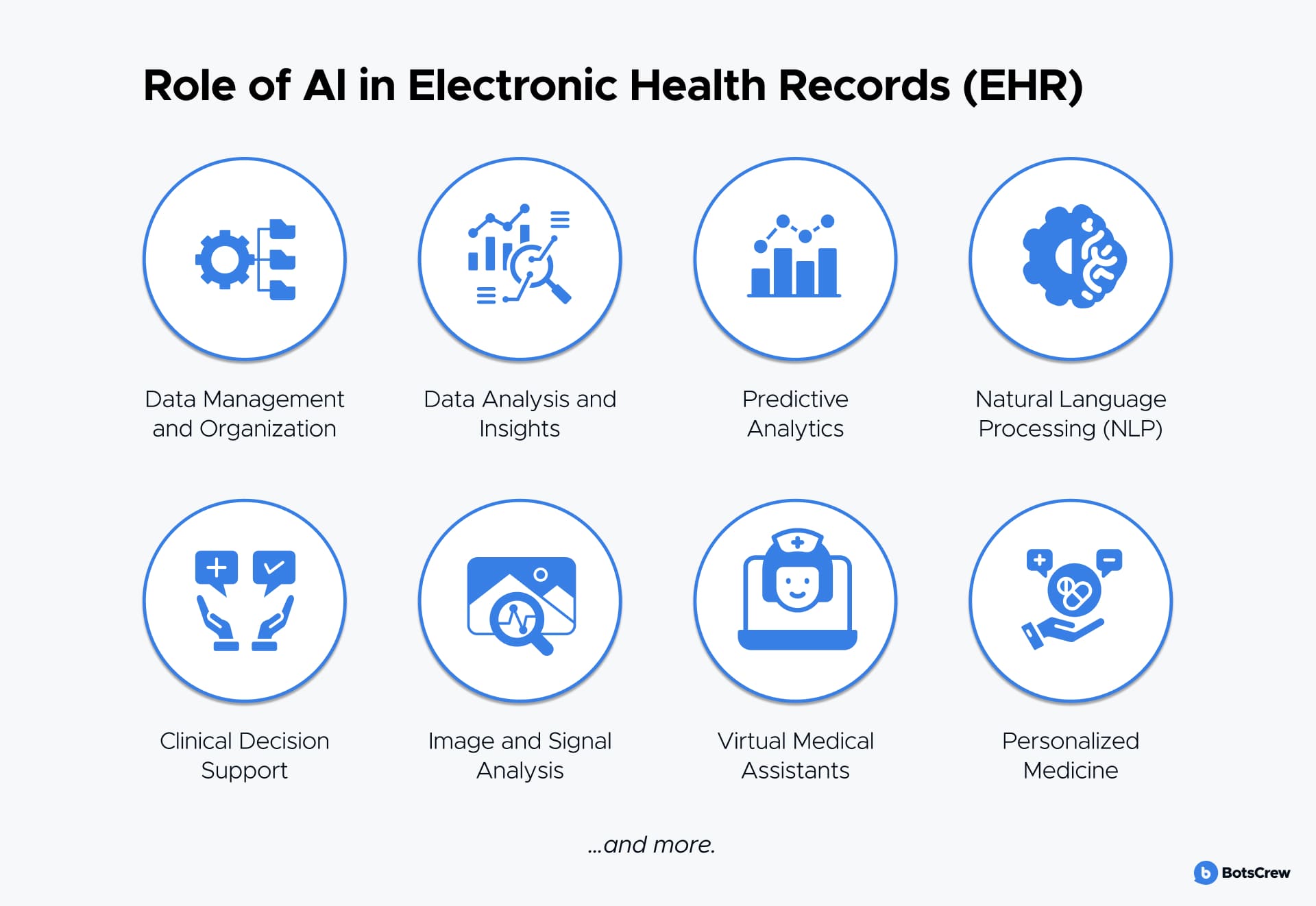
Breaking Down the Cost of AI-Powered EHR Development
So, you're thinking about rolling out an AI-driven Electronic Health Records (EHR) system? The price tag can range from $30,000 to $70,000+, depending on how tricked-out you want it. The final cost depends on factors like system complexity, feature depth, and project scale — basically, how ambitious your vision is. Here's the lowdown on where your budget will go:
The Big-Ticket Items in EHR Development
- Scope & Complexity (15–20%). Before a single line of code is written, you need to map out your project's scope. Bigger, more intricate systems demand more planning, which means higher costs.
- Features & Functionality (20–25%). Want cutting-edge AI-driven insights, predictive analytics, and seamless automation? That R&D doesn't come cheap. The more bells and whistles, the steeper the price tag.
- Customization (10–15%). One-size-fits-all? Not in healthcare. Tailoring an EHR system to fit your specific workflows and operational needs adds to the development effort (and the bill).
- Regulatory Compliance (10–15%). Navigating HIPAA, GDPR, and other data security laws is non-negotiable. Security and compliance testing will cost you, but skimping here could mean hefty fines down the road.
- Data Integration (10–15%). If your EHR needs to sync seamlessly with legacy systems, lab databases, or third-party apps, expect a chunk of your budget to go toward smooth and secure data flow.
- UI/UX (5–10%). No one wants to wrestle with clunky software and a sleek, intuitive design is key for adoption.
- Testing & Quality Assurance (10–15%). In healthcare, system glitches aren't just frustrating — they can be life-threatening. Rigorous testing ensures your EHR is airtight before going live.
- Deployment & Maintenance (10–15%). Once your EHR is up and running, you'll need ongoing updates, bug fixes, and optimizations to keep it performing at peak efficiency.
Hidden Costs to Keep on Your Radar
Beyond core development, there are other must-haves that can inflate your budget:
✅ AI Integration & Data Migration: training AI models and migrating existing patient records into a new system takes time (and money).
✅ Infrastructure Costs: cloud hosting, server maintenance, and data storage fees will stack up over time.
✅ Cybersecurity & Compliance: investing in rock-solid security protocols is crucial to fend off breaches and avoid regulatory penalties.
At the end of the day, investing in AI in EHR isn't just about the upfront cost. It is about long-term efficiency, accuracy, and compliance. And when done right, the ROI can make it all worthwhile.
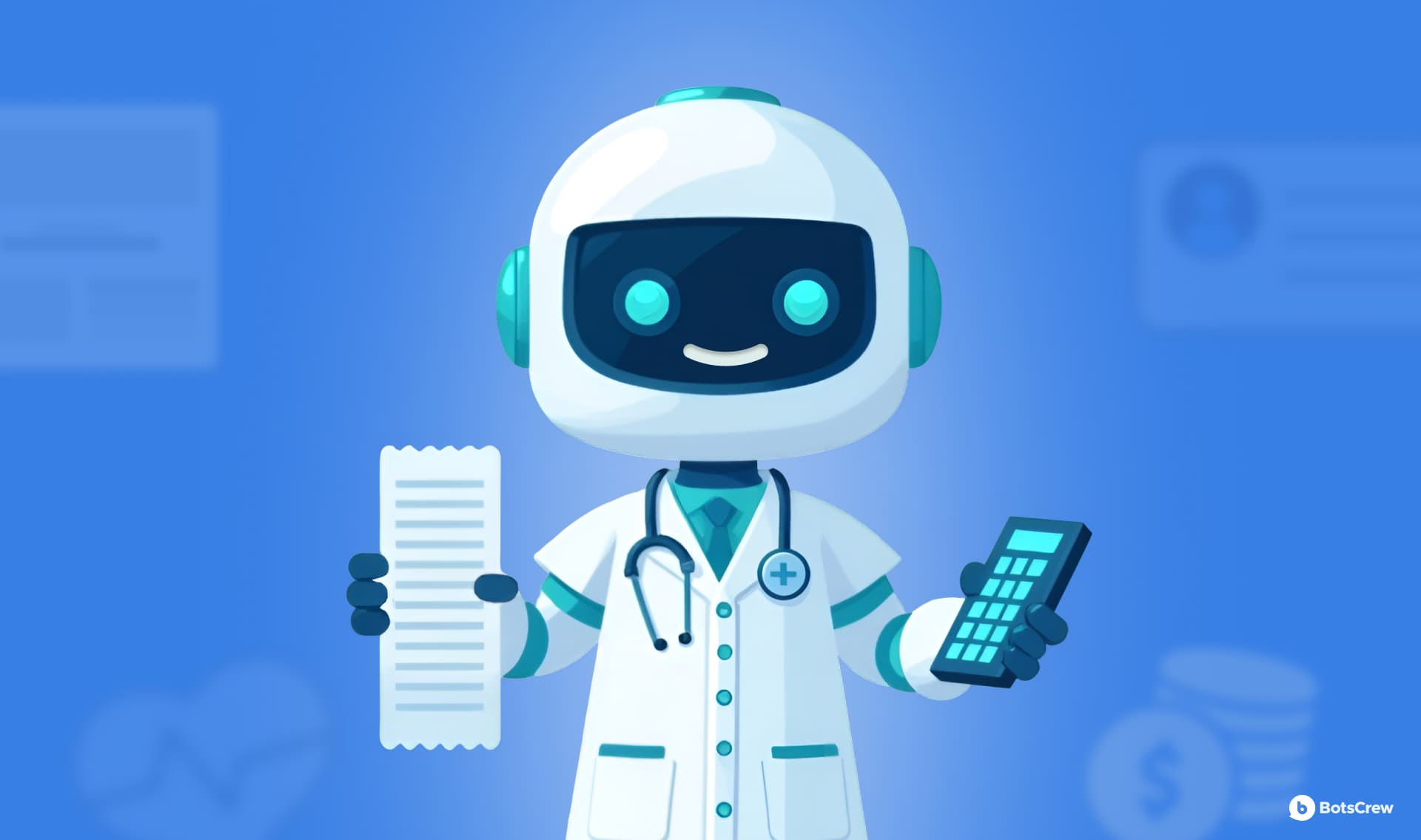
Challenges and Solutions in AI EHR Implementation
Using AI to improve electronic health records presents a groundbreaking opportunity to transform healthcare. However, this innovation comes with significant challenges that must be addressed to ensure seamless implementation and optimal benefits.
#1. Data privacy and security. Medical professionals and IT teams worry about data privacy, compliance, and potential risks when integrating AI-driven solutions. Questions arise: Who has access to patient data? How is it protected? Can AI be trusted with sensitive health information?
The fear is understandable — healthcare data is among the most regulated and valuable, making it a prime target for cyber threats. Striking a balance between AI-powered efficiency and stringent security standards is crucial.
💡 When it comes to security in healthcare, everything hinges on the type of data being processed. The biggest concerns revolve around access to medical records and sharing them with third parties. Regulations like HIPAA (U.S.) and GDPR (E.U.) impose strict controls on how this data can be used and shared.
For general analytics or non-sensitive tasks, there’s more flexibility and fewer restrictions. However, when dealing with commercial AI models from third-party providers, the risks decrease, allowing for greater creativity in implementation.
Handling PHI (Protected Health Information)
Working with PHI adds complexity. One option is using commercial AI models under a HIPAA Business Associate Agreement (BAA) — a contract between two HIPAA-compliant companies that allows secure data exchange. OpenAI, for example, offers this option.
But even with a BAA in place, transmitting personal health data remains a sensitive issue. An alternative is self-hosted AI models, which keep all data within the healthcare provider's system, ensuring compliance but requiring significant infrastructure investment.
Deploying AI in Cloud-Based Healthcare Systems
For healthcare providers leveraging cloud platforms like AWS, GCP, or Azure, security compliance becomes easier. These providers offer specialized tools to handle sensitive data securely:
- AWS provides tailored solutions for protected health data.
- Azure offers a similar suite of compliance-ready services.
- GCP also includes built-in regulatory compliance tools.
The key challenge is determining what kind of data will be processed and understanding the security constraints before integrating AI solutions.
While AI integration in healthcare follows standard IT principles, the added complexity of handling sensitive medical data requires extra layers of security and compliance.
#2. Training and adoption challenges. Healthcare staff may struggle to adapt to AI and EHR systems, leading to resistance and inefficiencies in implementation.
Providing hands-on training and demonstrating the benefits of AI tools — such as reduced documentation time and improved decision-making — can encourage adoption. Continuous support and feedback mechanisms should be in place to address concerns and refine system usability.
3. Data quality and standardization. AI models rely on high-quality, structured data for accurate insights. Inconsistent data entry, incomplete records, and lack of standardization can hinder AI's effectiveness.
Investing in data cleansing and normalization processes ensures data accuracy. Establishing universal coding systems and structured data formats enhances interoperability and improves AI performance.
#5. Ethical considerations and algorithm transparency. AI decision-making must be transparent, unbiased, and aligned with ethical healthcare standards. Lack of accountability for AI-driven recommendations can raise legal and ethical concerns.
AI algorithms should undergo rigorous validation by clinicians before deployment. Ensuring interpretability in AI models will foster trust among healthcare professionals. Ethical guidelines must also be established, emphasizing human oversight in critical medical decisions.
#6. Regulatory compliance. AI apps in healthcare must comply with evolving regulations and legal requirements, which can be complex and time-consuming to navigate.
Organizations should engage compliance experts to ensure adherence to legal standards. Keeping up with regulatory changes and proactively updating AI systems to meet new requirements will prevent legal complications.
#7. Collaboration and knowledge sharing. Many healthcare institutions operate in silos, slowing down AI adoption and innovation.
Encouraging collaboration among hospitals, AI researchers, and tech firms can drive advancements and shared best practices. Industry conferences, knowledge-sharing platforms, and open research initiatives can accelerate AI adoption in healthcare.
📝 Integrating AI in the EHR system isn't just about adopting the latest technology. It is about choosing the right solutions that align with your organization's needs. Healthcare leaders must take a strategic approach by:
✅ Assessing current EHR inefficiencies to identify where AI can provide the most value.
✅ Prioritizing data security and compliance to ensure AI implementation meets regulatory standards.
✅ Investing in staff training to maximize adoption and effectiveness.
Curious about how AI can optimize your EHR? Contact us to learn more about AI solutions for better patient care and operational efficiency.